Now we have a high-level understanding of what generative networks entail, we can focus on a specific type of generative models. One of them is the VAE, proposed by both Kingma and Welling (2013) as well as Rezende, Mohamed, and Wierstra (2014). This model is actually very similar to the autoencoders we saw in the last chapter, but they come with a slight twist—well, several twists, to be more specific. For one, the latent space being learned is no longer a discrete one, but a continuous one by design! So, what's the big deal? Well, as we explained earlier, we will be sampling from this latent space to generate our outputs. However, sampling from a discrete latent space is problematic. The fact that it is discrete implies that there will be regions in the latent space with discontinuities, meaning that if these regions were to be randomly sampled...
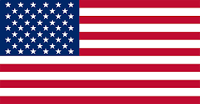
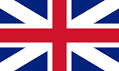
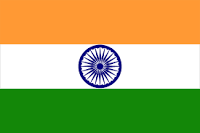
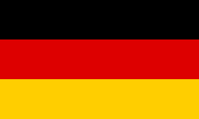
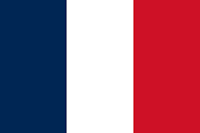
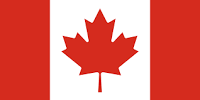
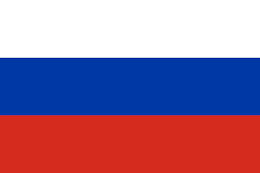
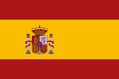
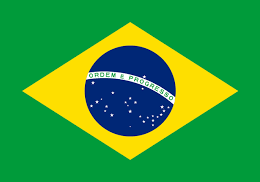
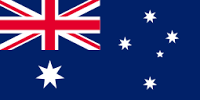

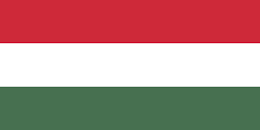
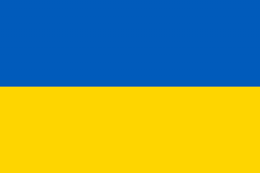
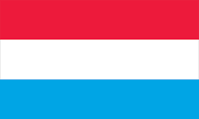
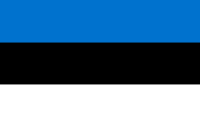
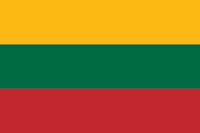
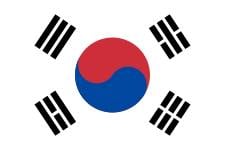
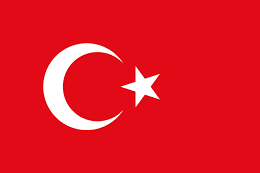
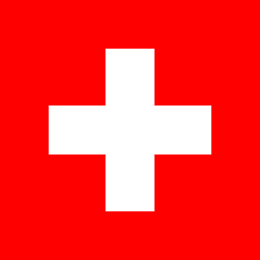
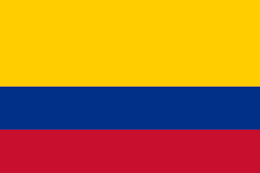
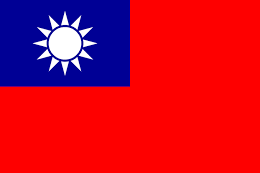
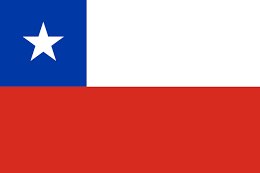
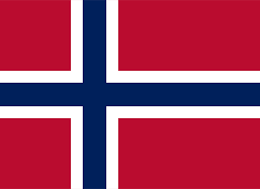
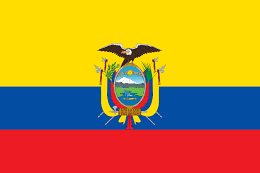
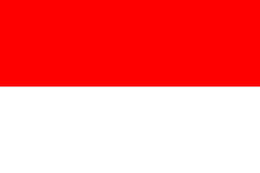
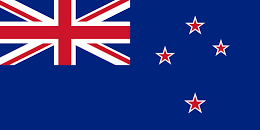
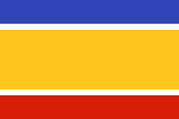
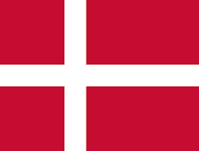
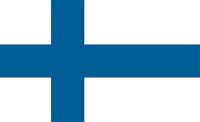
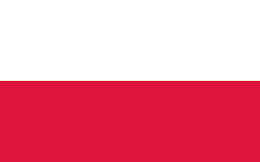
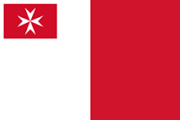
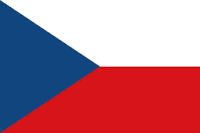
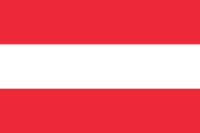
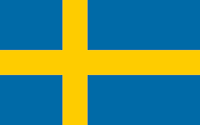
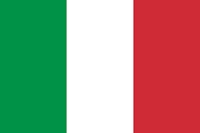
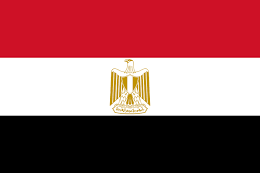
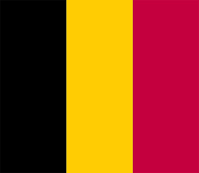
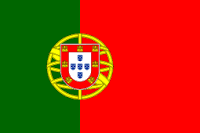
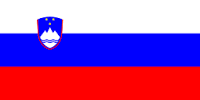
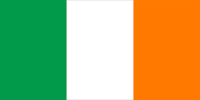
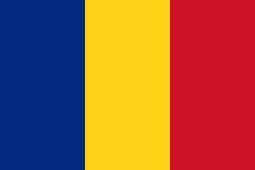
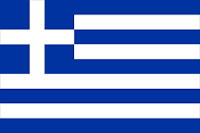
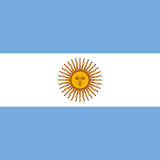
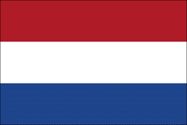
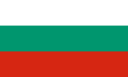
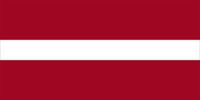
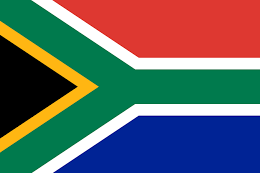
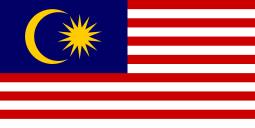
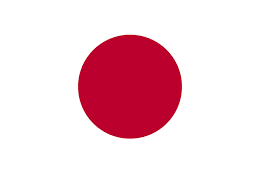
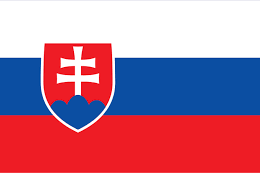
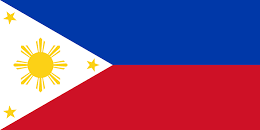
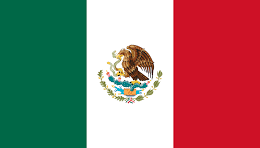
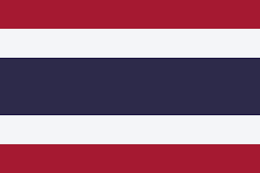