Attention
Attention is one of the key breakthroughs in machine translation that gave rise to better working NMT systems. Attention allows the decoder to access the full state history of the encoder, leading to creating a richer representation of the source sentence, at the time of translation. Before delving into the details of an attention mechanism, let's understand one of the crucial bottlenecks in our current NMT system and the benefit of attention in dealing with it.
Breaking the context vector bottleneck
As you have probably already guessed, the bottleneck is the context vector, or thought vector, that resides between the encoder and the decoder (see Figure 10.15):
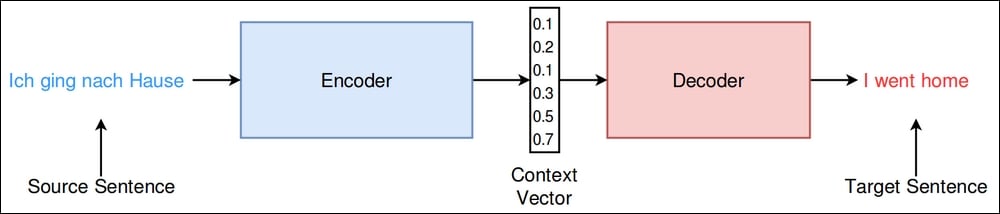
Figure 10.16: The encoder-decoder architecture
To understand why this is a bottleneck, let's imagine translating the following English sentence:
I went to the flower market to buy some flowers
This translates to the following:
Ich ging zum Blumenmarkt, um Blumen zu kaufen
If we are to compress this into a fixed length vector...