Gradient agreement as an optimization
The gradient agreement algorithm is an interesting and recently introduced algorithm that acts as an enhancement to meta learning algorithms. In MAML and Reptile, we try to find a better model parameter that's generalizable across several related tasks so that we can learn quickly with fewer data points. If we recollect what we've learned in the previous chapters, we've seen that we randomly initialize the model parameter and then we sample a random batch of tasks,
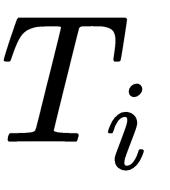
from the task distribution,
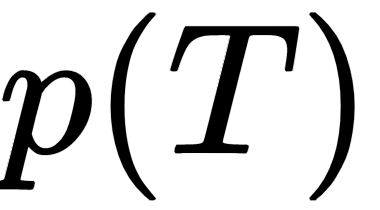
. For each of the sampled tasks,
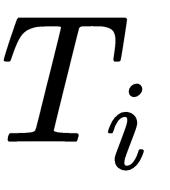
, we minimize the loss by calculating gradients and we get the updated parameters,
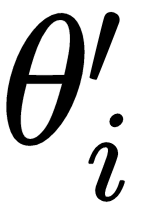
, and that forms our inner loop:

After calculating the optimal parameter for each of the sampled tasks, we perform meta optimization— that is, we perform meta optimization by calculating loss in a new set of tasks, we minimize loss by calculating gradients with respect to the optimal parameters
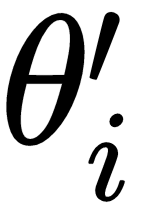
, which we obtained in the inner loop, and we update our...