In this chapter, we took a diversion and built our own DRL environments for training with our own code, or another framework, or using the ML-Agents framework from Unity. At first, we looked at the basics of installing the ML-Agents toolkit for the development of environments, training, and training with our own code. Then, we looked at how to build a basic Unity environment for training from a Gym interface like we have been doing throughout this whole book. After that, we learned how our RainbowDQN sample could be customized to train an agent. From there, we looked at how we can create a brand new environment from the basics. We finished this chapter by looking at managing rewards in environments and the set of tools ML-Agents uses to enhance environments with sparse rewards. There, we looked at several methods Unity has added to ML-Agents to assist with difficult environments...
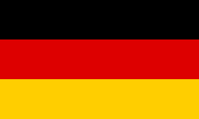
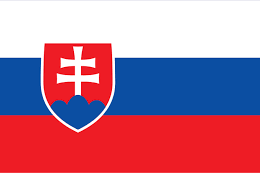
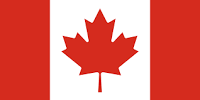
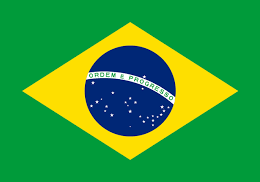

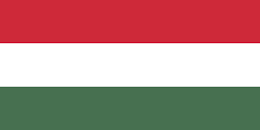
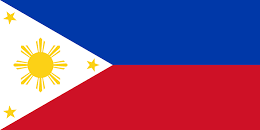
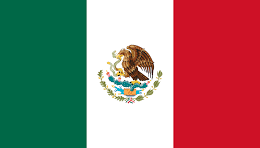
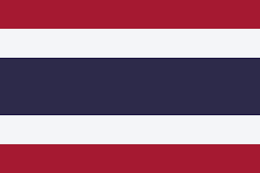
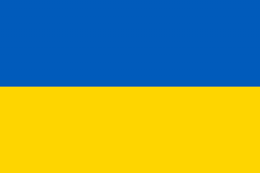
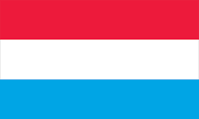
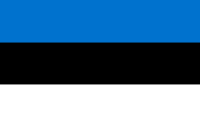
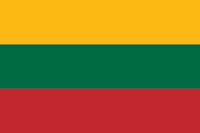
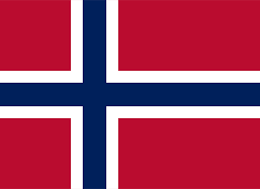
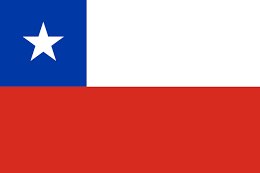
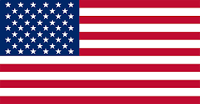
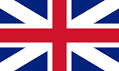
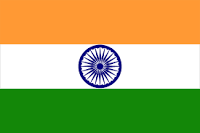
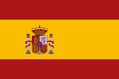
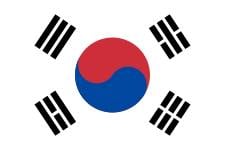
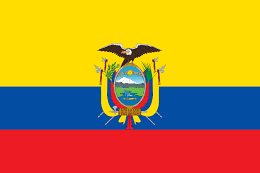
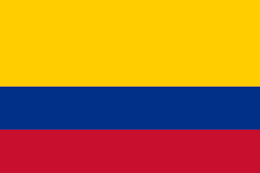
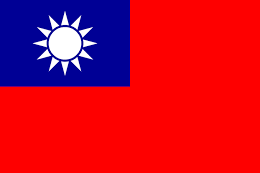
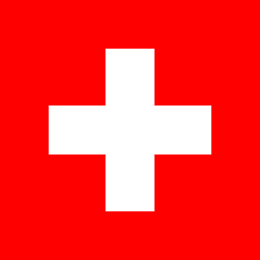
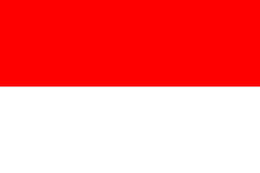
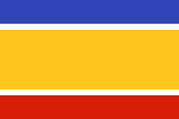
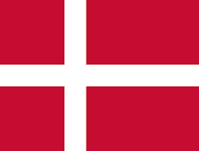
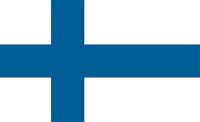
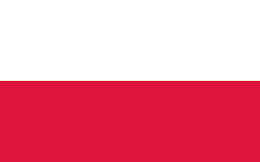
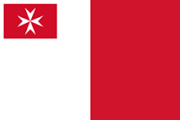
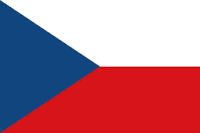
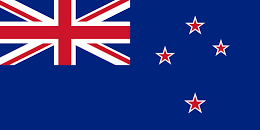
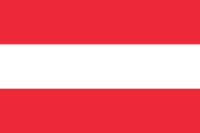
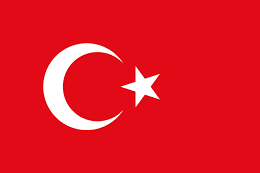
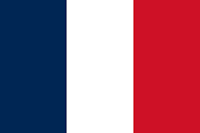
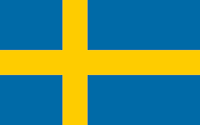
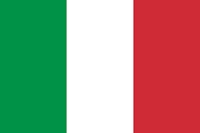
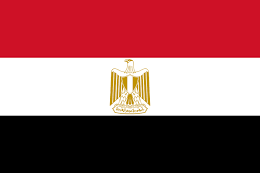
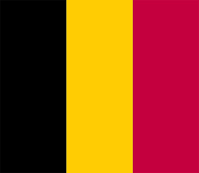
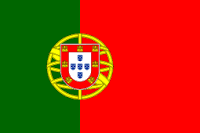
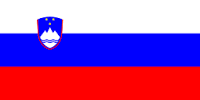
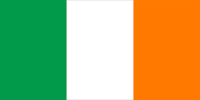
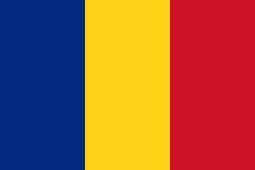
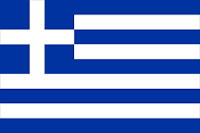
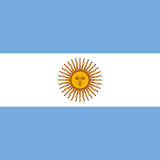
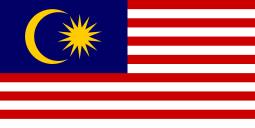
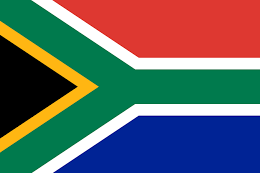
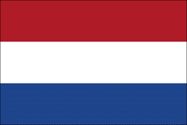
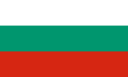
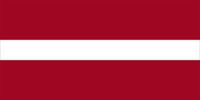
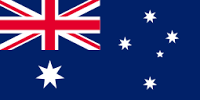
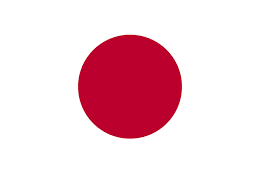
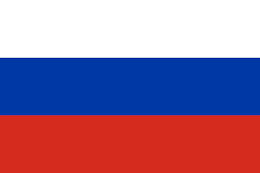