As of today, policy gradient is one of the most used RL algorithms. Research has shown that when properly tuned, they perform better than DQNs and, at the same time, do not suffer from excessive memory and computation disadvantages. Unlike Q learning, policy gradients use a parameterized policy that can select actions without consulting a value function. In policy gradients, we talk about a performance measure η(θp); the goal is to maximize the performance and hence the weights of the NN are updated according to the gradient ascent algorithm. However, TensorFlow does not have a maximum optimizer, thus we use the negative of the gradient of performance, -∇η(θp), and minimize it.
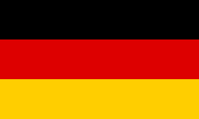
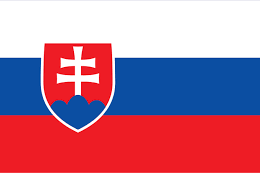
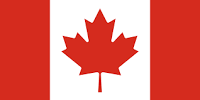
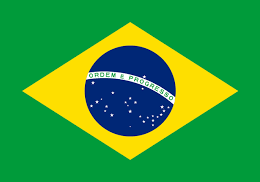

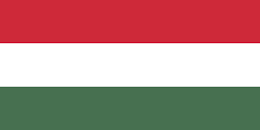
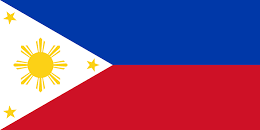
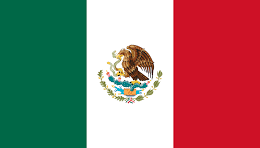
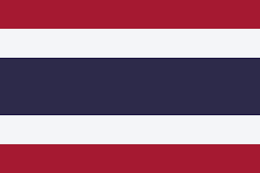
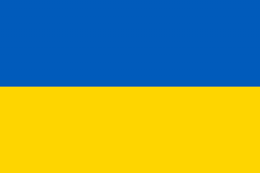
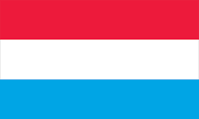
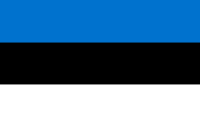
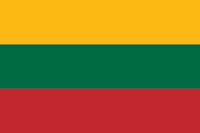
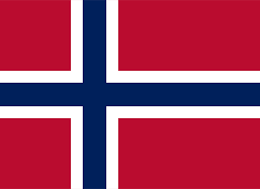
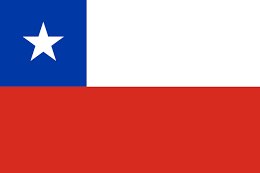
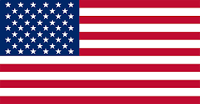
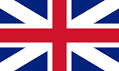
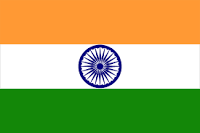
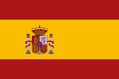
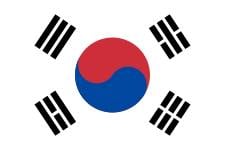
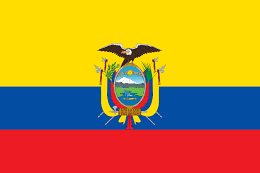
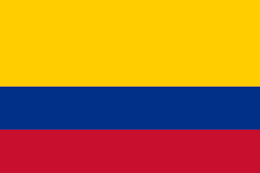
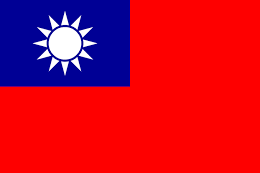
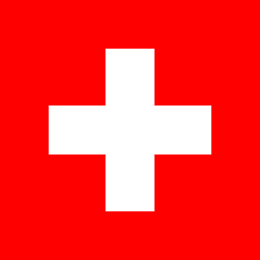
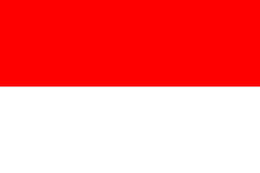
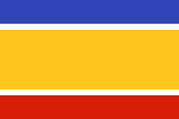
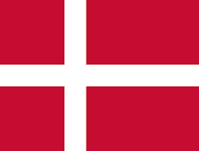
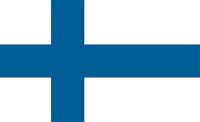
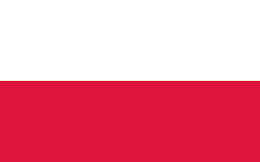
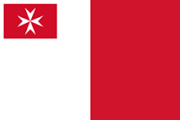
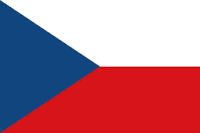
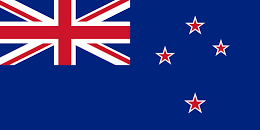
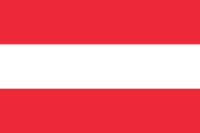
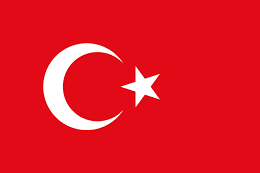
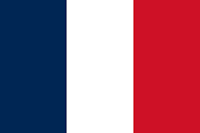
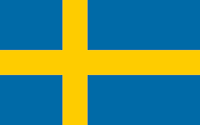
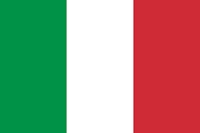
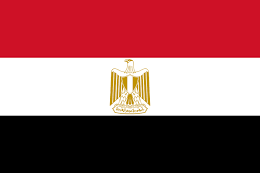
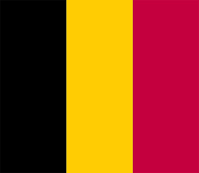
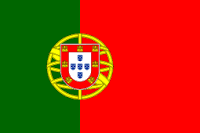
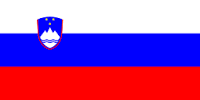
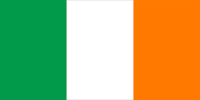
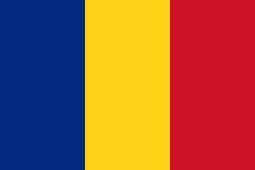
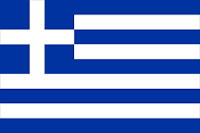
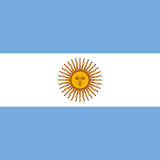
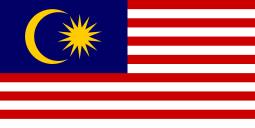
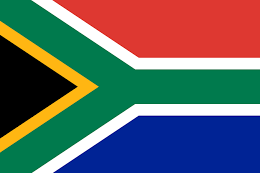
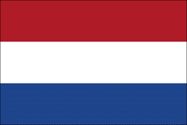
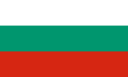
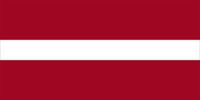
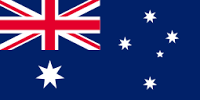
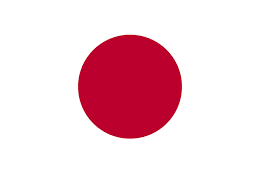
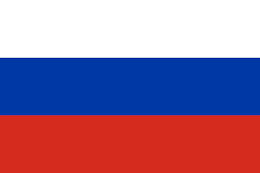