We often face this preconceived notion of rewards-based learning or training as comprising of an action being completed, followed by a reward, be it good or bad. While this notion of RL works completely fine for a single action-based task, such as the old multi-arm bandit problem we looked at earlier, or teaching a dog a trick, recall that reinforcement learning is really about an agent learning the value of actions by anticipating future rewards through a series of actions. At each action step, when the agent is not exploring, the agent will determine its next course of action based on what it perceives as having the best reward. What is not always so clear is what those rewards should represent numerically, and to what extent that matters. Therefore, it is often helpful to map out a simple set of reward functions that describe the learning behavior...
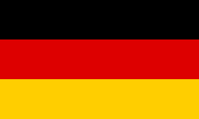
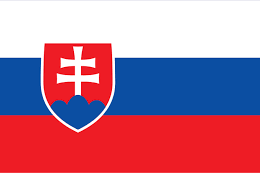
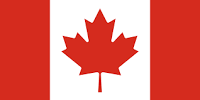
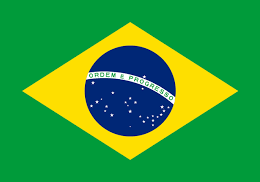

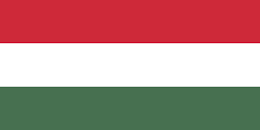
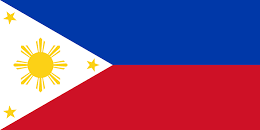
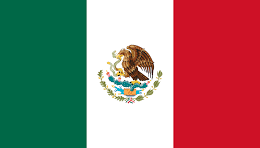
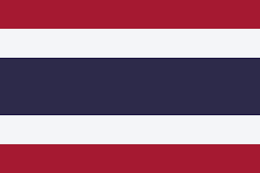
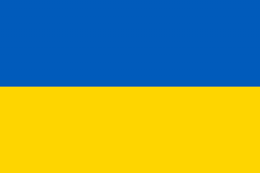
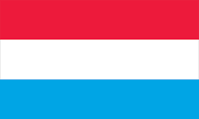
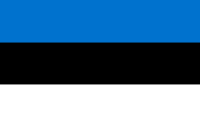
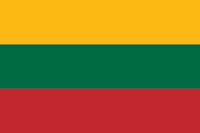
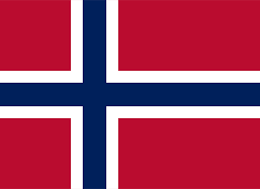
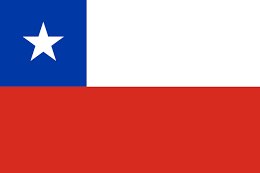
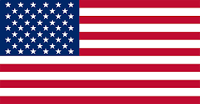
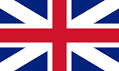
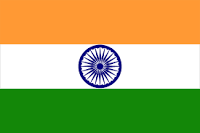
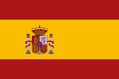
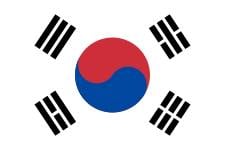
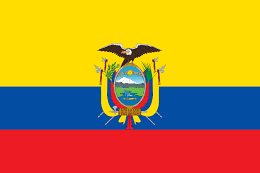
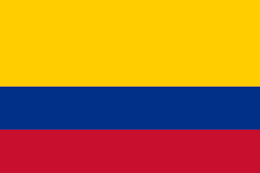
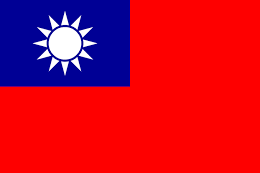
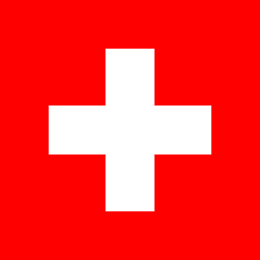
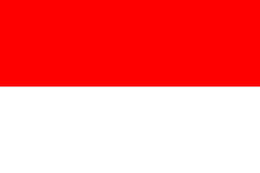
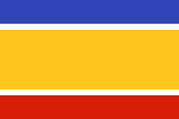
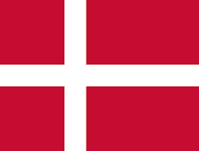
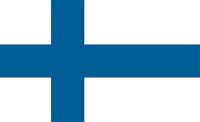
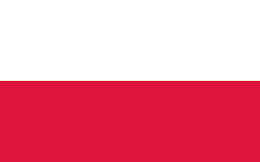
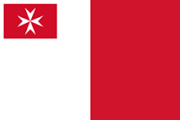
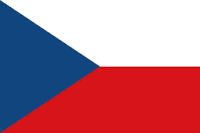
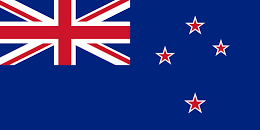
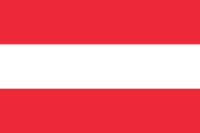
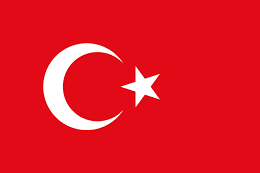
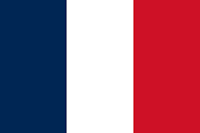
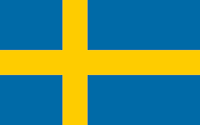
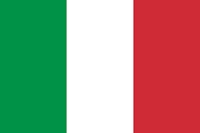
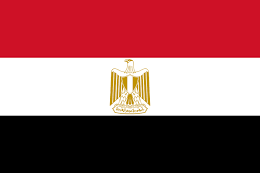
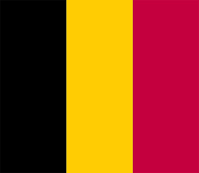
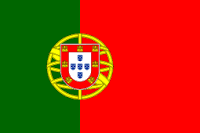
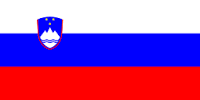
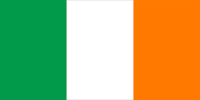
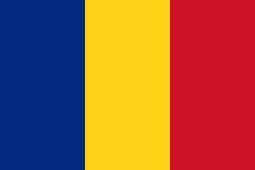
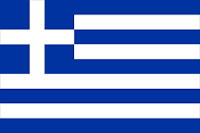
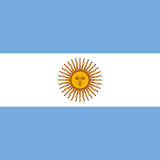
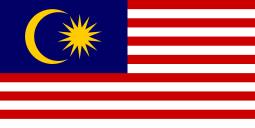
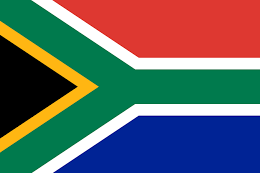
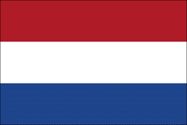
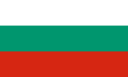
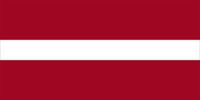
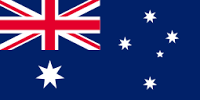
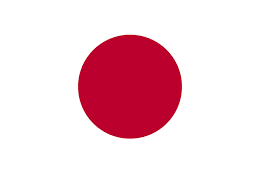
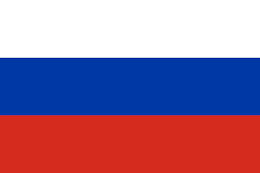