Let's use an example to understand neural networks in more detail:
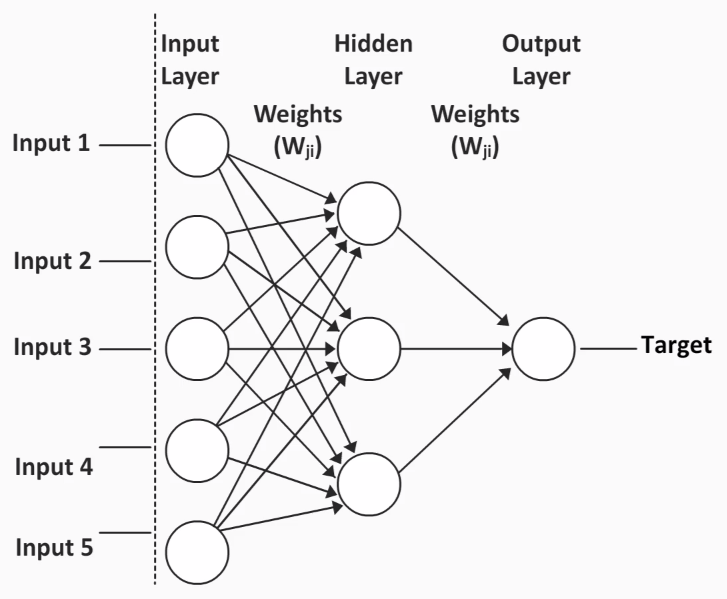
Notice that every neuron in the Input Layer is connected to every neuron in the Hidden Layer, for example, Input 1 is connected to the first, second, and even the third neuron in the Hidden Layer. This implies that there will be three different weights, and these weights will be a part of three different equations.
This is what happens in this example:
- The Hidden Layer intervenes between the Input Layer and the Output Layer.
- The Hidden Layer allows for more complex models with nonlinear relationships.
- There are many equations, so the influence of a single predictor on the outcome variable occurs through a variety of paths.
- The interpretation of weights won't be straightforward.
- Weights correspond to the variable importance; they will initially be random, and then they will go through a bunch of different iterations and will be changed based on the feedback of the iterations. They will then have their real meaning of being associated with variable importance.
So, let's go ahead and see how these weights are determined and how we can form a functional neural network.