The user-item rating matrix is usually a huge matrix. The one we got from our dataset here comprises 30,114 rows and 19,228 columns, and most of the values in this matrix (99.999%) are zeros. This is expected. Say you own a streaming service with thousands of movies in your library. It is very unlikely that a user will watch more than a few dozen of them. This sparsity creates another problem. If one user watched the movie The Hangover: Part 1 and another user watched The Hangover: Part 2, from the matrix's point of view, they watched two different movies. We already know that collaborative filtering algorithms don't use users or item features. Thus, it is not aware of the fact that the two parts of The Hangover movie belong to the same franchise, let alone knowing that they both are comedies. To deal with this shortcoming, we need to transform our user-item rating matrix. We want the new matrix, or matrices, to be smaller and to capture...
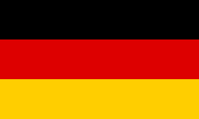
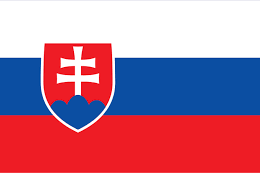
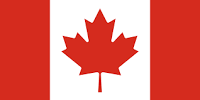
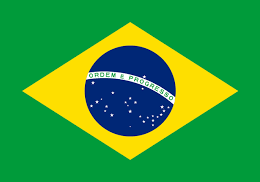

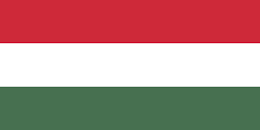
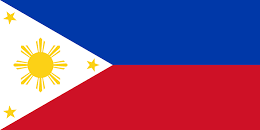
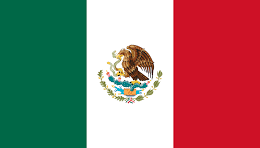
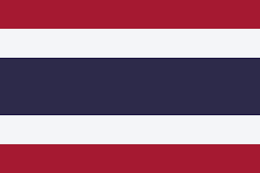
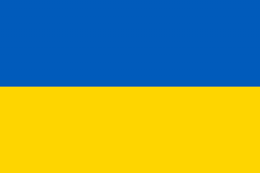
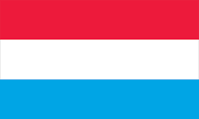
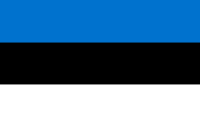
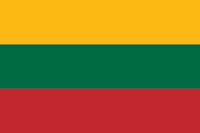
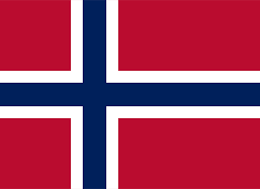
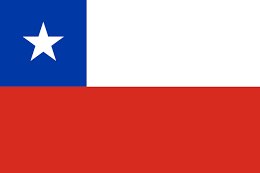
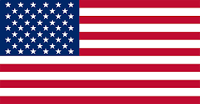
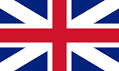
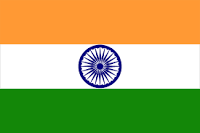
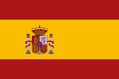
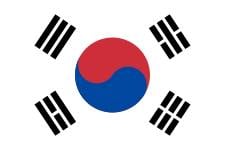
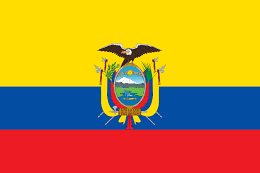
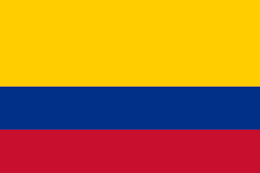
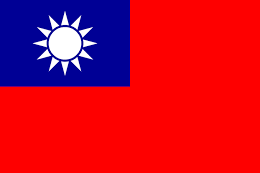
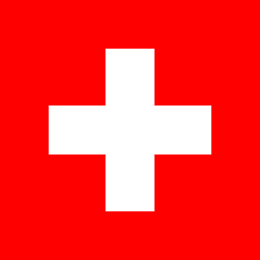
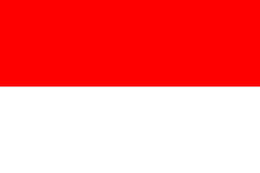
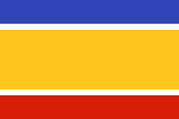
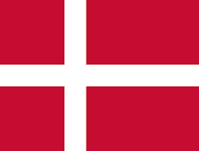
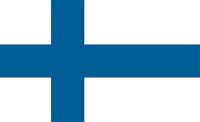
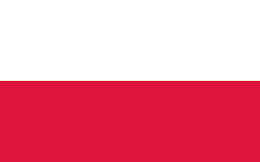
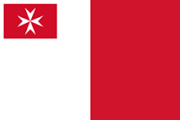
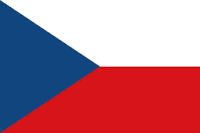
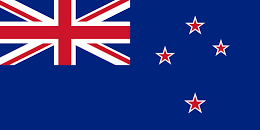
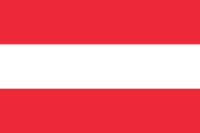
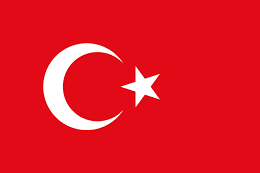
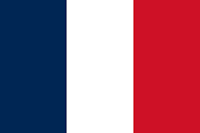
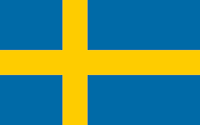
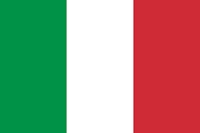
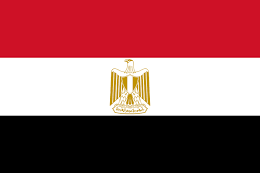
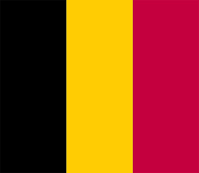
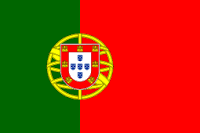
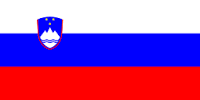
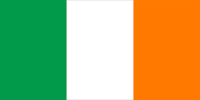
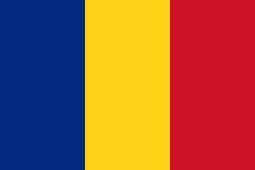
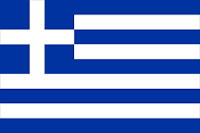
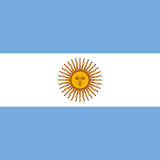
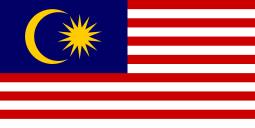
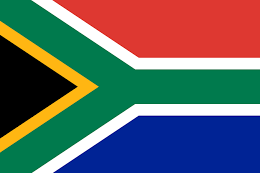
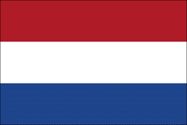
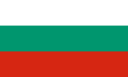
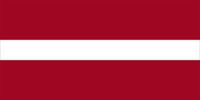
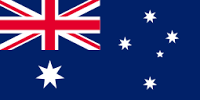
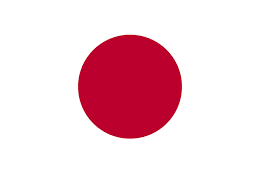
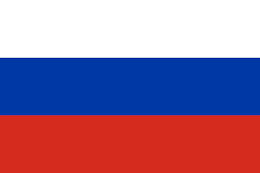