Reporting pattern
Design separate column families in Cassandra for the reporting needs. Keep the operational data in RDBMS and the reporting data in Cassandra column families. The most important reason why this separation of concerns is good practice is because of the tunable consistency feature in Cassandra. Depending on the use cases and for performance reasons, various consistency parameters may be used in the Cassandra column families and in the applications using Cassandra for read and write operations. When data ingestion happens in Cassandra, the consistency parameters have to be tuned to have fast writes.
The consistency parameters used for fast writes may not be suitable for fast reads. So, it is better to design separate column families for reporting purposes. From the same operational data, if various types of reports have to be created, it may be wise to create separate column families for these different reporting requirements. It is also a common practice to preprocess the operational data to generate fast reports. Historical reporting, data archival, statistical analysis, providing data feeds, inputs for machine learning algorithms such as recommendation systems and so on benefit a lot from accessing data from the Cassandra column families specifically designed for reporting.
Motivations/solutions
Coming from the RDBMS world, people rarely think about the reporting needs in the very beginning. The main reason behind that is, there is good flexibility with the SQL queries, and you can pretty much get any kind of report from the RDBMS tables because you may join RDBMS tables. So, the application designers and data modelers focused on the data and the business logic first. Then, they came to thinking about the reports. Even though this strategy worked, it introduced lots of application performance problems either toward the end of the application development or when the data volume has grown beyond certain limit. The best thing to do in these kind of situations is to design separate Cassandra column families for the reporting needs.
In social media and real media applications commonly used by millions of users at a given time, reporting needs are huge. Most importantly, the performance of those reports are even more paramount. For example, in a movie streaming website, users post videos. Users follow other users. The followers like the videos posted by the users whom they are following. Now, take the two important views in the website: the first one that gives the list of videos liked by a given user, the second one gives the list of users liking a given video. In the RDBMS world, it is fine to use one table to store the data items to generate these two reports. In Cassandra, it is better to define two column families to generate these two reports. You may be wondering why this can't be achieved by reading out of a single column family. The reason is that the sorting order matters in Cassandra, and the records are stored in sorted order.
In Cassandra, it may be necessary to create different column families to produce different reports. As mentioned earlier, it is fine to write the same piece of data into multiple Cassandra column families. There is no need to panic as the latest versions of Cassandra comes with batching capability for data manipulation operations. In this way, the data integrity may be ensured. It may not be as flexible and powerful as many RDBMS, but there are ways to do this in Cassandra. For example, take the case of a hypothetical Twitter-like application.
Users tweet and the tweets have to be shown differently in the default view, differently in the listing using hashtags, differently in the user time line, and so on. Assuming that Cassandra is being used for storing the tweets, you may design different Cassandra column families for materializing these different views. When a new tweet comes in, that record will be inserted into all these different column families. To maintain the data integrity, all these INSERT
statements may be designed as atomic unit of statements by enclosing them between the BEGIN BATCH
and APPLY BATCH
statements of CQL, as batches are atomic by default.
When it comes to reporting, RDBMS fails miserably in many use cases. This is seen when the report data is produced by many table joins, and the number of records in these tables are huge. This is a common situation when there is complex business logic to be applied to the operational data stored in the RDBMS before producing the reports. In such situations, it is always better to go with creating separate column families in Cassandra for the reporting needs. This may be done in two ways. The first method is the online way, in which the operational data is transformed into analytical or reporting data and stored in Cassandra column families. The second method is the batching way. In regular intervals, transform the operational data into analytical or reporting data in a batch process with business logic processors storing the data in Cassandra column families.
Predictive analytics or predictive modeling is very common these days in the commercial and scientific applications. A huge amount of operational data is processed, sliced, and diced by the data scientists using various machine learning algorithms and produces outputs for solving classification, regression, and clustering problems. These are highly calculation-intensive operations and deals with huge amount of operational data. It is practically impossible to do these calculations on the fly for the instantaneous requests from the users of the system. In this situation, the best course of action is to continually process the data and store the outputs in Cassandra column families.
Tip
There is a huge difference between the reporting data and analytical data. The former deals with producing the data from the data store as per the user's selection criteria. The latter deals with the data to be processed to give a result to the user as an answer to some of their questions, such as "why there is a budget overrun this year?", "when the forecast and the actual started deviating?" and so on. Whenever such questions are asked, the analytical data is processed and a result is given.
