- Prove that the SSE converges with zero when we increase the number of desired clusters, K.
- Using the example in this chapter, change the number of centroids and see how the final result varies.
- What will happen if we move each cluster closer together?
- There are multiple enhanced initialization methods of K-means. Let's try out the following initial centroids:
- Forgy method: Choose K observations from the data points as centroids.
- Random partition: Assign each data point to a cluster randomly.
- Replace the naive K-means implementation that we used with the implementation in machinelearn.js.
- It's not guaranteed that the K-means algorithm can be converged with the global optima. Illustrate where K-means only returns poor results.
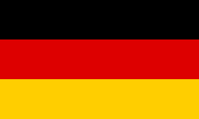
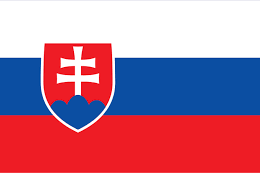
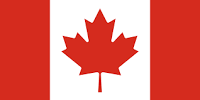
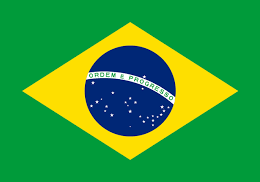

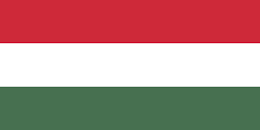
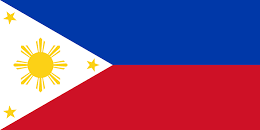
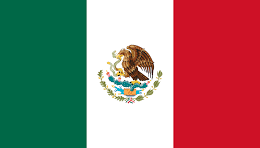
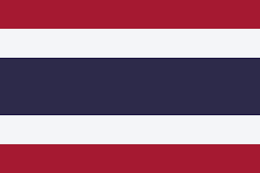
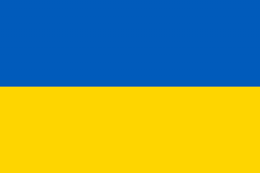
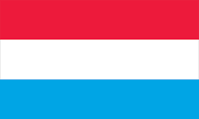
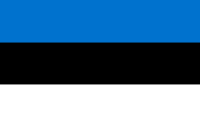
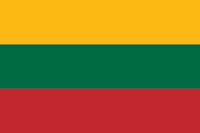
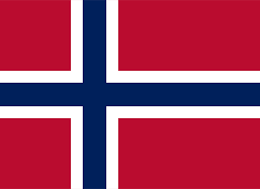
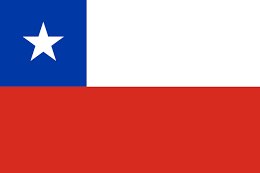
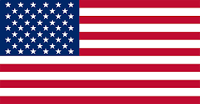
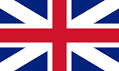
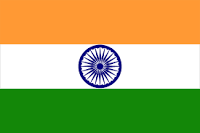
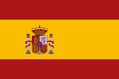
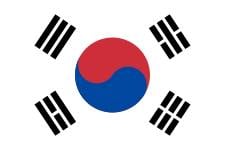
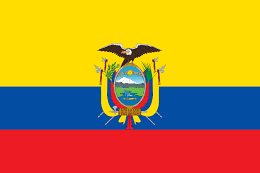
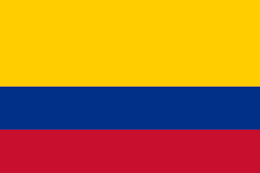
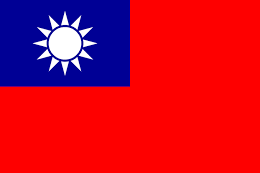
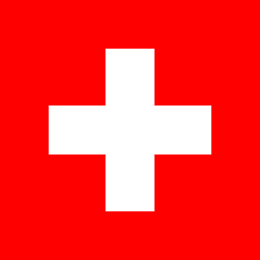
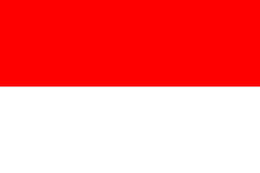
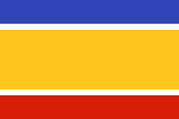
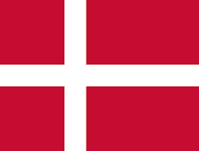
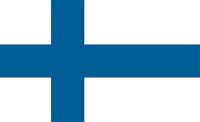
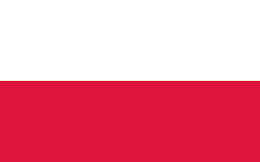
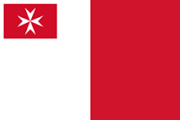
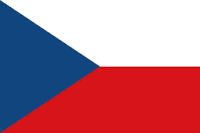
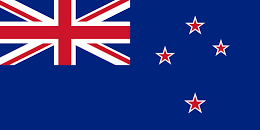
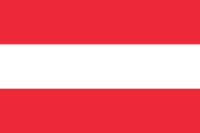
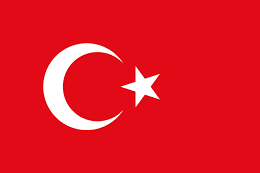
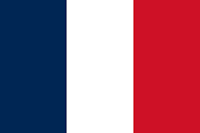
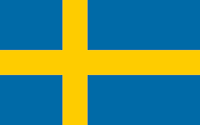
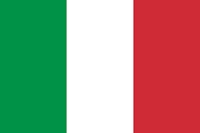
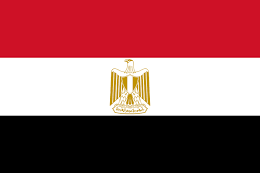
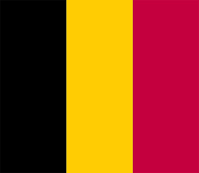
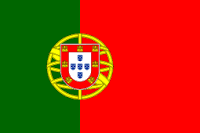
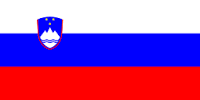
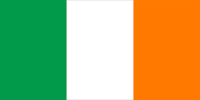
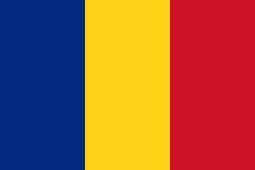
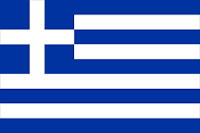
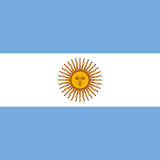
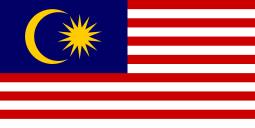
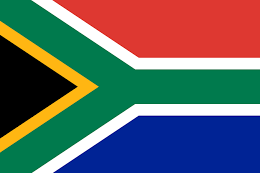
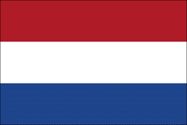
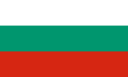
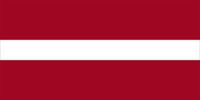
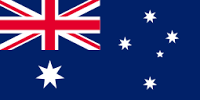
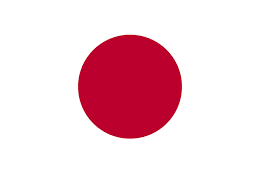
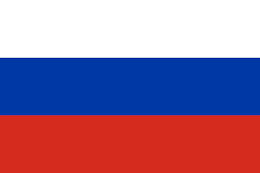