In all previous chapters, we have always supposed that our datasets were drawn from an implicit data-generating process pdata and all the algorithms assumed xi ∈ X as independent and identically distributed (IID) and uniformly sampled. We were supposing that X represented pdata with enough accuracy so that an algorithm could learn to generalize with limited initial knowledge. In this chapter, instead, we are interested in directly modeling pdata without any specific restriction (for example, a Gaussian mixture model achieves this goal by imposing a constraint on the structure of the distributions). Before discussing some very powerful approaches, it's helpful to briefly recap the properties of a generic continuous probability density function p(x) defined on a measurable subset X ℜn (to avoid confusion, we are going to indicate...
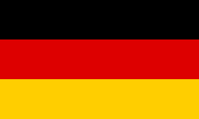
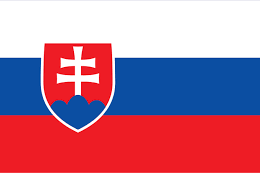
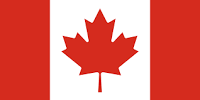
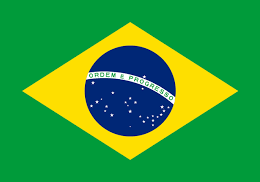

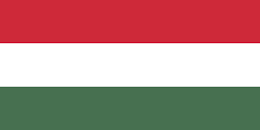
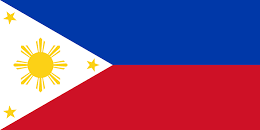
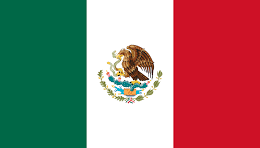
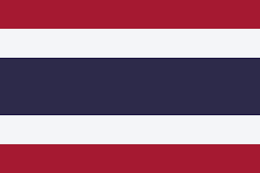
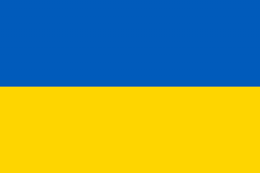
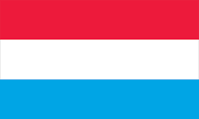
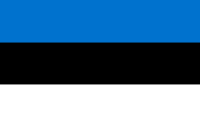
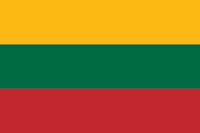
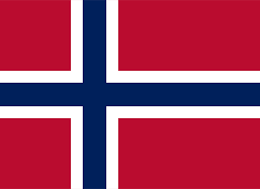
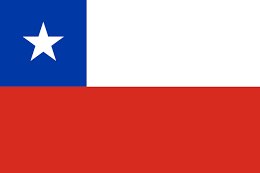
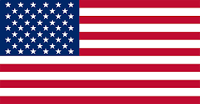
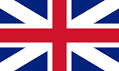
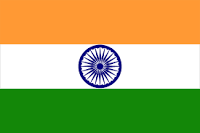
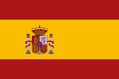
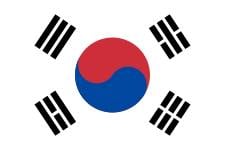
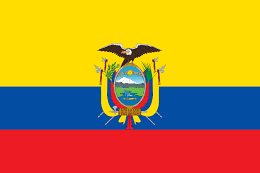
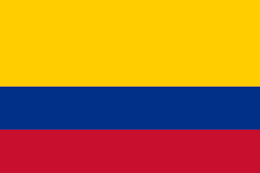
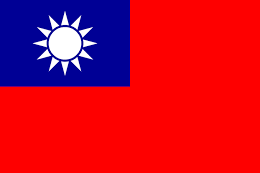
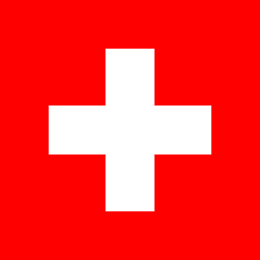
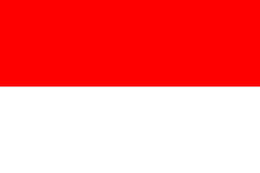
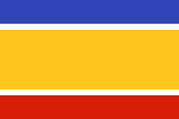
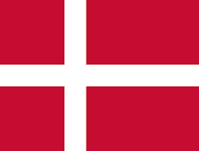
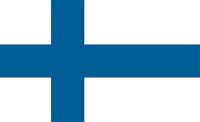
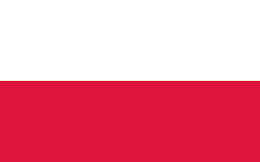
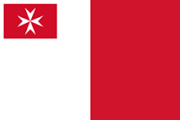
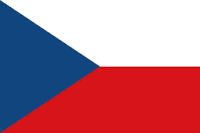
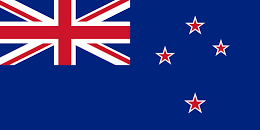
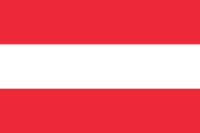
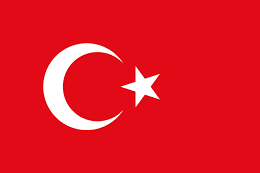
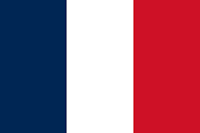
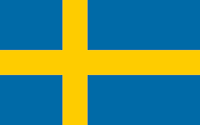
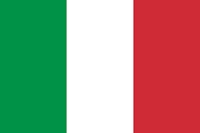
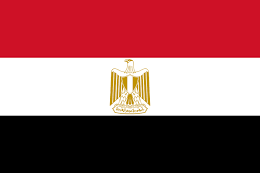
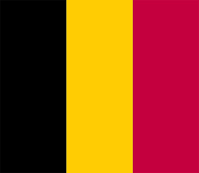
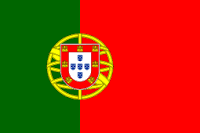
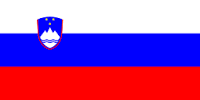
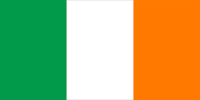
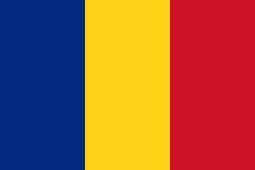
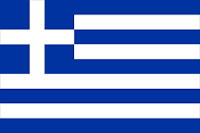
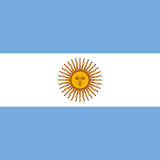
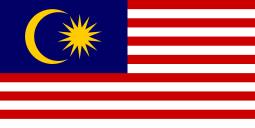
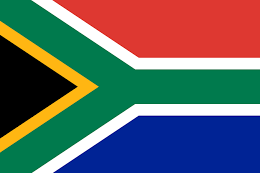
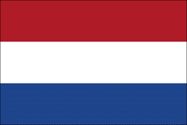
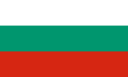
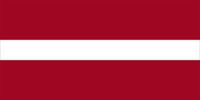
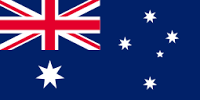
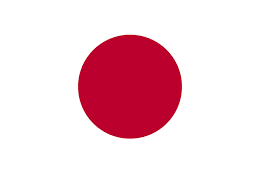
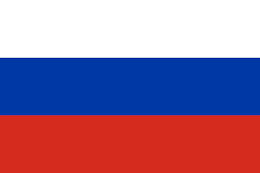