How can we ensure that our agent relies on a good balance of old and new strategies? This problem is made worse through the random initialization of weights for our Q-network. Since the predicted Q-values are a result of these random weights, the model will generate sub-optimal predictions at the initial training epochs, which in turn results in poor Q-value learning. Naturally, we don't want our network to rely too much on strategies it generates at first for given state-action pairs. Just like the dopamine addicted rat, the agent cannot be expected to perform well in the long term if it doesn't explore new strategies and expand its horizons instead of exploiting known strategies. To address this problem, we must implement a mechanism that encourages the agent to try out new actions, ignoring the learned Q-values. Doing so basically...
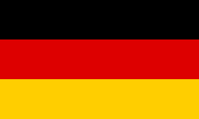
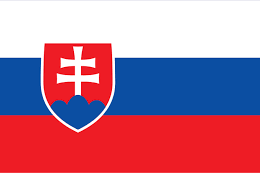
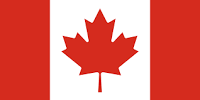
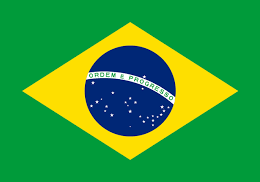

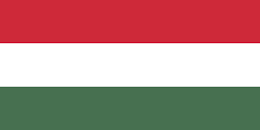
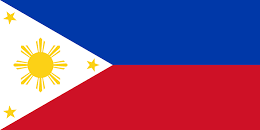
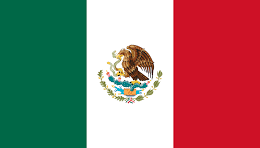
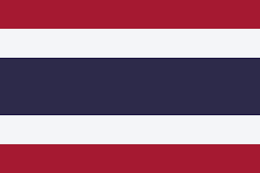
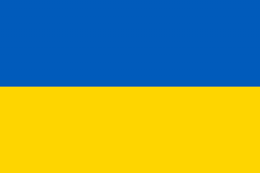
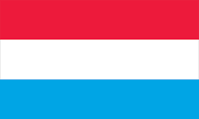
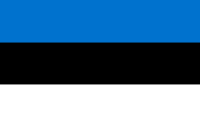
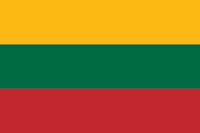
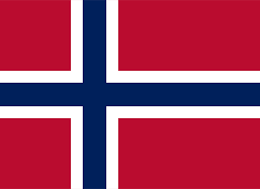
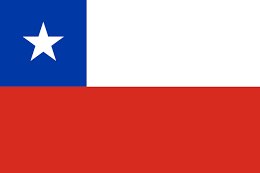
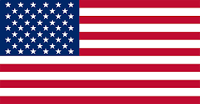
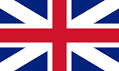
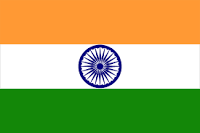
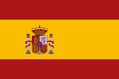
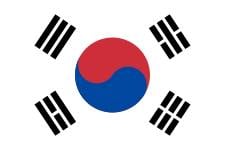
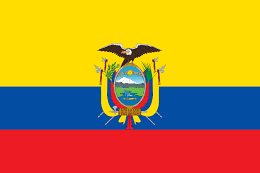
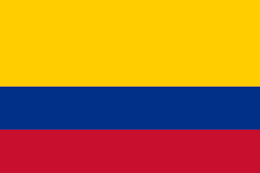
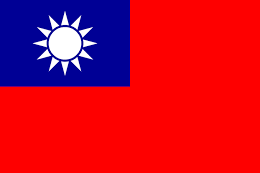
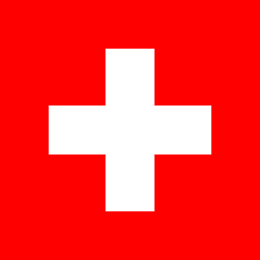
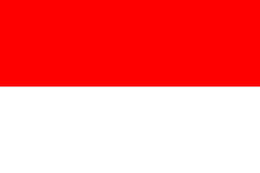
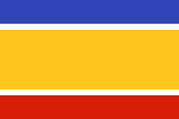
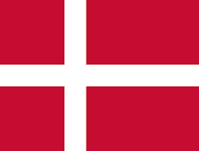
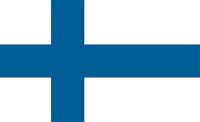
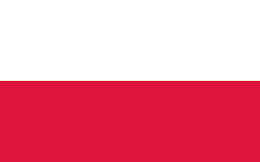
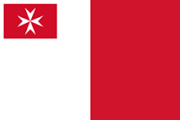
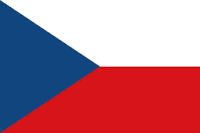
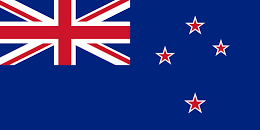
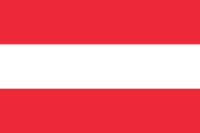
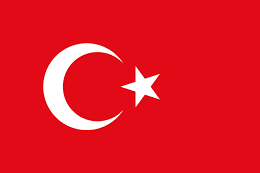
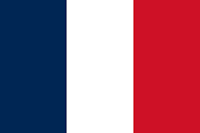
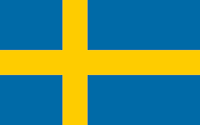
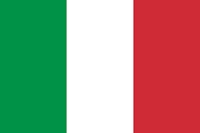
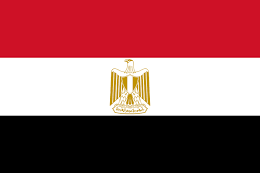
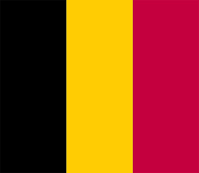
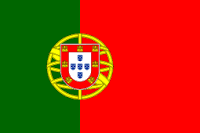
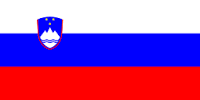
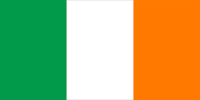
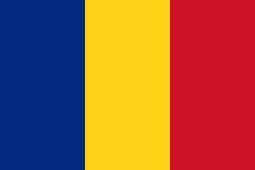
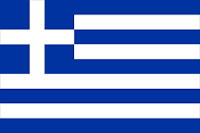
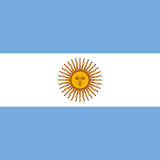
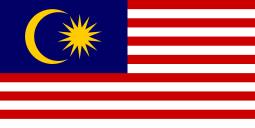
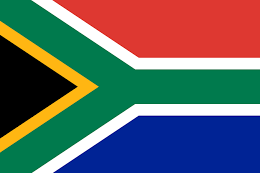
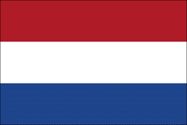
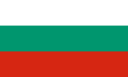
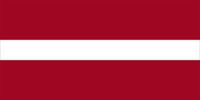
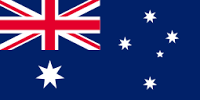
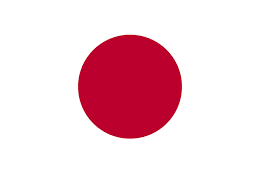
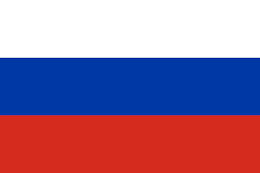