For this purpose, we use the visualize_cam function, which essentially generates a Grad-CAM that maximizes the layer activations for a given input, for a specified output class.
The visualize_cam function takes the same four arguments we saw earlier, plus an additional one. We pass it the arguments corresponding to a Keras model, a seed input image, a filter index corresponding to our output class (ImageNet index for leopard), as well as two model layers. One of these layers remains the fully connected dense output player, whereas the other layer refers to the final convolutional layer in the ResNet50 model. The method essentially leverages these two reference points to generate the gradient weighted class activation maps, as shown:
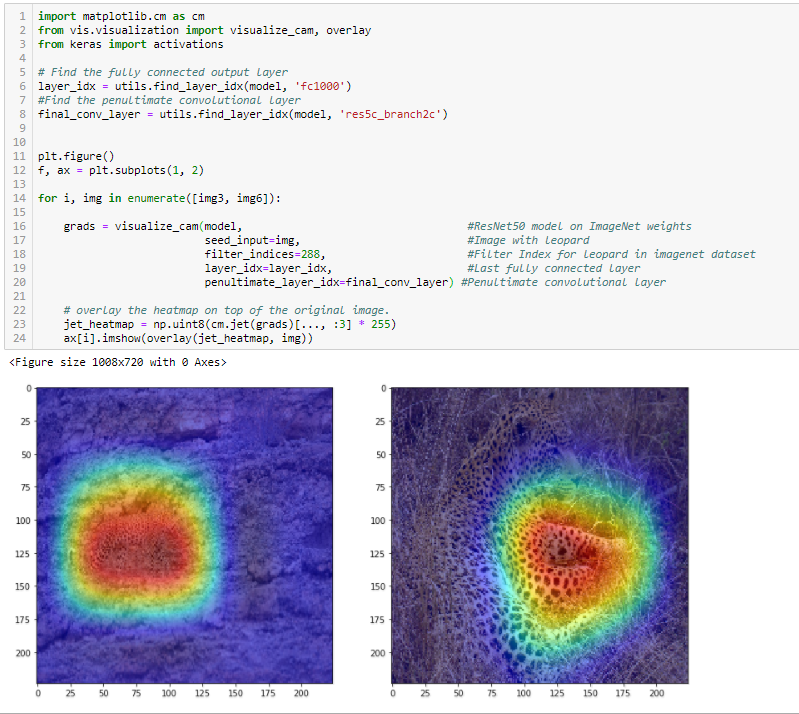
As we see, the network correctly identifies the leopards in both images. Moreover, we notice that the...