Classification and regression decision tree models
Classification and regression trees (CART) are a type of supervised learning algorithm that can be used both for classification and regression problems.
In a classification problem, the goal is to predict the class, label, or category of a data point or an object. One example of a classification problem is to predict whether there will be customer churn or if a customer will purchase a product based on historical data.
In a regression problem, the goal is to predict a continuous numerical value, such as the price of a house based on the input features. For example, a regression CART model could be used to predict the price of a house based on input features, such as its size, location, and other relevant features.
CART models are built by recursively splitting the data into subsets based on the value of a feature that best separates the data. The algorithm chooses the feature that maximizes the separation of the classes or minimizes the variance of the target variable. The splitting process is repeated until the data are no longer able to be split further.
This process creates a tree-like structure where each internal node represents a feature or attribute, and each leaf node represents a predicted class label or a predicted continuous value. The tree can then be used to predict the class label or continuous value for new data points by following the path down the tree based on their features.
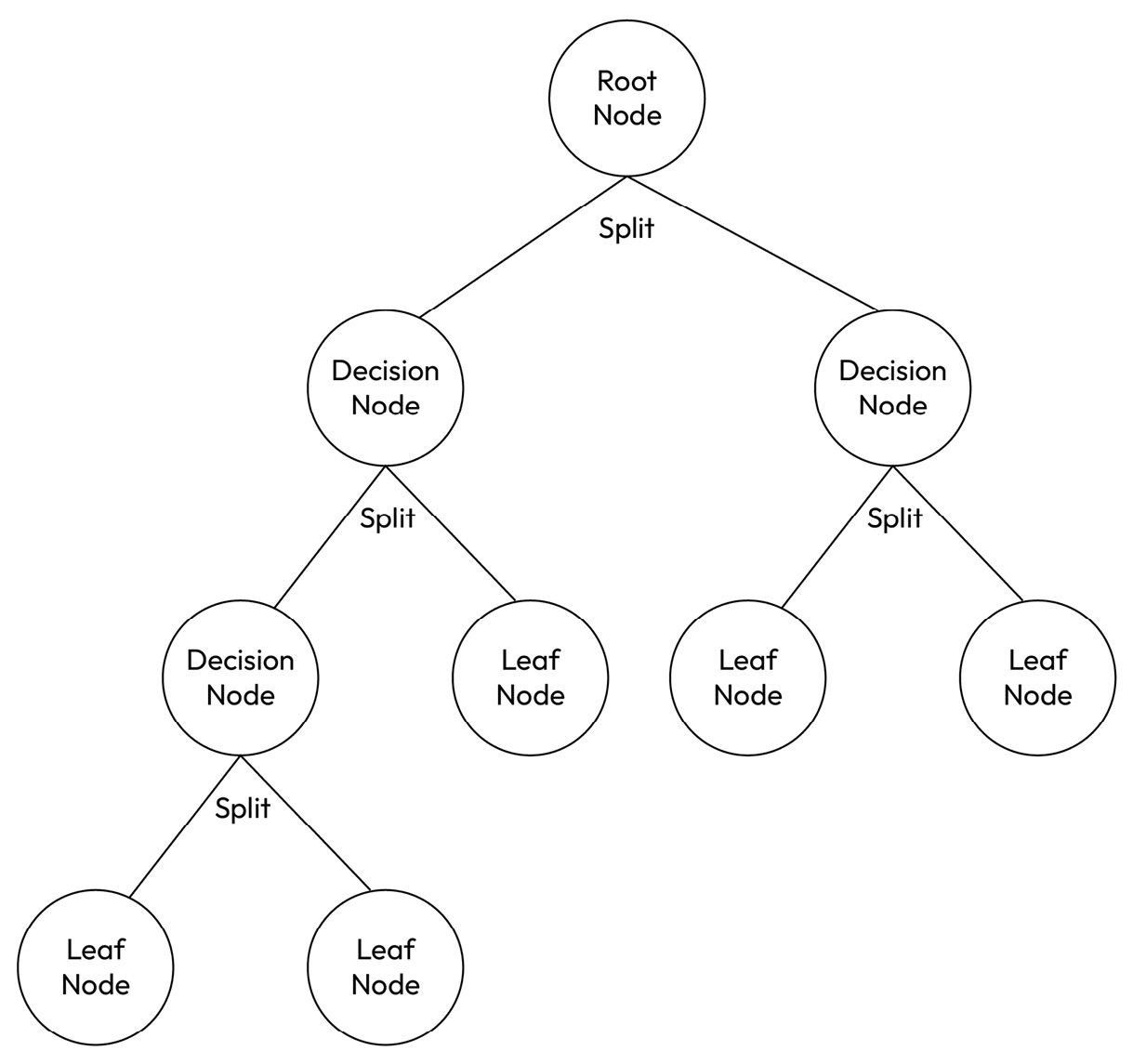
Figure 1.1 – A sample classification and regression tree
CART models are easy to explain and can handle both categorical and numerical features. However, they can be prone to overfitting. Overfitting is a phenomenon in machine learning where a model performs extremely well on the training data but fails to generalize well to unseen data. Regularization techniques such as pruning can be used to prevent overfitting. Pruning in machine learning refers to the technique of selectively removing unnecessary or less important features from a model to improve its efficiency, reduce its complexity, and prevent overfitting. The following table summarizes the advantages and disadvantages of CART models:
Advantages of CART models |
Disadvantages of CART models |
Easy to understand and interpret |
Prone to overfitting |
Relatively fast to train |
Sensitive to noise in the data |
Can be used for both classification and regression problems |
Can be computationally expensive to train, especially for large datasets, because they need to search through all possible splits in the data in order to find the optimal tree structure |
Table 1.1 – Advantages and disadvantages of CART models
As seen in the preceding table, overall, CART models are a powerful supervised learning-based tool that can be used for a variety of machine learning tasks. However, they have limitations, and we must take steps to prevent overfitting.