In Chapter 7, Dimensionality Reduction And Component Analysis, we discussed some common methods that can be employed to reduce the dimensionality of a dataset, given its peculiar statistical properties (for example, the covariance matrix). However, when complexity increases, even kernel principal component analysis (kernel PCA) might be unable to find a suitable lower-dimensional representation. In other words, the loss of information can overcome a threshold that guarantees the possibility of rebuilding the samples effectively. Autoencoders are models that exploit the extreme non-linearity of neural networks, in order to find low-dimensional representations of a given dataset. In particular, let's assume that X is a set of samples drawn from a data-generating process, pdata(x). For simplicity, we will consider xi ∈ ℜn, but there are no restrictions...
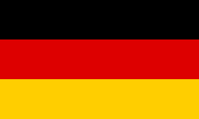
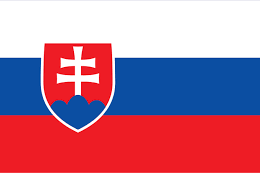
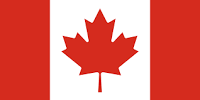
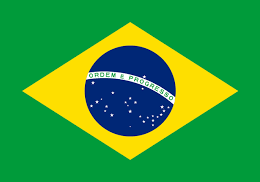

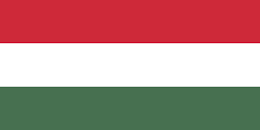
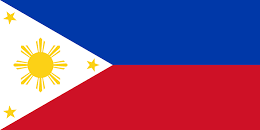
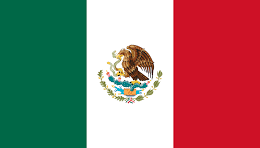
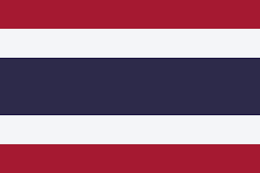
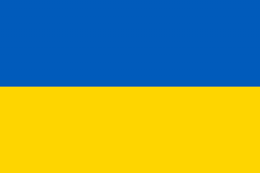
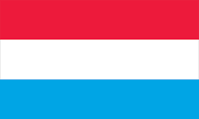
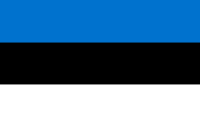
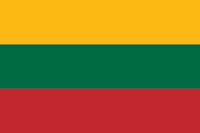
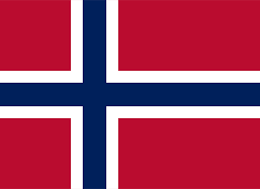
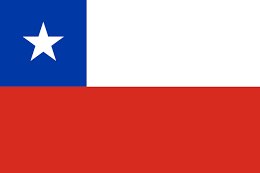
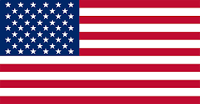
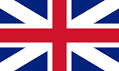
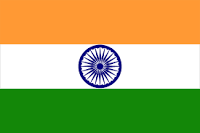
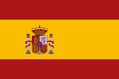
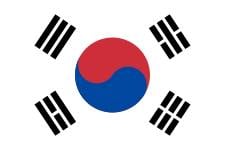
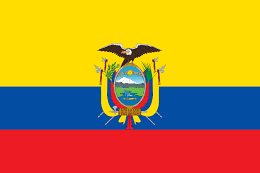
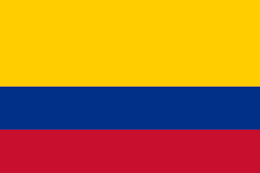
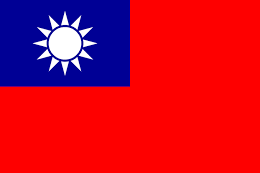
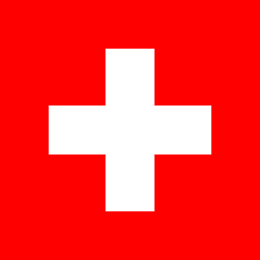
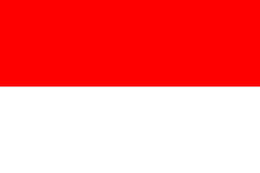
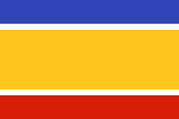
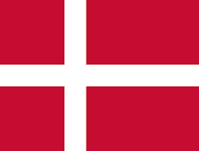
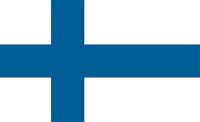
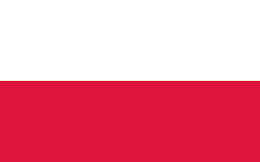
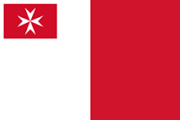
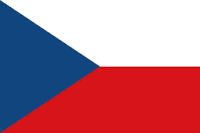
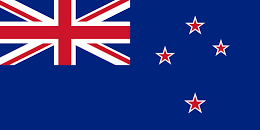
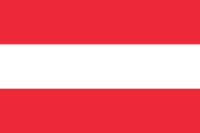
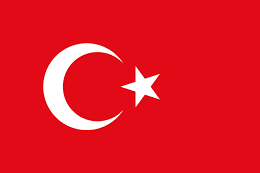
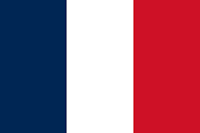
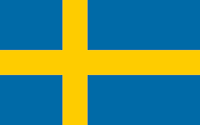
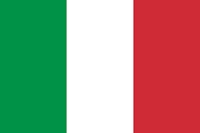
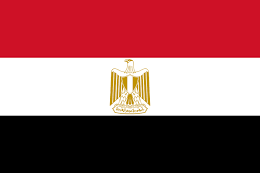
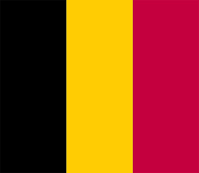
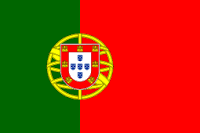
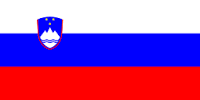
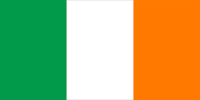
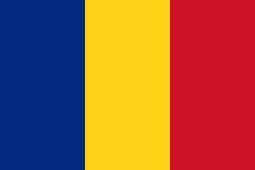
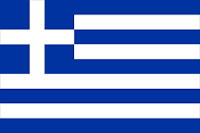
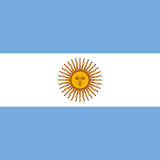
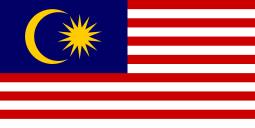
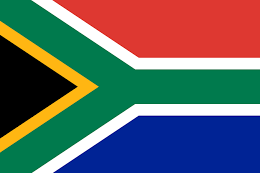
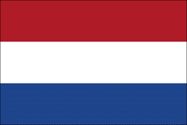
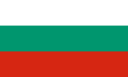
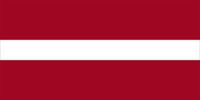
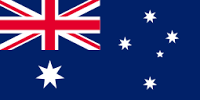
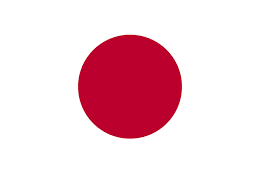
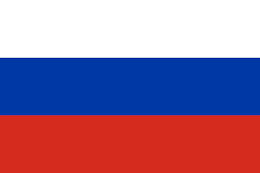