One of the key challenges in classifying images is the availability of large training datasets. For example, to create Amazon Go-type experiences, the e-commerce retailer may have trained their machine learning algorithms on large volumes of images. When we don't have images covering all types of real-world scenarios – scenarios ranging from time of the day (brightness), ambience around the target item, and item angle – we're unable to train image classification algorithms that are able to perform well in real-life environments. Furthermore, it takes a lot of effort to build a convolutional neural network architecture that is optimal for the dataset at hand. These considerations range from the number of convolutional layers to the batch size, to the optimizer, and to dropout rates. It takes...
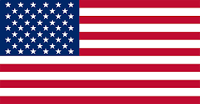
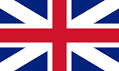
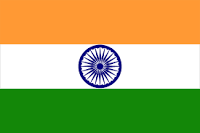
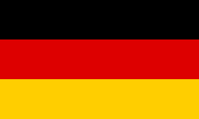
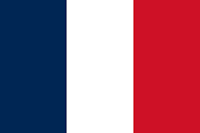
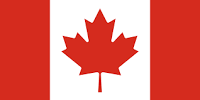
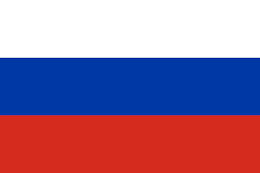
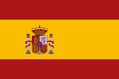
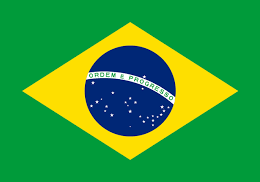
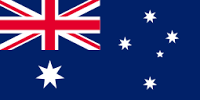

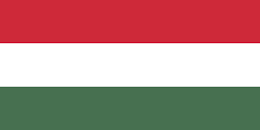
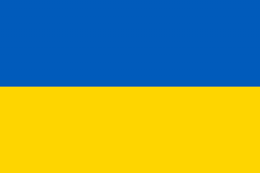
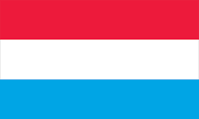
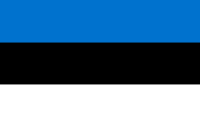
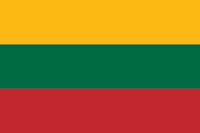
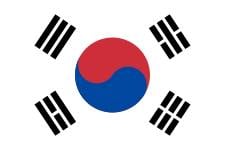
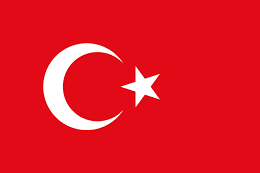
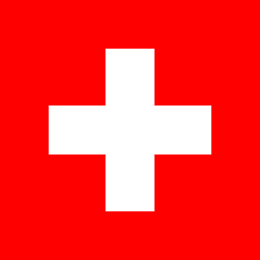
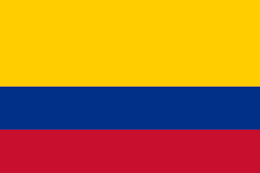
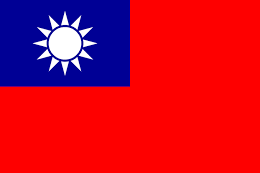
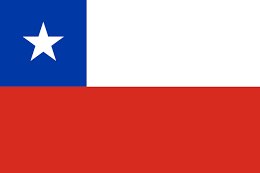
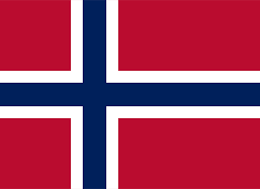
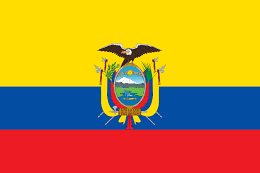
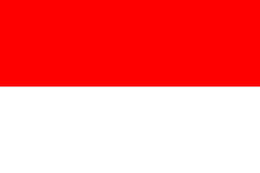
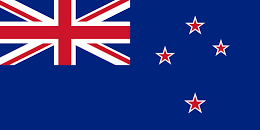
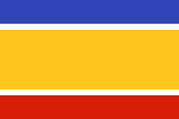
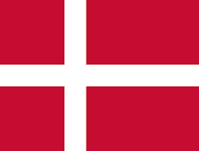
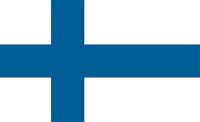
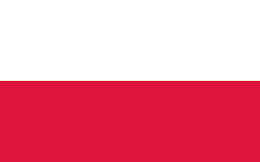
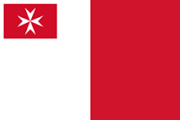
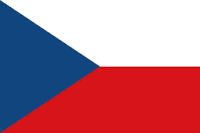
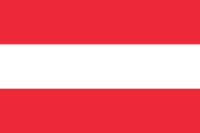
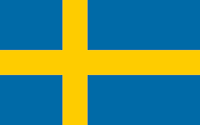
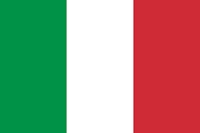
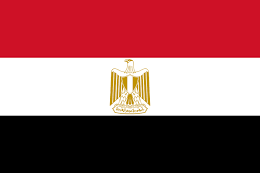
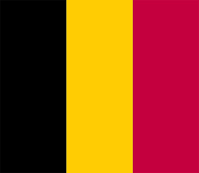
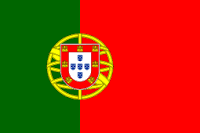
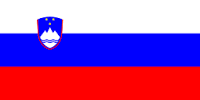
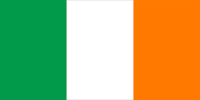
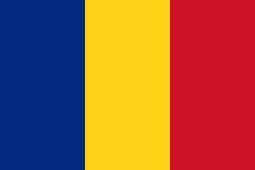
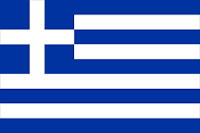
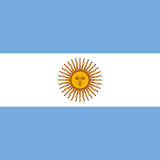
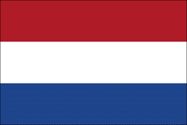
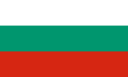
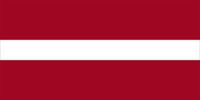
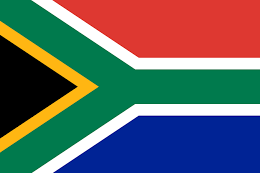
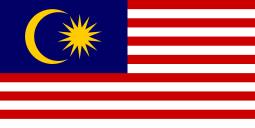
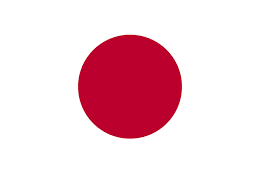
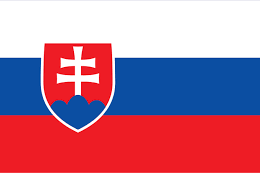
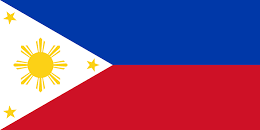
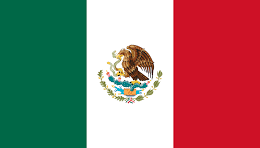
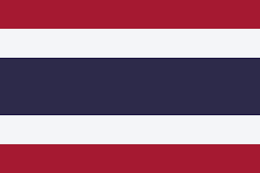