The data science Venn diagram
It is a common misconception that only those with advanced degrees such as a Ph.D. or math prodigies can understand the math/programming behind data science. This is false. Understanding data science begins with three basic areas:
- Math/statistics: This involves using equations and formulas to perform analysis
- Computer programming: This is the ability to use code to create outcomes on a computer
- Domain knowledge: This refers to understanding the problem domain (medicine, finance, social science, and so on)
The following Venn diagram provides a visual representation of how these three areas of data science intersect:
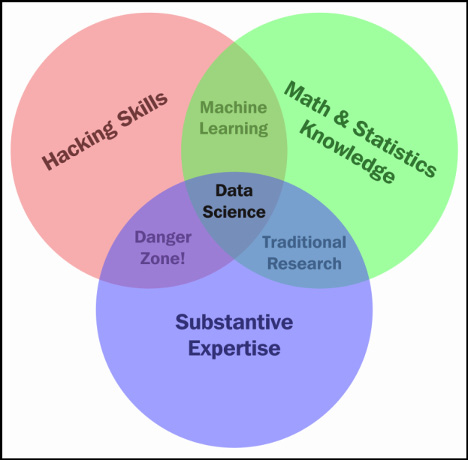
Figure 1.2 – The Venn diagram of data science
Those with hacking skills can conceptualize and program complicated algorithms using computer languages. Having a math and statistics background allows you to theorize and evaluate algorithms and tweak the existing procedures to fit specific situations. Having substantive expertise (domain expertise) allows you to apply concepts and results in a meaningful and effective way.
While having only two of these three qualities can make you intelligent, it will also leave a gap. Let’s say that you are very skilled in coding and have formal training in day trading. You might create an automated system to trade in your place but lack the math skills to evaluate your algorithms. This will mean that you end up losing money in the long run. It is only when you boost your skills in coding, math, and domain knowledge that you can truly perform data science.
The quality that was probably a surprise for you was domain knowledge. It is just knowledge of the area you are working in. If a financial analyst started analyzing data about heart attacks, they might need the help of a cardiologist to make sense of a lot of the numbers.
Data science is the intersection of the three key areas mentioned earlier. To gain knowledge from data, we must be able to utilize computer programming to access the data, understand the mathematics behind the models we derive, and, above all, understand our analyses’ place in the domain we are in. This includes the presentation of data. If we are creating a model to predict heart attacks in patients, is it better to create a PDF of information or an app where we can type in numbers and get a quick prediction? All these decisions must be made by the data scientist.
The intersection of math and coding is machine learning. This book will look at machine learning in great detail later on, but it is important to note that without the explicit ability to generalize any models or results to a domain, machine learning algorithms remain just that – algorithms sitting on your computer. You might have the best algorithm to predict cancer. You could be able to predict cancer with over 99% accuracy based on past cancer patient data, but if you don’t understand how to apply this model in a practical sense so that doctors and nurses can easily use it, your model might be useless.
Both computer programming and math will be covered extensively in this book. Domain knowledge comes with both the practice of data science and reading examples of other people’s analyses.
The math
Most people stop listening once someone says the word “math.” They’ll nod along in an attempt to hide their utter disdain for the topic but hear me out. As an experienced math teacher, I promise that this book will guide you through the math needed for data science, specifically statistics and probability. We will use these subdomains of mathematics to create what are called models. A data model refers to an organized and formal relationship between elements of data, usually meant to simulate a real-world phenomenon.
The central idea of using math is that we will use it to formalize relationships between variables. As a former pure mathematician and current math teacher, I know how difficult this can be. I will do my best to explain everything as clearly as I can. Of the three areas of data science, math is what allows us to move from domain to domain. Understanding the theory allows us to apply a model that we built for the fashion industry to a financial domain.
The math covered in this book ranges from basic algebra to advanced probabilistic and statistical modeling. Do not skip over these chapters, even if you already know these topics or you’re afraid of them. Every mathematical concept that I will introduce will be introduced with care and purpose, using examples. The math in this book is essential for data scientists.
There are many types of data models, including probabilistic and statistical models. Both of these are subsets of a larger paradigm, called machine learning. The essential idea behind these three topics is that we use data to come up with the best model possible. We no longer rely on human instincts – rather, we rely on data. Math and coding are vehicles that allow data scientists to step back and apply their skills virtually anywhere.