Graphical representation of vital statistics is an important use case in many of the applications. These days many applications provide huge amount of customization for the users to generate user-defined graphs and charts. For making this happen, there are sophisticated graphing and charting software packages that are available in the market. Many of these software packages expect the data in certain format to produce the graphical images. Most of the time, the operational data may not be available in the specific format suitable for these specialized software packages. In these situations, the best choice of any application designer is to transform the operational data to suit the graphing and charting requirements. This is a good opportunity to use separate Cassandra column families to store the data specific for the graphing and charting.
Operational and historical reporting are two different types of needs in many applications. Operational data is used to report the present, and historical data is used to report the past. Even in the operational reporting use cases, there are very good reasons to separate the reporting data to different Cassandra column families. In the historical reporting use cases, it is even more important because the data grows over a period of time. If the velocity of the operational data is very high, then the historical reporting becomes even more cumbersome. Bank account statements, credit card statements, payslips, telephone bills, and so on, are very good examples of historical reporting use cases.
In the past, organizations used to keep the historical data as long as it is needed in the system for the compliance and governance requirements. Things have changed. Many organizations have started keeping the data eternally to provide value-added services as the storage is becoming cheaper and cheaper these days. Cassandra is a linearly scalable NoSQL data store. The more storage requirements you have, the more nodes you can add to its cluster as and when you need without any need to reconfigure or any downtime, and the cluster will start making use of the newly added nodes. Read operations are really fast, so reporting is a highly used use case supported by Cassandra column families with the clever use of tunable consistency.
In the old generation applications, operational data is archived for posterity and auditing purposes. Typically, after its decided lifetime, operational data is taken offline and archived so that the data growth is not affecting the day-to-day operations of the system. The main reason why this archival is needed is because of the constraints in the data storage solutions and the RDBMS used. Clustering and scaling out of RDBMS-based data store is very difficult and extremely expensive. The new generation NoSQL data stores such as Cassandra are designed to scale out and run on commodity hardware. So, the need to take the data offline doesn't exist at all. Design Cassandra column families to hold the data marked for archival and they can be kept online for ever. Watch out the data growth and keep on adding more and more Cassandra nodes into the Cassandra cluster as and when required.
The emergence of cloud as the platform of choice and proliferation of Software as a Service (SaaS) applications introduced one more complexity into the application design, which is multitenancy. Multitenancy promotes the use of one instance of an application catering to the needs of multiple customers. Most of these SaaS applications give its customers a good amount of customization in terms of the features and reports. The service providers who host these SaaS have a new challenge of maintaining customer specific data and reports. This is a good use case where separate Cassandra column families to be used for maintaining customer-specific data needed for the tailor made reports.
Financial exchanges, trading systems, mobile phone services, weather forecasting systems, airline reservation systems, and the like produce high-volume data and process them with subsecond response to their end users. Obviously, the reporting needs are also huge in those applications in terms of the number of records to be processed and the complexity of data processing required. In all these systems-separating operations data and reporting data is a very important requirement. Cassandra is a good fit in all these reporting use cases.
Data transformation is an important step in producing many reports. In the enterprise application integration use cases, often one application will have to provide data to another application in a certain format. XML and JSON are two important data exchange formats. In applications with service-oriented architecture, whether they consume or produce services, data is required in specific formats. Whatever the technology used to perform the transformation may be, because of the volume of the data, it is practically impossible to process these data as and when required on a real-time basis. Preprocessing is required in many situations to produce the data in specific formats. Even though RDBMS supports data types such as BLOB and CLOB to store huge chunk of data, often the limitations of RDBMS will take effect. NoSQL data stores such as Cassandra are designed to handle very sophisticated data types built using user-defined data types, and it is easy to use them for storing the preprocessed data for the future reporting purposes.
Providing data feeds to external systems is a very common requirement these days. This is a very effective mechanism for disseminating data asynchronously to the subscribers of the data through popular mechanisms such as RSS feeds. The data designated for the data feeds must be derived from the operational data. Cassandra column families may be designed to serve such requirements.
