As we just saw, a basic value iteration approach can be used to update the Bellman equation and iteratively find ideal state-action pairs to optimally navigate a given environment. This approach actually stores new information at each time step, iteratively making our algorithm more intelligent. However, there is a problem with this method as well. It's simply not scalable! The taxi cab environment is simple enough, with 500 states and 6 actions, to be solved by iteratively updating the Q-values, thereby estimating the value of each individual state-action pair. However, more complex simulations, like a video game, may potentially have millions of states and hundreds of actions, which is why computing the quality of each state-action pair becomes computationally unfeasible and logically inefficient. The only option we are left with, in such circumstances...
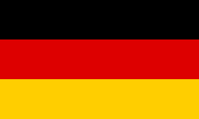
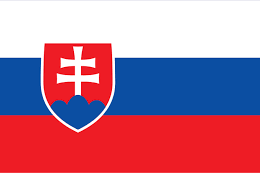
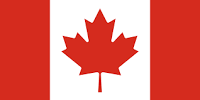
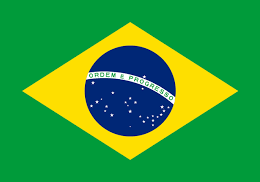

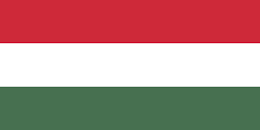
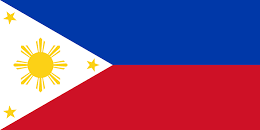
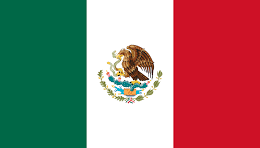
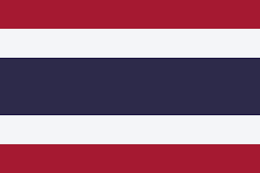
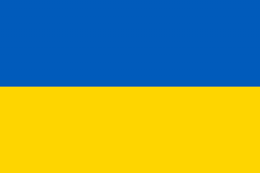
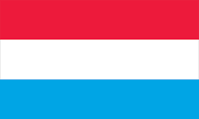
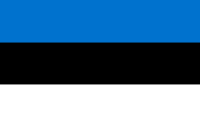
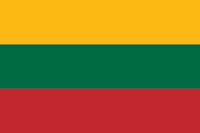
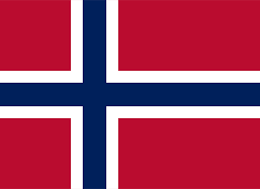
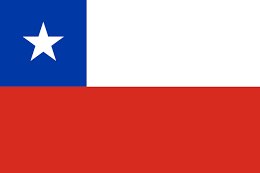
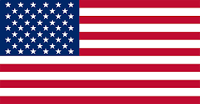
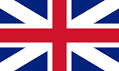
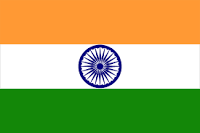
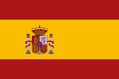
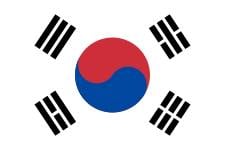
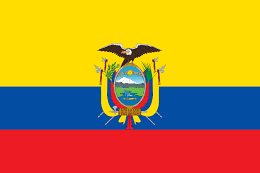
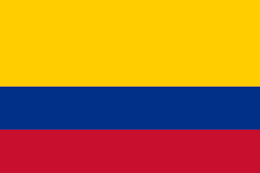
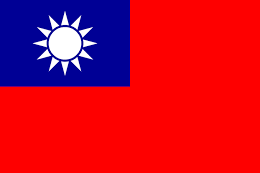
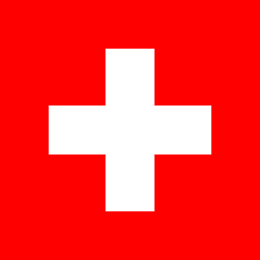
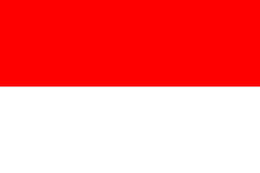
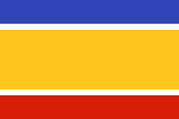
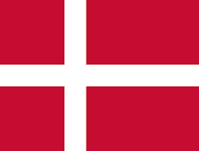
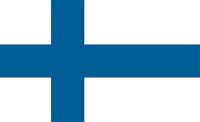
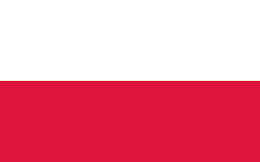
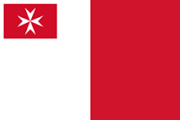
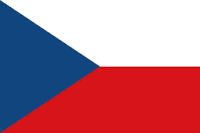
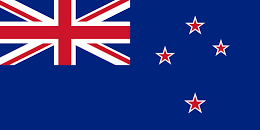
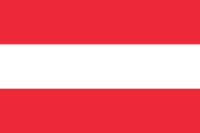
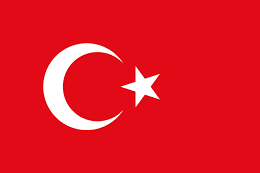
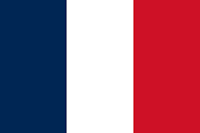
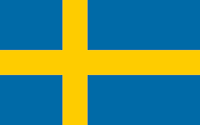
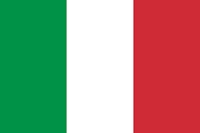
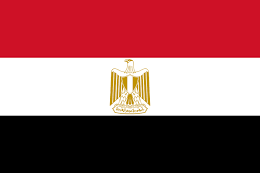
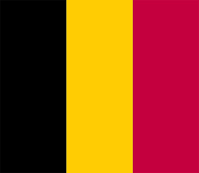
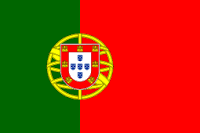
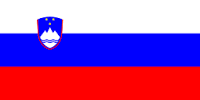
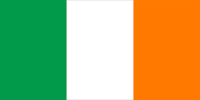
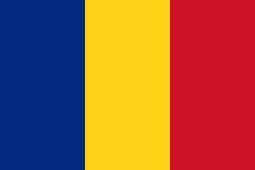
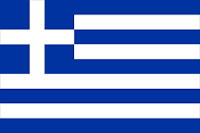
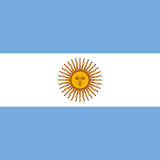
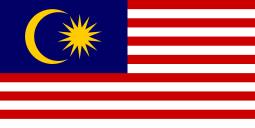
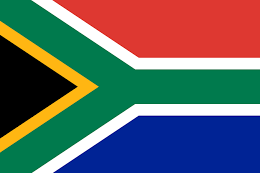
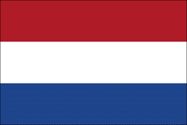
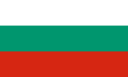
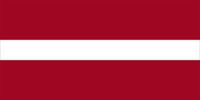
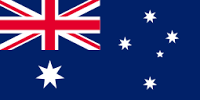
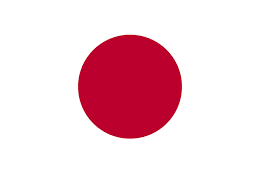
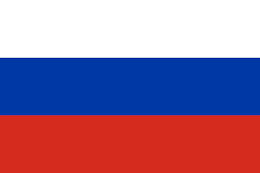