Feature tour of model management and deployment capabilities
In this section, we'll dive into SageMaker's model hosting and monitoring capabilities. By the end of this section, you should understand the basics of SageMaker model endpoints along with the use of SageMaker Model Monitor. You'll also learn about deploying models on edge devices with SageMaker Edge Manager.
Model Monitor
In some organizations, the gap between the ML team and the operations team causes real problems. Operations teams may not understand how to monitor an ML system in production, and ML teams don't always have deep operational expertise.
Model Monitor tries to solve that problem: it will instrument a model endpoint and collect data about the inputs to, and outputs from, an ML model used for inference. It can then analyze that data for data drift and other quality problems, as well as model accuracy or quality problems. The following diagram shows an example of model monitoring data captured for an inference endpoint:
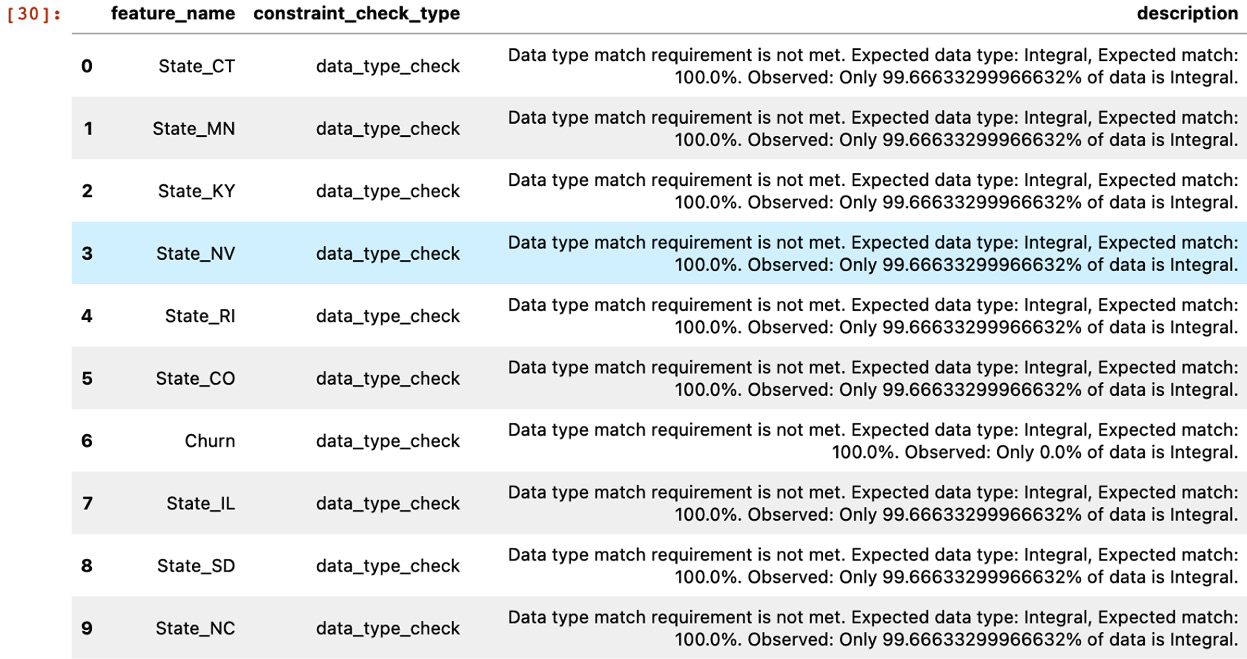
Figure 1.17 – Model Monitor checking data quality on inference inputs
Model endpoints
In some cases, you need to get a large number of inferences at once, in which case SageMaker provides a batch inference capability. But if you need to get inferences closer to real time, you can host your model in a SageMaker managed endpoint. SageMaker handles the deployment and scaling of your endpoints. Just as important, SageMaker lets you host multiple models in a single endpoint. That's useful both for A/B testing (that is, you can direct some percentage of traffic to a newer model) and for hosting multiple models that are tuned for different traffic segments.
