One of the most important requirements when it comes to training machine learning (ML) models and deep neural networks (DNNs) is having large training datasets with distributions (mostly unknown, which we learn about during ML or DNN training) from a given sample space so that ML models and DNNs can learn from this given training data and generalize well to unseen future or separated out test data. Also, a validation dataset, which often comes from the same source as the training set distribution, is critical to fine-tuning model hyperparameters. In many cases, developers start with whatever data is available—either a little or a lot—to train machine learning models, including high capacity deep neural networks. Regardless of the data's size and format, it is important to feed training, validation, and test data to...
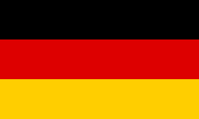
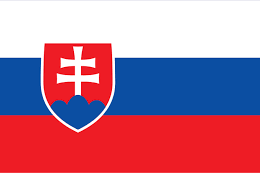
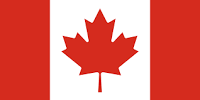
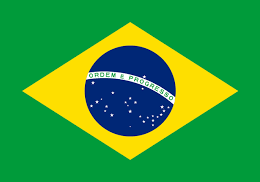

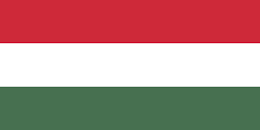
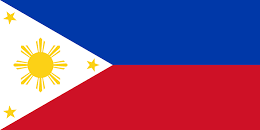
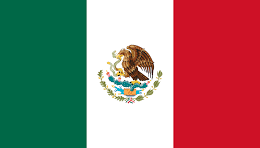
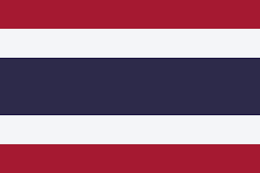
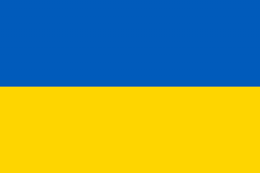
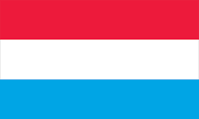
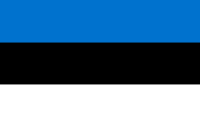
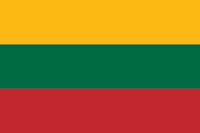
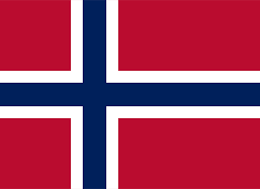
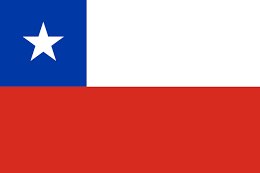
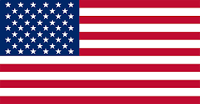
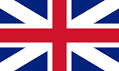
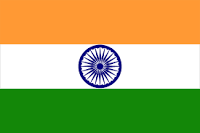
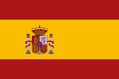
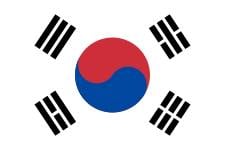
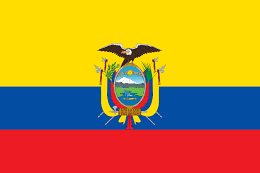
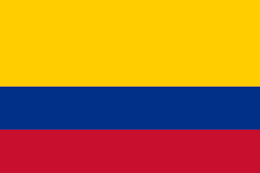
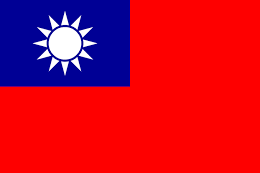
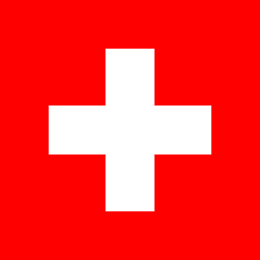
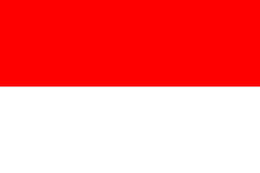
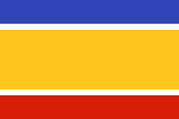
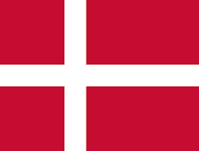
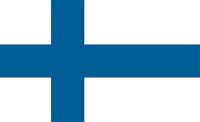
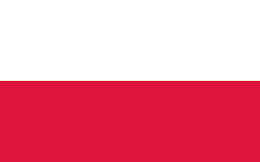
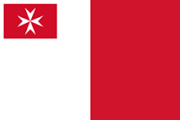
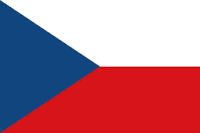
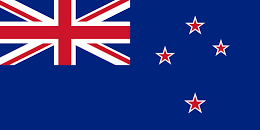
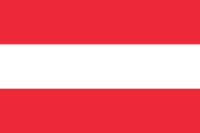
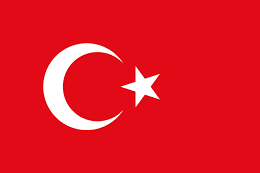
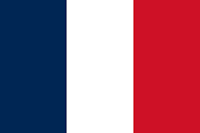
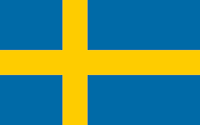
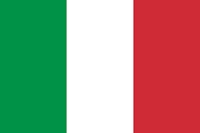
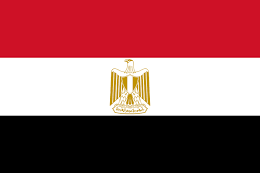
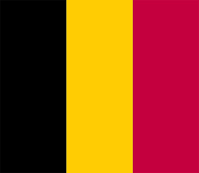
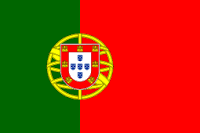
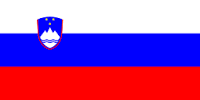
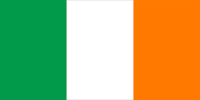
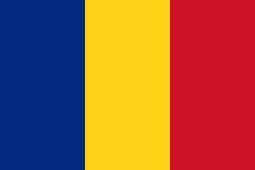
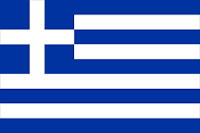
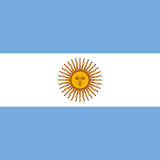
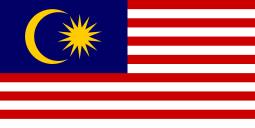
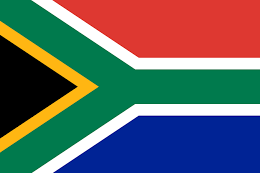
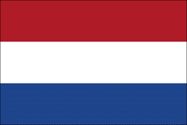
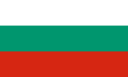
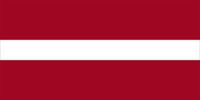
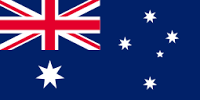
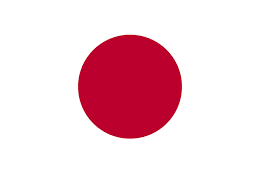
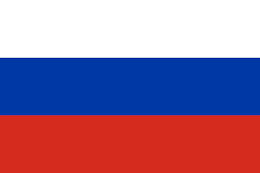