Keras is a Python library that provides a simple and clean way to create a range of deep learning models. Keras code was released under the MIT license. Keras has been structured based on austerity and simplicity, and it provides a programming model without ornaments that maximizes readability. It allows expressing neural networks in a very modular way, considering a model like a sequence or a single graph. This is a good approximation, because the components of a deep learning model are discrete elements that can be arbitrarily combined. The new components are easily aggregated and modifiable within the framework designed for engineers, to quickly test and explore new ideas. Last but not least, using the Python programming language provides constructs that allow clear programming on both a small and large scale. In the following screenshot, we can see the Keras official home page (https://keras.io/):
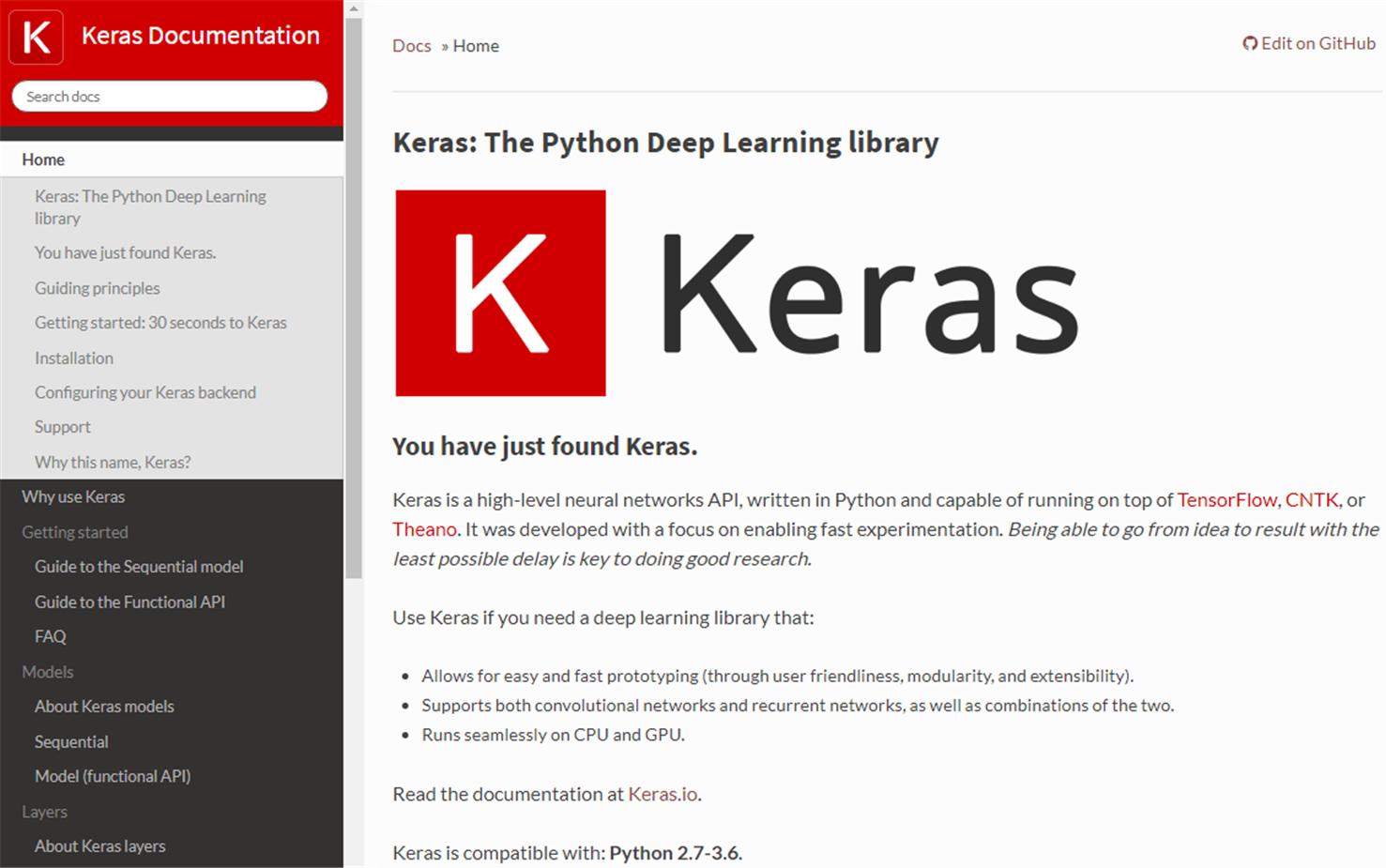
Ease of use is the strongpoint of Keras. During the design phase, the user has been the focus of attention of the developers, producing a product that reduces the user's work via simple and consistent APIs. In this way, the number of actions necessary to solve common use cases is reduced. In addition, the results are returned in a clear manner, making the identification of possible errors very simple.
In Keras, a model is represented by a sequence of autonomous and completely configurable modules that can be connected with the lowest possible number of restrictions. Everything in Keras is a module—neural layers, cost functions, optimizers, initialization schemes, activation functions, and regularization schemes. These independent modules can be combined to create new, more complex models.
All modules available in Keras are simple to add, and so are new classes and functions in a programming language. In addition, the modules are already available, and are accompanied by numerous examples that explain their practical use. But Keras is not limited to the availability of the built-in modules. The user will be able to easily create new modules, making Keras an easily extensible environment.
The keras library bases its technology on the levels that are used to manage input and output. An application in Keras can be implemented through the following four simple steps:
- Prepare input and output data.
- Create the first level to manage the input data.
- Set up intermediate levels to perform the analysis.
- Create the output level to manage the targets.
Keras works as a specific high-level API for neural networks. It can act as a user interface and can extend the functionality of other deep learning framework backends on which it runs. Thanks to this feature, Keras has become a wrapper for migration between frameworks. Not only can algorithms and models of neural networks for deep learning be exchanged, but also networks and preliminary weights.
Wrapper libraries consist of a thin layer of code that translates a library's existing interface into a compatible interface.
On the other hand, since Keras is autonomous, it can be used without having to interact with the backend framework on which it is running. Keras has its own chart data structures for defining computational charts; it is not based on the data structures of the underlying backend framework. This way, you will not have to learn how to program the backend framework.
Keras is easy to learn and use. Using Keras is like working with Lego blocks, you just have to put in sequence a series of compatible modules. It was created so that people can quickly perform the experimental phase of the model using a highly modular and extensible framework. Keras focuses on defining levels for the neural network. You do not have to deal with tensors, but it's easy to write with less code.