As we can see, the efficiency of our agent to solve an environment depends on what policy it uses to match state-action pairs at each time step. Hence, a function, known as a policy function (Ï€), can specify the combination of state-action pairs for each time step the agent comes across. As the simulation runs, the policy is responsible for producing the trajectory, which is composed of game-states; actions that are taken by our agent as a response; and a reward that's generated by the environment, as well as the next state of the game the agent receives. Intuitively, you can think of a policy as a heuristic that generates actions that respond to the generated states of an environment. A policy function itself can be a good or a bad one. If your policy is to shoot first and ask questions later, you may end up shooting a hostage. Hence...
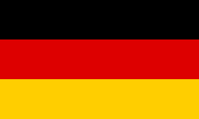
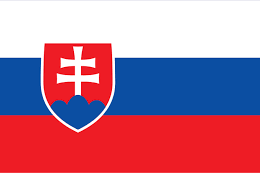
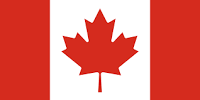
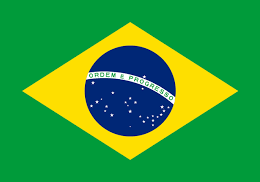

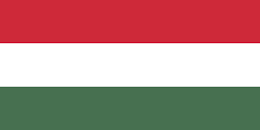
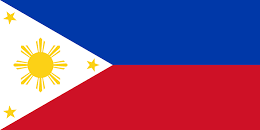
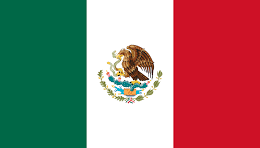
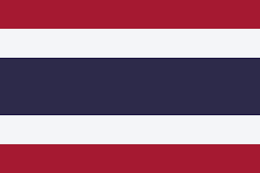
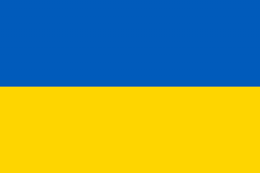
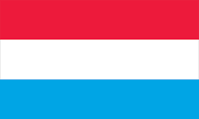
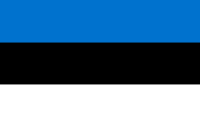
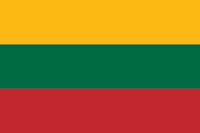
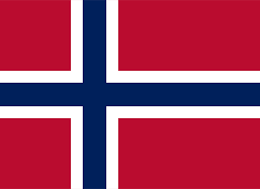
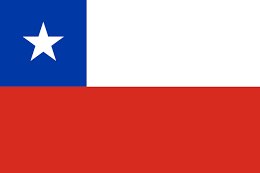
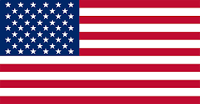
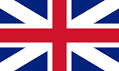
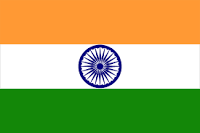
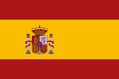
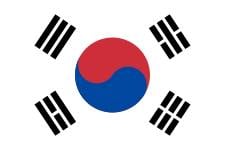
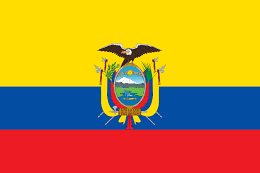
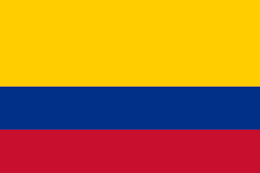
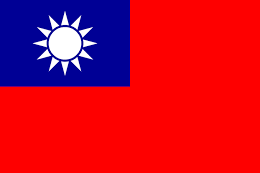
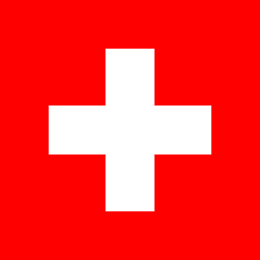
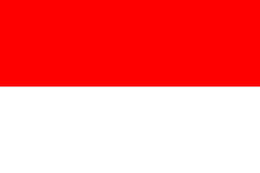
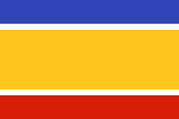
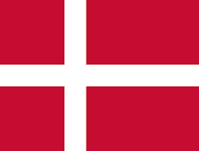
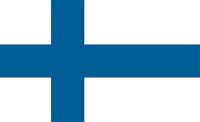
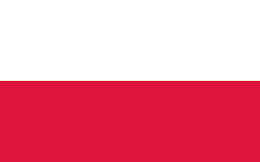
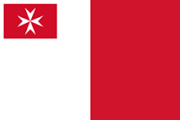
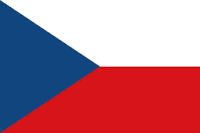
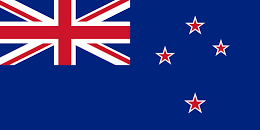
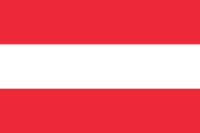
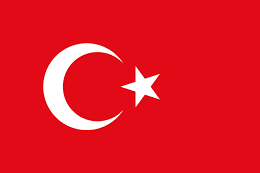
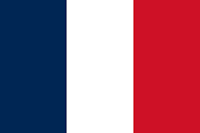
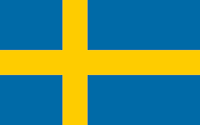
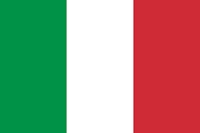
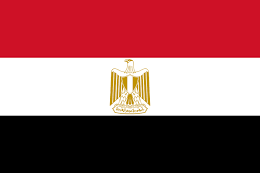
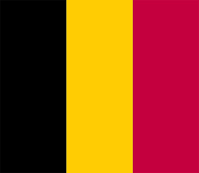
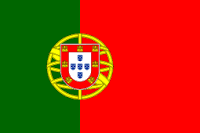
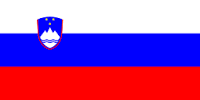
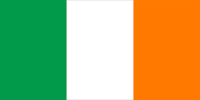
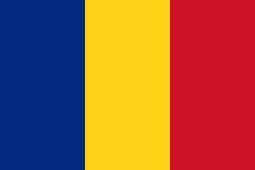
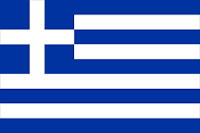
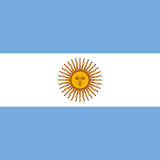
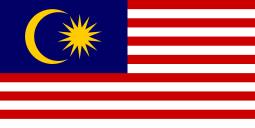
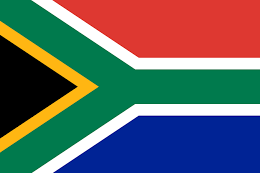
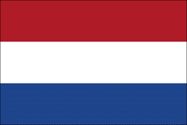
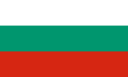
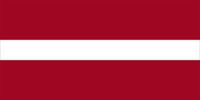
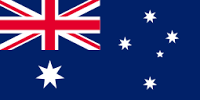
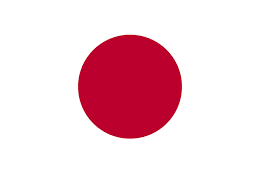
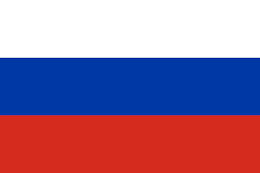