In this chapter, we reviewed the topics that we discussed in this book. We briefly went through the trends that are observable in the marketing industry and how data science and machine learning are becoming more and more important in marketing. Then, we reviewed a typical data science workflow, where you start with problem definition, then move onto data collection, preparation, and analysis, and finally move to feature engineering and model building. While working on future data science projects, it will be worthwhile to keep the workflow diagram we looked at in the back of your head and when stuck with what to do next, refer back to this diagram for ideas. We have also shared some of the challenges you might face when working with real-world datasets. The three main challenges we covered were data issues, infrastructure issues, and choosing the right model. More specifically...
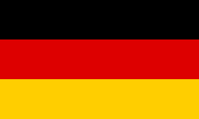
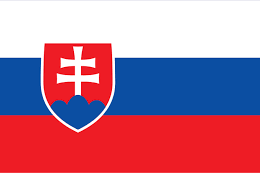
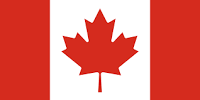
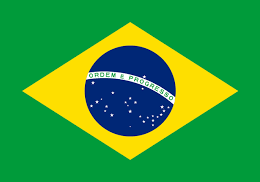

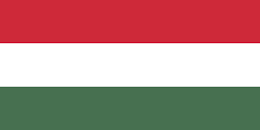
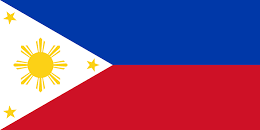
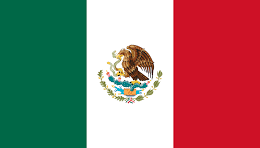
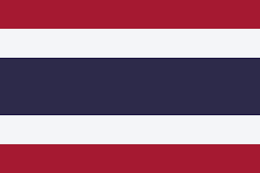
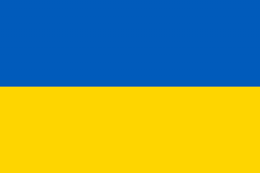
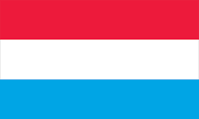
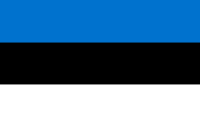
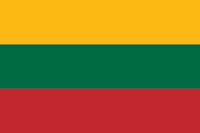
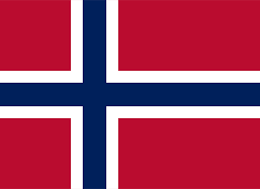
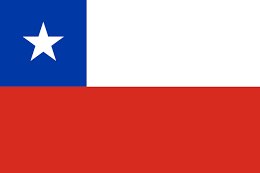
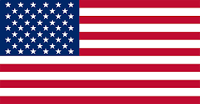
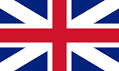
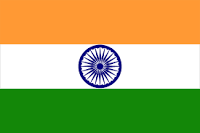
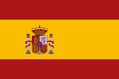
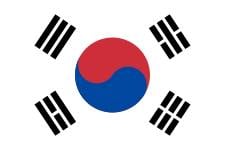
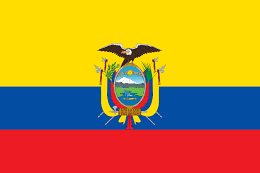
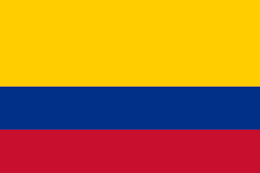
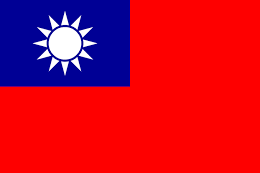
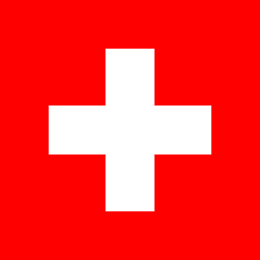
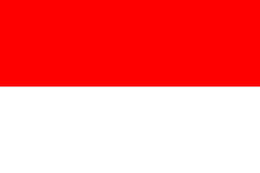
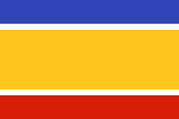
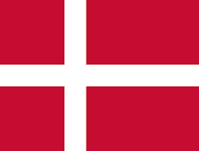
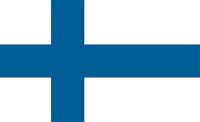
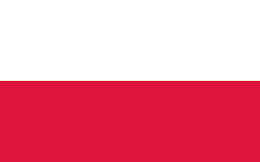
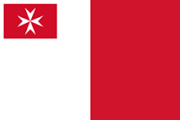
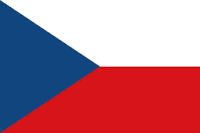
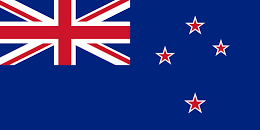
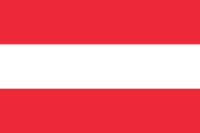
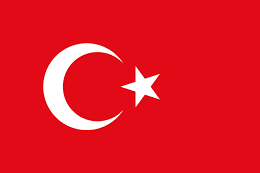
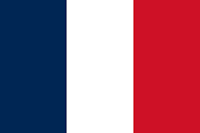
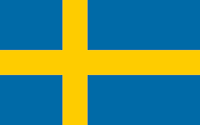
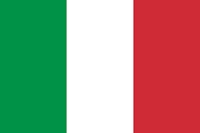
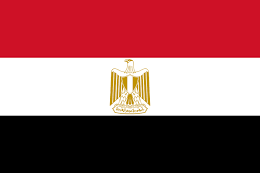
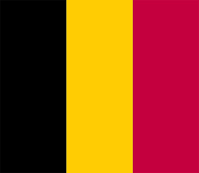
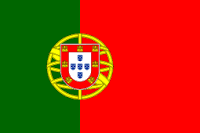
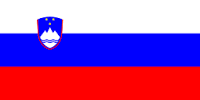
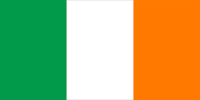
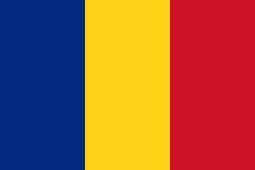
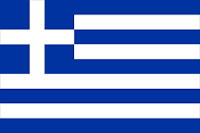
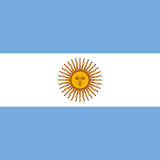
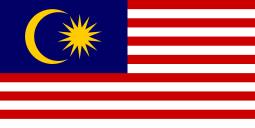
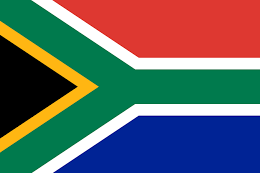
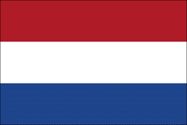
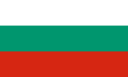
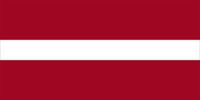
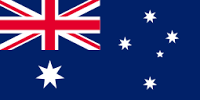
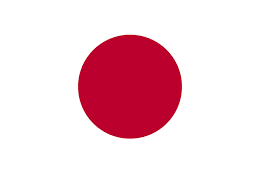
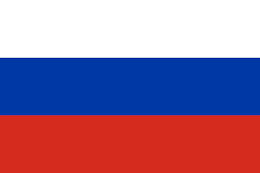