Big Data science and the role of Hadoop and Spark
Data science is all about the following two aspects:
- Extracting deep meaning from the data
- Creating data products
Extracting deep meaning from data means fetching the value using statistical algorithms. A data product is a software system whose core functionality depends on the application of statistical analysis and machine learning to the data. Google AdWords or Facebook's People You May Know are a couple of examples of data products.
A fundamental shift from data analytics to data science
A fundamental shift from data analytics to data science is due to the rising need for better predictions and creating better data products.
Let's consider an example use case that explains the difference between data analytics and data science.
Problem: A large telecoms company has multiple call centers that collect caller information and store it in databases and filesystems. The company has already implemented data analytics on the call center data, which provided the following insights:
- Service availability
- The average speed of answering, average hold time, average wait time, and average call time
- The call abandon rate
- The first call resolution rate and cost per call
- Agent occupancy
Now, the telecoms company would like to reduce the customer churn, improve customer experience, improve service quality, and cross-sell and up-sell by understanding the customers in near real-time.
Solution: Analyze the customer voice. The customer voice has deeper insights than any other information. Convert all calls to text using tools such as CMU Sphinx and scale out on the Hadoop platform. Perform text analytics to derive insights from the data, to gain high accuracy in call-to-text conversion, create models (language and acoustic) that are suitable for the company, and retrain models on a frequent basis with any changes. Also, create models for text analytics using machine learning and natural language processing (NLP) to come up with the following metrics while combining data analytics metrics:
- Top reasons for customer churn
- Customer sentiment analysis
- Customer and problem segmentation
- 360-degree view of the customer
Notice that the business requirement of this use case created a fundamental shift from data analytics to data science implementing machine learning and NLP algorithms. To implement this solution, new tools and techniques are used and a new role, data scientist, is needed.
A data scientist has a combination of multiple skill sets—statistics, software programming, and business expertise. Data scientists create data products and extract value from the data. Let's see how data scientists differ from other roles. This will help us in understanding roles and tasks performed in data science and data analytics projects.
Data scientists versus software engineers
The difference between the data scientist and software engineer roles is as follows:
- Software engineers develop general-purpose software for applications based on business requirements
- Data scientists don't develop application software, but they develop software to help them solve problems
- Typically, software engineers use Java, C++, and C# programming languages
- Data scientists tend to focus more on scripting languages such as Python and R
Data scientists versus data analysts
The difference between the data scientist and data analyst roles is as follows:
- Data analysts perform descriptive and diagnostic analytics using SQL and scripting languages to create reports and dashboards.
- Data scientists perform predictive and prescriptive analytics using statistical techniques and machine learning algorithms to find answers. They typically use tools such as Python, R, SPSS, SAS, MLlib, and GraphX.
Data scientists versus business analysts
The difference between the data scientist and business analyst roles is as follows:
- Both have a business focus, so they may ask similar questions
- Data scientists have the technical skills to find answers
A typical data science project life cycle
Let's learn how to approach and execute a typical data science project.
The typical data science project life cycle shown in Figure 1.4 explains that a data science project's life cycle is iterative, but a data analytics project's life cycle, as shown in Figure 1.3, is not iterative. Defining problems and outcomes and communicating phases are not in the iterations while improving the outcomes of the project. However, the overall project life cycle is iterative, which needs to be improved from time to time after production implementation.
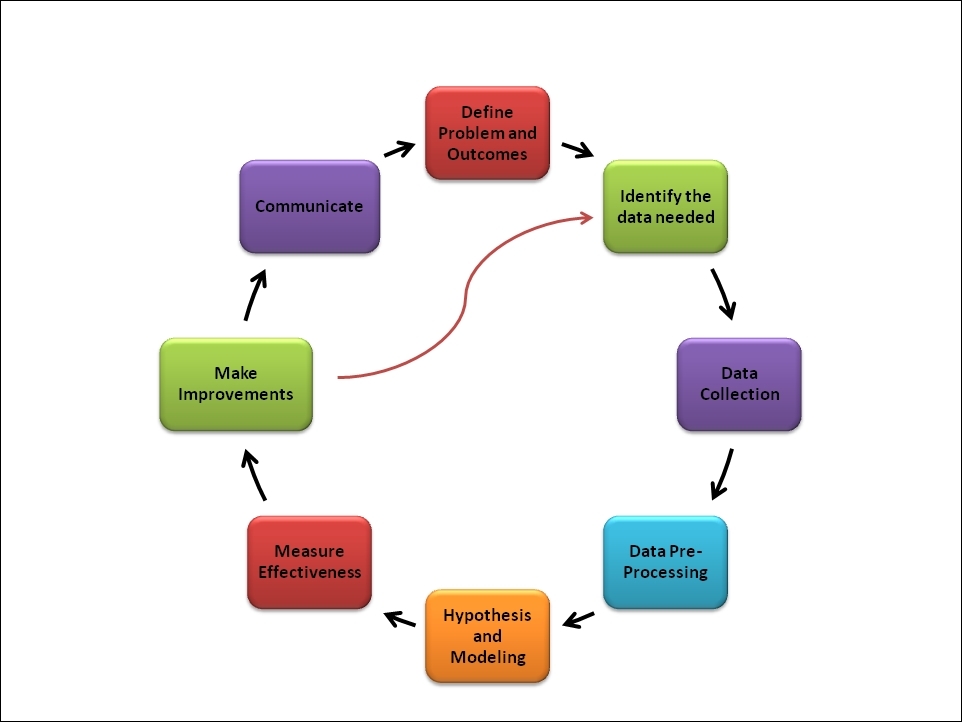
Figure 1.4: A data science project life cycle
Defining problems and outcomes in the data preprocessing phase is similar to the data analytics project, which is explained in Figure 1.3. So, let's discuss the new steps required for data science projects.
Hypothesis and modeling
Given the problem, consider all the possible solutions that could match the desired outcome. This typically involves a hypothesis about the root cause of the problem. So, questions around the business problem arise, such as why customers are canceling the service, why support calls are increasing significantly, and why customers are abandoning shopping carts.
A hypothesis would identify the appropriate model given a deeper understanding of the data. This involves understanding the attributes of the data and their relationships and building the environment for the modeling by defining datasets for testing, training, and production. Create the appropriate model using machine learning algorithms such as logistic regression, k-means clustering, decision trees, or Naive Bayes.
Measuring the effectiveness
Execute the model by running the identified model against the datasets. Measure the effectiveness of the model by checking the results against the desired outcome. Use test data to verify the results and create metrics such as Mean Squared Error (MSE) to measure effectiveness.
Making improvements
Measurements will illustrate how much improvement is required. Consider what you might change. You can ask yourself the following questions:
- Was the hypothesis around the root cause correct?
- Ingesting additional datasets would provide better results?
- Would other solutions provide better results?
Once you've implemented your improvements, test them again and compare them with the previous measurements in order to refine the solution further.
Communicating the results
Communication of the results is an important step in the data science project life cycle. The data scientist tells the story found within the data by correlating the story to business problems. Reports and dashboards are common tools to communicate the results.
The role of Hadoop and Spark
Apache Hadoop provides you with distributed storage and resource management, while Spark provides you with in-memory performance for data science applications. Hadoop and Spark have the following advantages for data science projects:
- A wide range of applications and third-party packages
- A machine learning algorithms library for easy usage
- Spark integrations with deep learning libraries such as H2O and TensorFlow
- Scala, Python, and R for interactive analytics using the shell
- A unification feature—using SQL, machine learning, and streaming together