Computer programming
Let’s be honest: you probably think computer science is way cooler than mathematics. That’s OK, I don’t blame you. The news isn’t filled with math news like it is with news on technology (although I think that’s a shame). You don’t turn on the TV to see a new theory on primes or about Euler’s equation – rather, you see investigative reports on how the latest smartphone can take better photos of cats or how generative AI models such as ChatGPT can learn to create websites from scratch. Computer languages are how we communicate with machines and tell them to do our bidding. A computer speaks many languages and, like a book, can be written in many languages; similarly, data science can also be done in many languages. Python, Julia, and R are some of the many languages that are available to us. This book will exclusively use Python.
Why Python?
We will use Python for a variety of reasons, listed as follows:
- Python is an extremely simple language to read and write, even if you’ve never coded before, which will make future examples easy to understand and read later on, even after you have read this book.
- It is one of the most common languages, both in production and in an academic setting (one of the fastest growing, as a matter of fact).
- The language’s online community is vast and friendly. This means that a quick search for the solution to a problem should yield many people who have faced and solved similar (if not the same) situations.
- Python has prebuilt data science modules that both novice and veteran data scientists can utilize.
The last point is probably the biggest reason we will focus on Python. These pre built modules are not only powerful but also easy to pick up. By the end of the first few chapters, you will be very comfortable with these modules. Some of these modules include the following:
- pandas
- PyTorch
- Scikit-learn
- Seaborn
- NumPy/scipy
- Requests (to mine data from the web)
- BeautifulSoup (for web-HTML parsing)
We will assume that you have basic Python coding skills. This includes the ability to recognize and use the basic types (int
, float
, boolean
, and so on) and create functions and classes with ease. We will also assume that you have mastery over logistical operators, including ==
, >=
, and <=
.
Example – parsing a single tweet
Here is some Python code that should be readable to you. In this example, I will be parsing some tweets about stock prices. If you can follow along with this example easily, then you are ready to proceed!
tweet = "RT @j_o_n_dnger: $TWTR now top holding for Andor, unseating $AAPL" words_in_tweet = tweet.split(' ') # list of words in tweet for word in words_in_tweet: # for each word in list if "$" in word: # if word has a "cashtag" print("THIS TWEET IS ABOUT", word) # alert the user
I will point out a few things about this code snippet line by line, as follows:
- First, we set a variable to hold some text (known as a string in Python). In this example, the tweet in question is
RT @robdv: $TWTR now top holding for Andor,
unseating $AAPL
. - The
words_in_tweet
variable tokenizes the tweet (separates it by word). If you were to print this variable, you would see the following:['RT', '@robdv:', '$TWTR', 'now', 'top', 'holding', 'for', 'Andor,', 'unseating', '$AAPL']
- We iterate through this list of words using a
for
loop, going through the list one by one. - Here, we have another
if
statement. For each word in this tweet, if the word contains the$
character, this represents stock tickers on Twitter.if "$" in word: # if word has a "cashtag"
- If the preceding
if
statement isTrue
(that is, if the tweet contains a cashtag), print it and show it to the user.The output of this code will be as follows:
THIS TWEET IS ABOUT $TWTR
THIS TWEET IS ABOUT $AAPL
Whenever I use Python in this book, I will ensure that I am as explicit as possible about what I am doing in each line of code. I know what it’s like to feel lost in code. I know what it’s like to see someone coding and want them to just slow down and explain what is going on.
As someone who is entirely self-taught in Python and has also taught Python at the highest levels, I promise you that I will do everything in my power to not let you feel that way when reading this book.
Domain knowledge
As I mentioned earlier, domain knowledge focuses mainly on knowing the particular topic you are working on. For example, if you are a financial analyst working on stock market data, you have a lot of domain knowledge. If you are a journalist looking at worldwide adoption rates, you might benefit from consulting an expert in the field. This book will attempt to show examples from several problem domains, including medicine, marketing, finance, and even UFO sightings!
Does this mean that if you’re not a doctor, you can’t work with medical data? Of course not! Great data scientists can apply their skills to any area, even if they aren’t fluent in it. Data scientists can adapt to the field and contribute meaningfully when their analysis is complete.
A big part of domain knowledge is presentation. Depending on your audience, it can matter greatly how you present your findings. Your results are only as good as your vehicle of communication. You can predict the movement of the market with 99.99% accuracy, but if your program is impossible to execute, your results will go unused. Likewise, if your vehicle is inappropriate for the field, your results will go equally unused. I know I’m throwing a lot at you already, but we should look at just a few more relevant terminologies so that we can hit the ground running.