In this chapter, we covered the basic considerations regarding how to choose the kinds of machines for the training clusters. These involve making tradeoffs between costs, memory sizes, compute power, and provisioning limitations. As for deep learning, we provided a concrete example on how to run distributed TensorFlow on SageMaker notebooks, and some guidelines on how to further distribute your deep learning pipelines through Apache Spark on EMR. In the next chapter, Optimizing Models in Spark and SageMaker, we will dive into the problem of tuning our models for optimal performance from the standpoint of model accuracy.
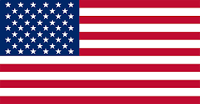
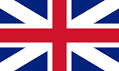
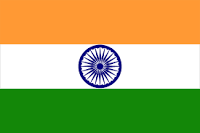
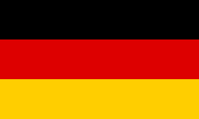
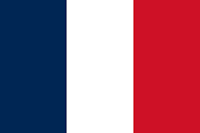
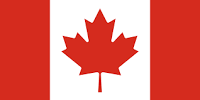
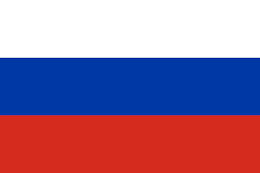
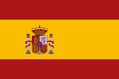
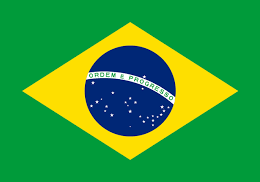
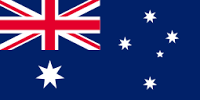

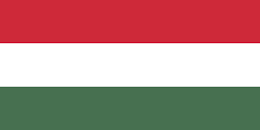
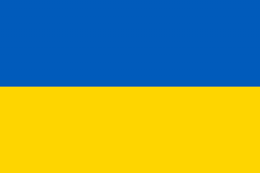
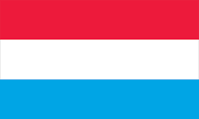
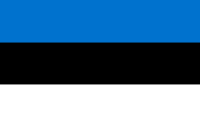
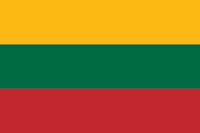
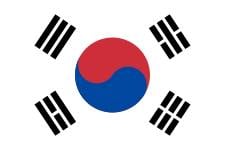
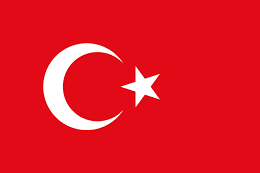
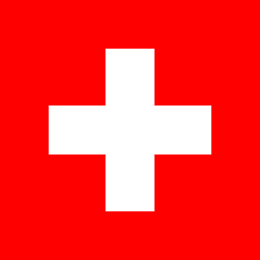
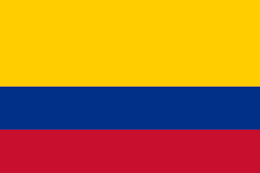
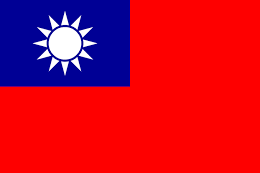
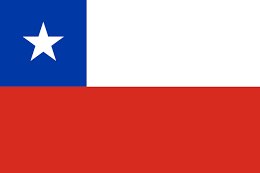
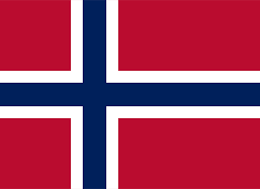
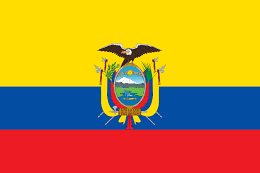
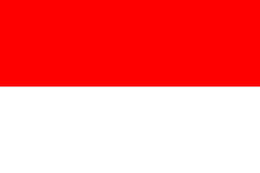
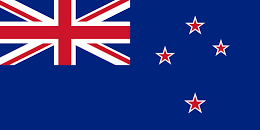
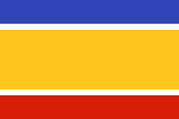
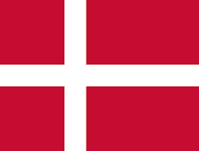
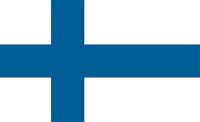
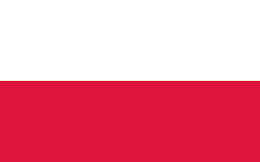
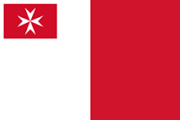
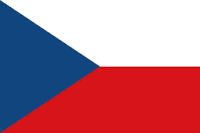
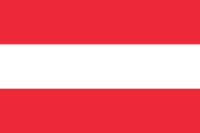
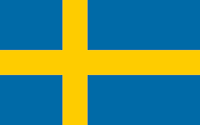
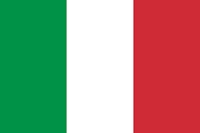
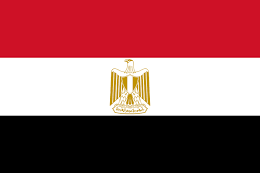
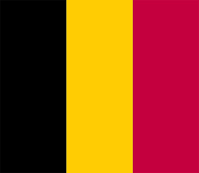
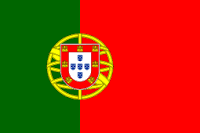
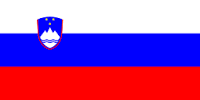
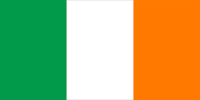
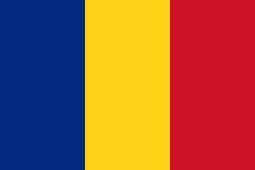
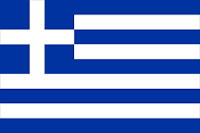
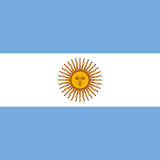
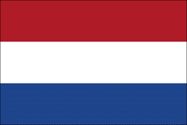
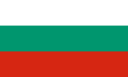
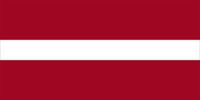
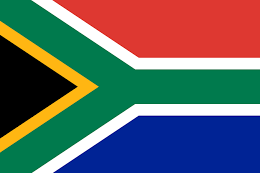
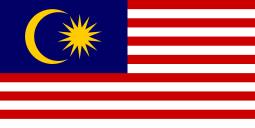
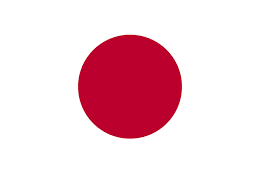
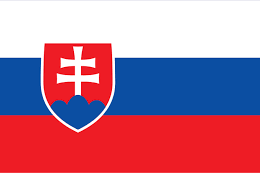
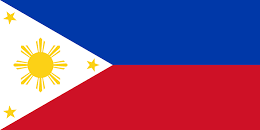
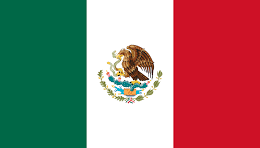
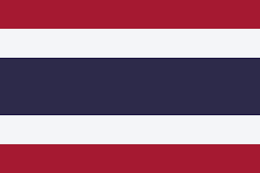