In previous chapters, we covered the basics of deep learning as applied to the fields of computer vision and natural language processing (NLP). Most of these techniques can be broadly classified as supervised learning techniques, where the goal is to learn patterns from training data and apply them to unseen test instances. This pattern learning is often represented as a model learnt over large volumes of training data. Obtaining such large volumes of labeled data is often a challenge. This necessitates a new approach to learning patterns from data with or without labels. To ensure correct training, minimal supervision may be provided in the form of a reward if the model correctly learns a pattern, or a penalty otherwise. Reinforcement learning provides a statistical framework to achieve this task in a principled manner. In this chapter, we will cover...
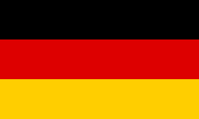
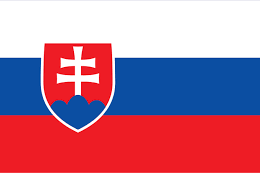
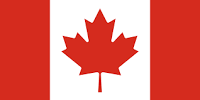
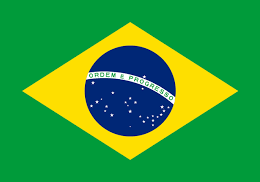

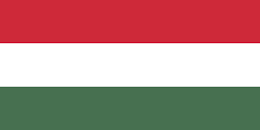
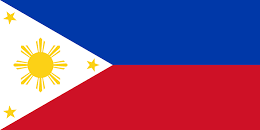
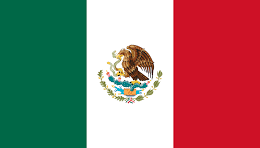
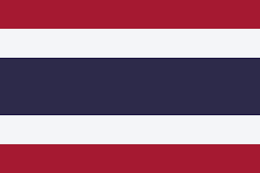
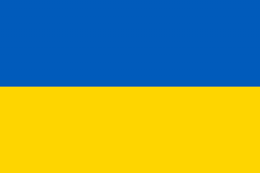
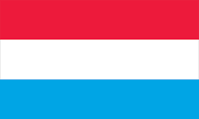
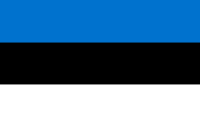
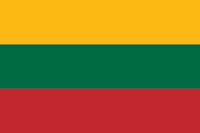
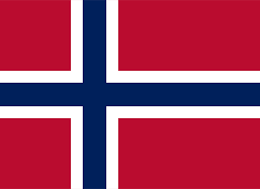
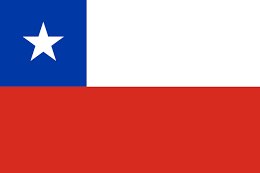
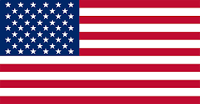
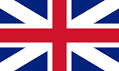
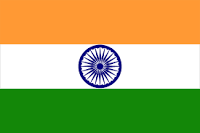
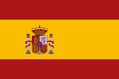
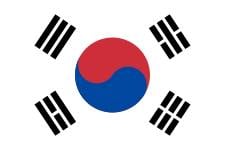
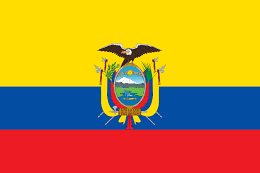
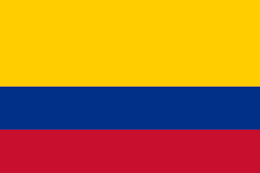
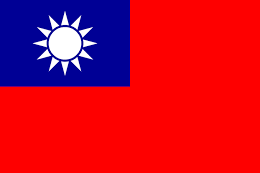
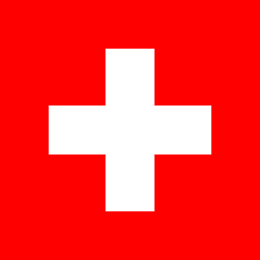
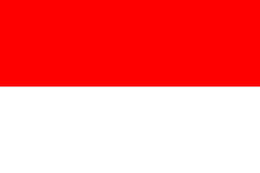
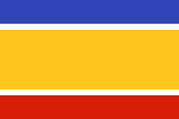
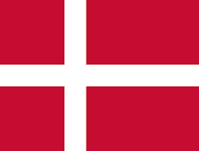
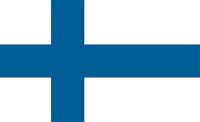
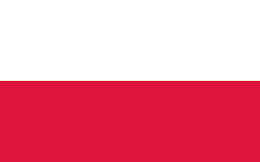
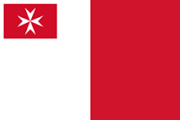
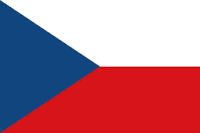
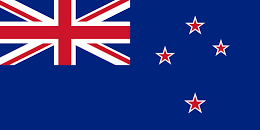
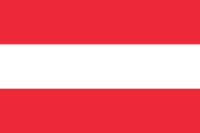
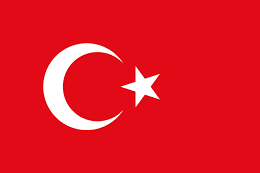
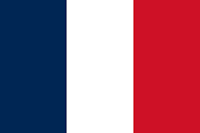
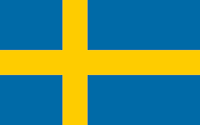
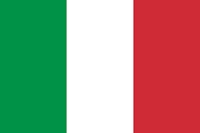
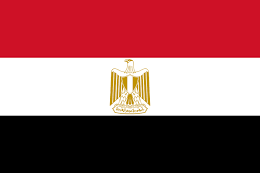
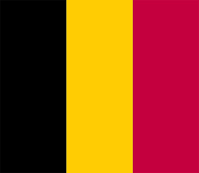
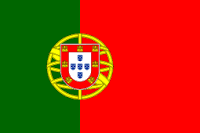
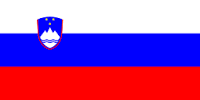
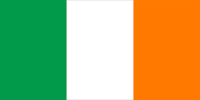
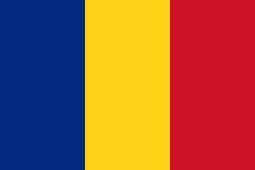
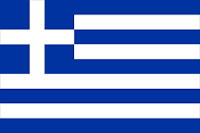
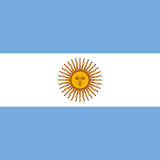
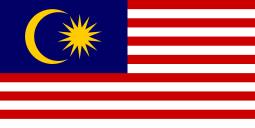
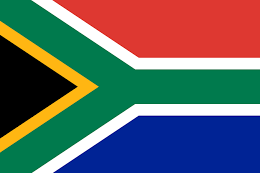
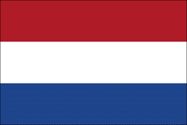
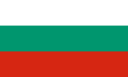
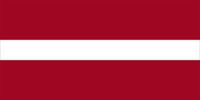
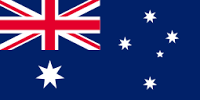
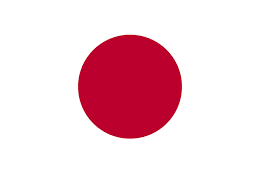
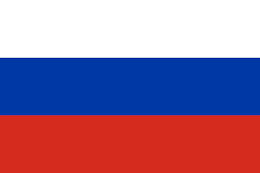