How computation can help answer different questions in life sciences
It is generally believed that biostatistics is mostly about numbers and graphs. The reality is quite different. Biostatistics is also about understanding life science problems and finding ways to resolve those using statistical methods. There are six main problem-solving skills in biostatistics:
- Helping life science professionals resolve research problems in these domains through the use of data
- Helping life science professionals interpret the results of their research
- Making sure the published research is both statistically and biologically valid
- Helping R&D professionals make decisions in the projects
- Revealing objective truths about different phenomena through the use of data
- Explaining the abstract features of mathematics and biology in an intuitive and easy-to-understand way
One of the most important impacts of biostatistics is transitioning from statistical knowledge to actual problem solutions in life sciences. This will be discussed in more detail in the rest of this chapter.
Biostatistics is needed to derive insights from life science experiments and convert measurements and observations to life science solutions.
Professionals in life science and biostatisticians, working together, design different types of experiments, measurements, and observations. All these can be written or stored as data. Data is a source of information from those experiments, measurements, and observations.
Data can originate from observations, too. One example of observation is the diagnosis by a dermatologist or the identification of species by biologists.
Biostatisticians are there to help make sure this data is valid and make it meaningful. Further, data should be organized and structured, often presented in the form of tables to be prepared for further analysis and interpretation.
To make the data useful, we must understand all the details about the data and how these are related to domains where biostatistics is applied. One of the most important aspects of biostatistics is the context around the data. This context can significantly affect the results and is one of the reasons why biostatisticians are more specialized in life science domains than general statisticians.
One of the main goals of biostatistics is to take all available inputs in the form of data and process them in such a way as to produce meaningful insights, answers, and conclusions and provide information to make decisions in life science.
Here is the biostatistics workflow:
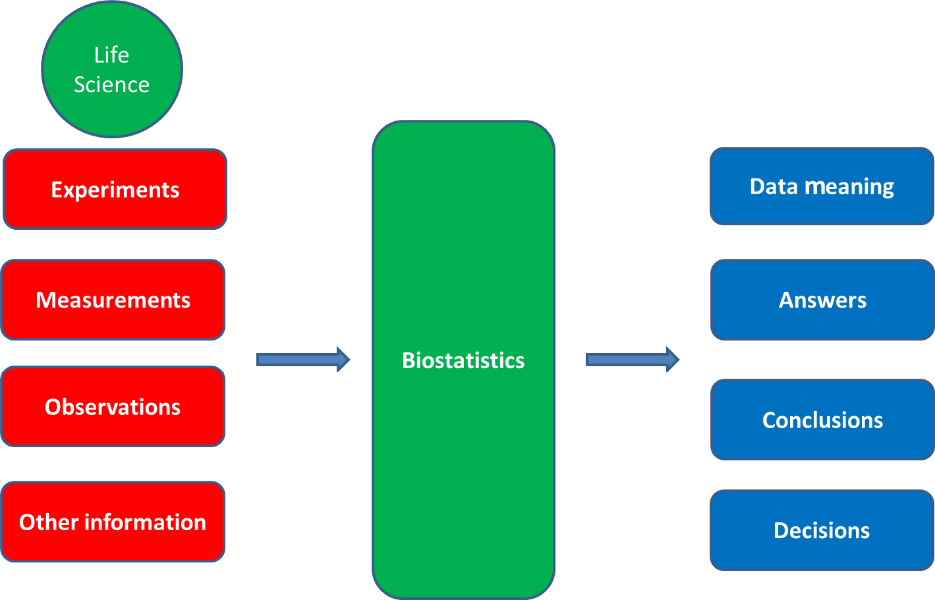
Figure 1.3 – Biostatistics workflow
There are two main types of data: numerical (for example, the measurement of the hemoglobin level in blood in which we are using numerical values such as grams per liter or g/L) and categorical, such as a doctor’s diagnoses of their patients in a form; “Yes” for a positive diagnosis or “No” for a negative diagnosis. These types of data can be further divided into subcategories, which will be discussed in detail in the next chapters.
Understanding data sources is essential for biostatistics. Biostatistics is focused on statistical models but also on domain knowledge and, as such, has evolved as a separate branch of both statistics and life sciences.
This book will provide many different examples that will show you how to use biostatistics specifically for different domains, such as diabetes research, cardiology, and biostatistical studies. Further, in this chapter, we will discuss how the Python programming language can facilitate the implementation of biostatistical methods.
Biostatistics and Python
Most biostatistical analyses today are implemented in some form of software or a programming language. I chose Python as a programming language for this book for several reasons. Python is one of the most advanced languages for data science and biostatistics. As programmers today are moving toward using Python, keep in mind that it is one of the most wanted skills in most areas that have to do with analytics. Libraries such as Biopython and SciPy are among the more than 100,000 libraries that make Python so versatile, meaning that almost any biostatistical analysis can be performed using this programming language. It is open source, meaning it is transparent and free for anyone to use.
The following figure is an example of using Python for biostatistics:
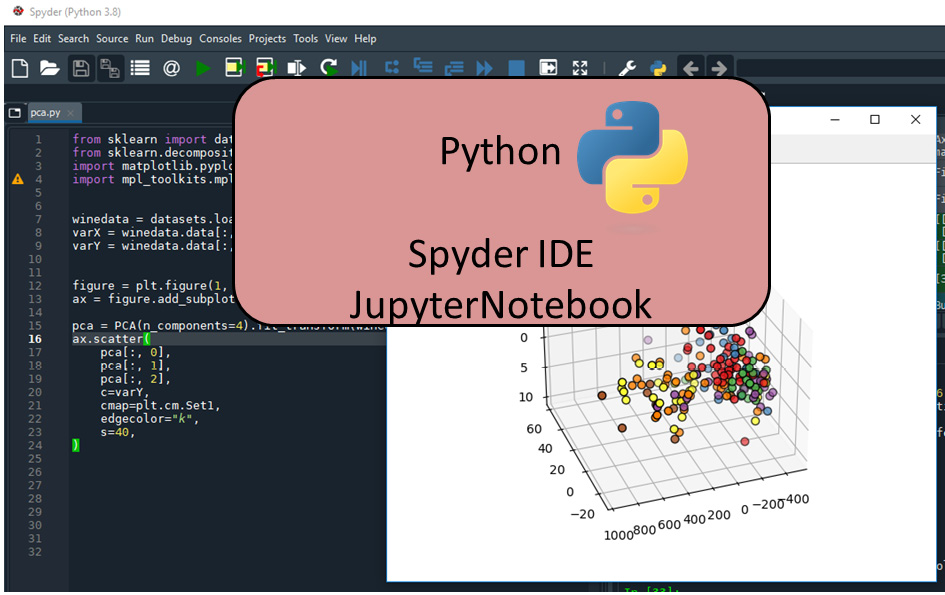
Figure 1.4 – Biostatistics and Python
Its integration with advanced machine learning and bioinformatics algorithms gives a biostatistician a whole new spectrum of approaches and provides the most advanced frameworks for using biostatistical algorithms at this time.
Finally, the most important part – learning Python through a portfolio of practical projects provides you, as a reader, with two important qualities: being able to use one of the most wanted programming languages out there can be beneficial for your career, and having a portfolio of more than 10 practical projects using biostatistics and Python provides significant resources for your portfolio as someone who plans to use or advance your career by using biostatistics.