You can also host an inference pipeline with multiple containers chained together, which is convenient if you need to preprocess inputs before performing inference. The following screenshot shows a model endpoint with two models serving different percentages of traffic:
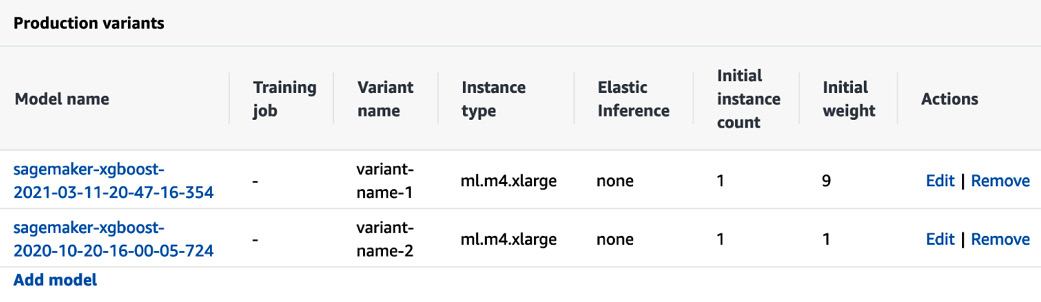
Figure 1.18 – Multiple models configured behind a single inference endpoint
Edge Manager
In some cases, you need to get model inferences on a device rather than from the cloud. You may need a lower response time that doesn't allow for an API call to the cloud, or you may have intermittent network connectivity. In video use cases, it's not always feasible to stream data to the cloud for inference. In such cases, Edge Manager and related tools such as SageMaker Neo help you compile models optimized to run on devices, deploy them, manage them, and get operational metrics back to the cloud. The following screenshot shows an example of a virtual device managed by Edge Manager:
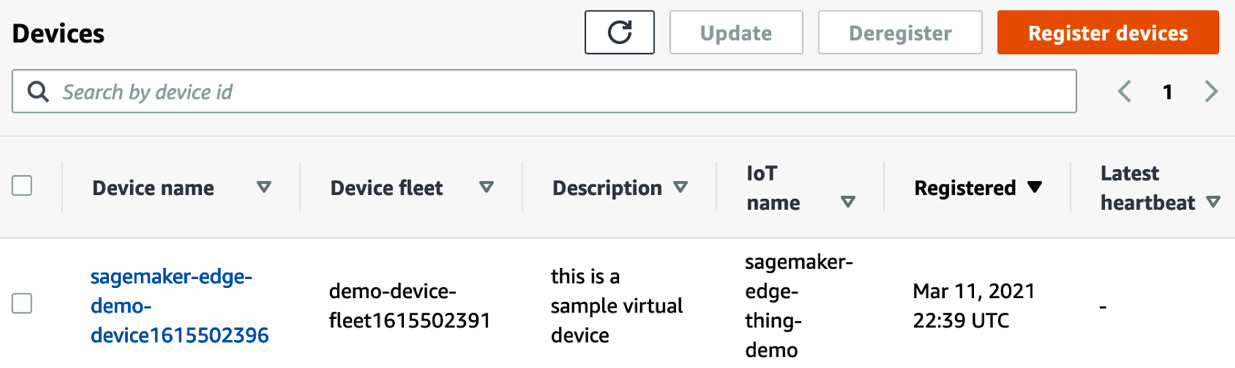
Figure 1.19 – A device registered to an Edge Manager device fleet
Before we conclude with the summary, let's have a recap of the SageMaker capabilities provided for the following primary ML phases:
- For data preparation:
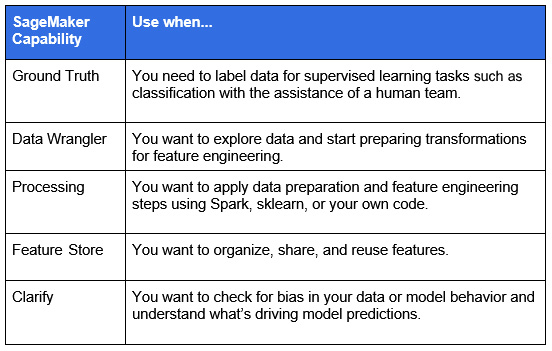
Figure 1.20 – SageMaker capabilities for data preparation
- For operations:
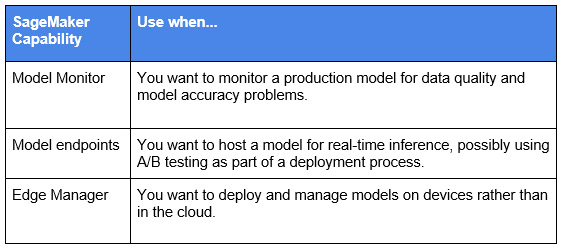
Figure 1.21 – SageMaker capabilities for operations
- For model training:
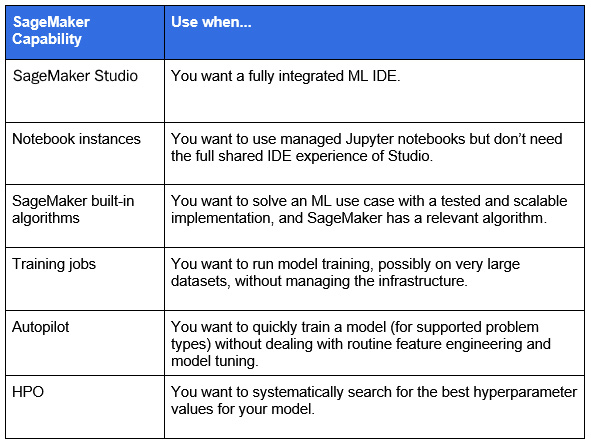
Figure 1.22 – SageMaker capabilities for model training
With this, we have come to the end of this chapter.