Best practices
Separation of operational and reporting data stores is a good idea in many cases, but care must be taken to check if it is violating any of the data integrity or business logic invariants in the system. Immutable data items are really good candidates for this separation because they are not going to be changed anytime in the future. It is better to keep the frequently changing data items in the operational data stores itself. In Cassandra, if column families are used only for reporting purposes, care must be taken on how to load the data into the column families. Typically, these column families will be optimized for fast reads. If too many writes into those column families are going to take place, the consistency parameters will have to be tuned very well so that it does not defeat the original purpose of creating those column families, which is to read data from it. It is very difficult to tune Cassandra column families to suit the needs of very fast writes and reads. One of these will need to be compromised. Since the original purpose of these column families is to provide fast reads, the speed of writes must be controlled. More detailed treatment on the tuning for fast reads and fast writes in Cassandra column families is given in the coming chapters of this book.
Example
Let's take the case of a normalized set of tables from an application using RDBMS, as shown in Figure 4. There are three tables in the relation. One stores the customer details, another one stores the order details, and the third one stores the order-line items. Assume that this is an operational table and the data size is huge. There is a one-to-many relation between the Customer
table and the Order
table. For every customer record in the Customer
table, there may be zero or more order records in the Order
table. There is a one-to-many relation between the Order
table and the OrderItems
table. For every order record in the Order table, there may be zero or more order item records in the OrderItems
table.
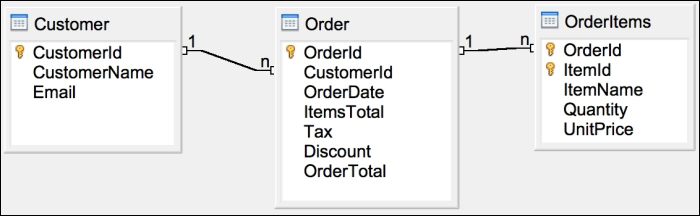
Figure 4
Assume that the requirement is to create a Cassandra column family to generate a monthly customer order summary report. The report should contain one record for each customer containing the order total for that month. The Cassandra column family will look like the one given in the Figure 5.
In the Cassandra column family MonthlyCustomerOrder
, a combination of CustomerId
, OrderYear
, and OrderMonth
columns form the primary key. The CustomerId
column will be the partition key. In other words, all the records for a given customer will be stored in one wide row of the column family.
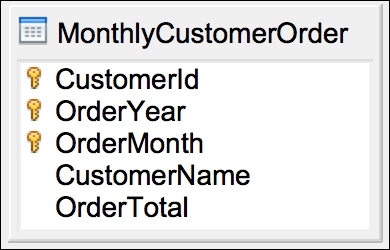
Figure 5
Assuming that the key space is created using the scripts given Figure 3, the following scripts given here will create only the required column family and then insert one record. Filling in the data in this column family may be done on a real-time basis or as a batch process. Since the data required for filling the Cassandra column family is not readily available from the RDBMS tables, a preprocessing needs to be done to prepare the data that goes into this Cassandra column family:
USE PacktCDP1; CREATE TABLE MonthlyCustomerOrder ( CustomerId bigint, OrderYear int, OrderMonth int, CustomerName text static, OrderTotal float, PRIMARY KEY (CustomerId, OrderYear, OrderMonth) ) WITH CLUSTERING ORDER BY (OrderYear DESC, OrderMonth DESC); INSERT INTO MonthlyCustomerOrder (CustomerId, OrderYear, OrderMonth, CustomerName, OrderTotal) VALUES (1,2015,6,'George Thomas', 255.5);
The following script gives the details of how the row in the Cassandra column family is physically stored. The commands given here are to be executed in the Cassandra CLI interface:
USE PacktCDP1; list MonthlyCustomerOrder; Using default limit of 100 Using default cell limit of 100 RowKey: 1 => (name=2015:6:, value=, timestamp=1434231618305061) => (name=2015:6:customername, value=476f657267652054686f6d6173, timestamp=1434231618305061) => (name=2015:6:ordertotal, value=437f8000, timestamp=1434231618305061) 1 Row Returned. Elapsed time: 42 msec(s).
The CQL SELECT
command given in Figure 6 gives the output in a human readable format:
SELECT * FROM MonthlyCustomerOrder;

Figure 6