FL as the main solution for data problems
So far in this chapter, we confirmed that big data has issues to be addressed. Data privacy must be preserved in order to protect not only individuals but also data users who would face risks of data breaches and subsequent fines. Biases in a set of big data can affect inference significantly through proxies, even when factors about gender and race are omitted, and focus on correlation rather than causation makes predictive models vulnerable to model drift.
Here, let us discuss the difference between a traditional big data ML system and an FL system in terms of their architectures, processes, issues, and benefits. The following diagram depicts a visual comparison between a traditional big data ML system and an FL system:
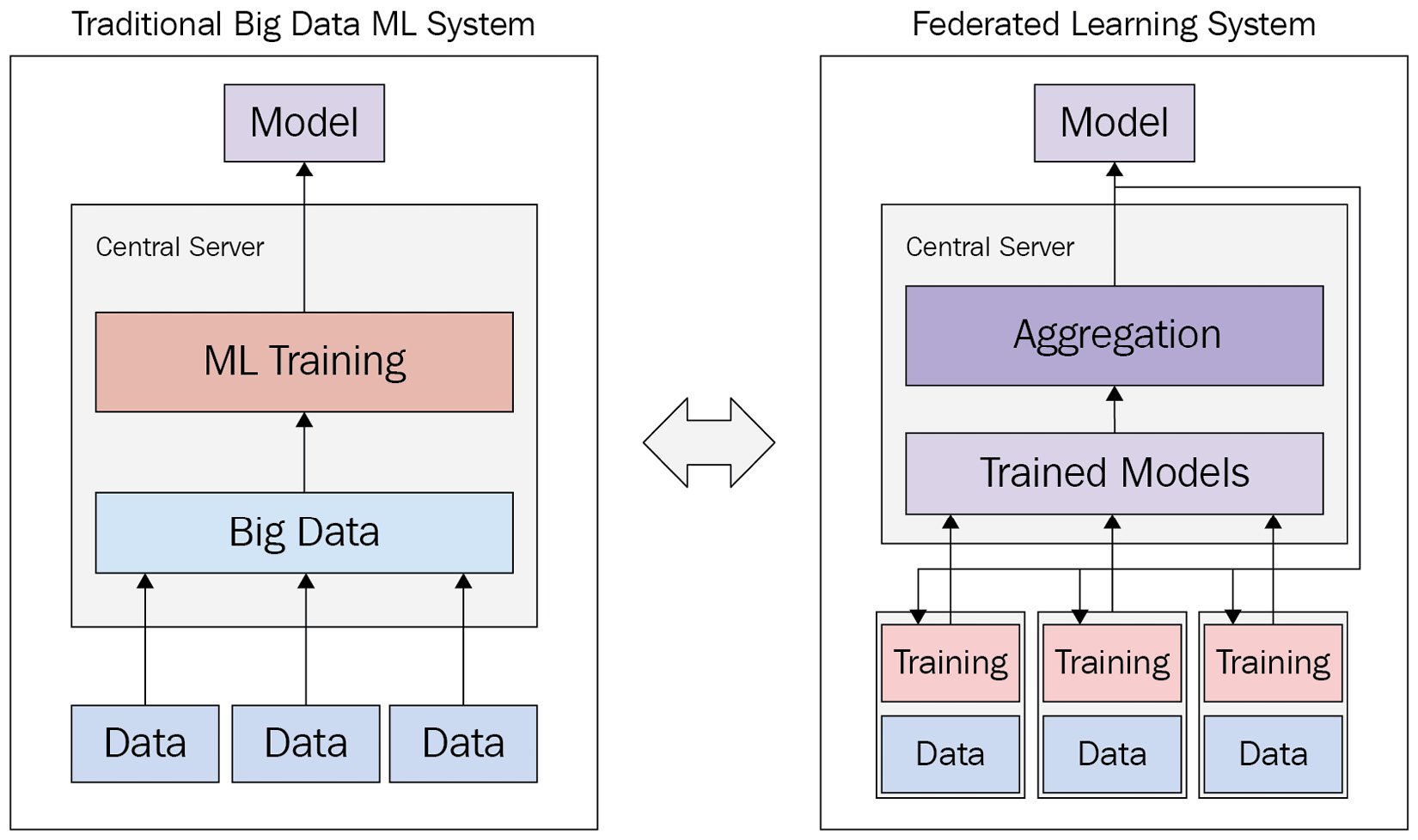
Figure 1.3 – Comparison between traditional big data ML system and FL system
In the traditional big data system, data is gathered to create large data stores. These large data stores are used to solve a specific problem using ML. The resulting model displays strong generalizability due to the volume of data it is trained on and is eventually deployed.
However, continuous data collection uses large amounts of communication bandwidth. In privacy-focused applications, the transmission of data may be banned entirely, making model creation impossible. Training large ML models on big data stores is computationally expensive, and traditional centralized training efficiency is limited by single-machine performance. Slow training processes lead to long delays between incremental model updates, leading to a lack of flexibility in accommodating new data trends.
On the other hand, in an FL system, ML training is performed directly at the location of the data. The resulting trained models are collected at the central server. Aggregation algorithms are used to produce an aggregated model from the collected models. The aggregated model is sent back to the data locations for further training.
FL approaches often incur overhead to set up and maintain training performance with distributed-system settings. However, even with a bit more complicated architecture and settings, there are benefits that excel its complication. Training is performed at the data location, so data is never transmitted, maintaining data privacy. Training can be performed asynchronously across a variable number of nodes, which results in efficient and easily scalable distributed learning. Only model weights are transmitted between server and nodes, thus FL is efficient in communication. Advanced aggregation algorithms can maintain training performance even in restricted scenarios and increase efficiency in standard ML scenarios too.
The vast majority of all AI projects do not seem to be delivered, or simply fall short altogether. To deliver an authentic AI application and product, all the issues discussed previously need to be considered seriously. It is obvious that FL, together with other key technologies to deal with local data processed by the ML pipeline and engine, is getting to be a critical solution to resolve data-related problems in a continuous and collaborative manner.
How can we harness the power of AI and ML to optimize the technical system for society in its entirety—that is, bring about a more joyous, comfortable, convenient, and safe world while being data minimalistic and ethical, as well as delivering improvements continuously? We contend that the key is a collective intelligence or intelligence-centric platform, also discussed in Chapter 10, Future Trends and Developments. In subsequent chapters of the book, we introduce the concept, design, and implementation of an FL system as a promising technology for orchestrating collective intelligence with networks of AI and ML models to fulfill those requirements discussed so far.