Classical statistical methods don't handle outliers well. The worst part is that even the most basic methods suffer this problem: for example, the sample mean, which is the maximum likelihood estimate for the µ parameter (assuming that the distribution is Gaussian), can be wrong even with a single contaminated observation. For example, the average between the numbers: 3, 4, and 5, is 4; and if we replace that last value (=5) with a new contaminated value =100, the new average will be =107/3. Let's introduce the concept of breakdown point for an estimator. The breakdown point (of an estimator) is the proportion of values that the estimator can handle before yielding wrong results. In the case of the mean that we just explained, the breakdown is 0; meaning that even a single contaminated observation would make the estimator give wrong results. The median...
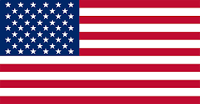
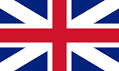
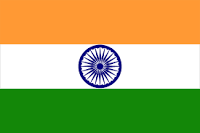
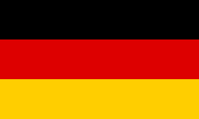
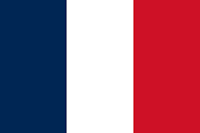
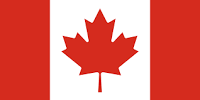
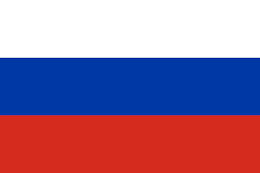
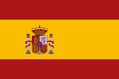
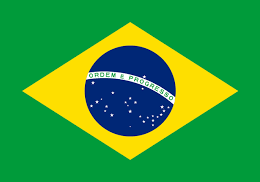
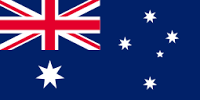

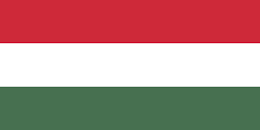
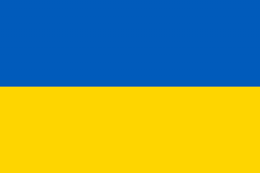
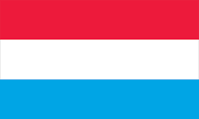
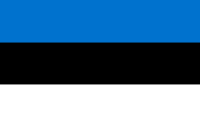
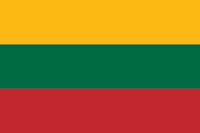
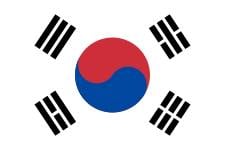
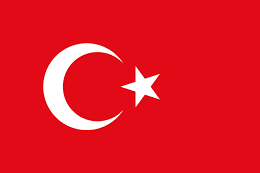
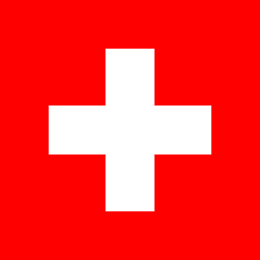
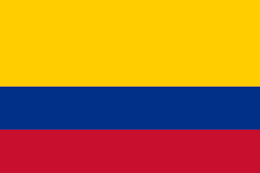
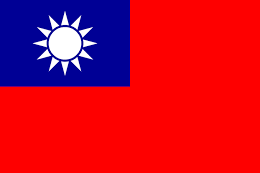
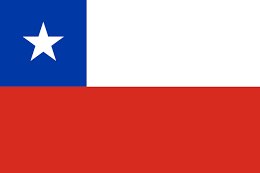
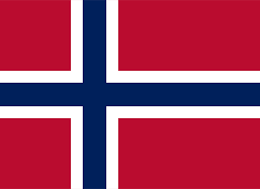
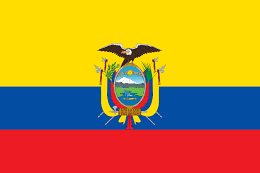
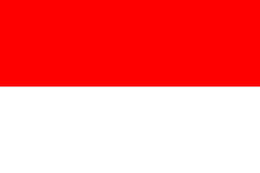
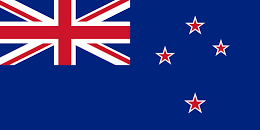
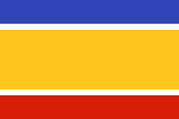
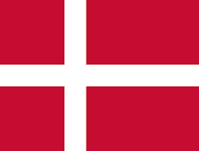
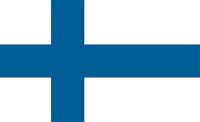
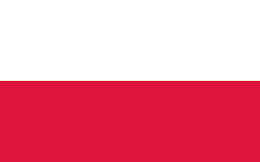
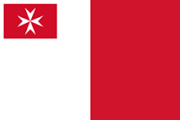
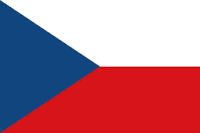
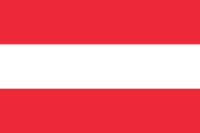
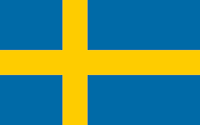
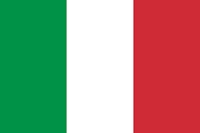
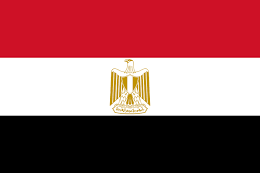
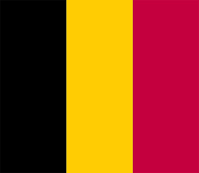
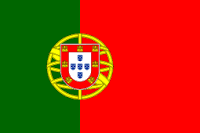
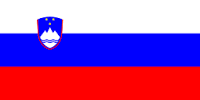
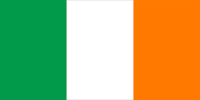
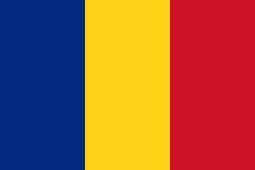
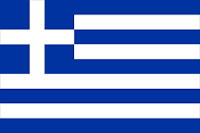
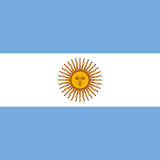
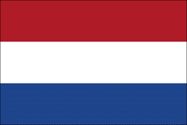
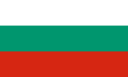
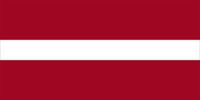
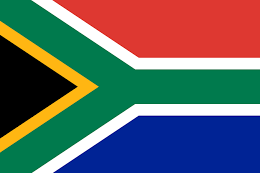
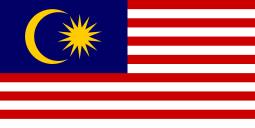
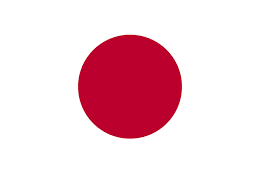
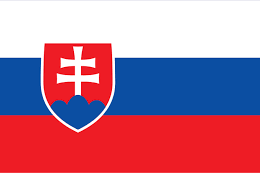
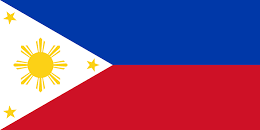
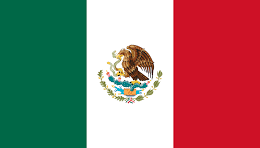
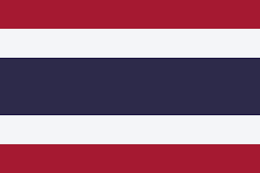