What is ML?
ML is a subfield of artificial intelligence (AI) in which computer systems learn patterns from data to perform specific tasks or make predictions on unseen data without being explicitly programmed. In 1959, Arthur Samuel defined ML as a “field of study that gives computers the ability to learn without being explicitly programmed to do so.” To give clarity to the definition given to us by Arthur Samuel, let us unpack it using a well-known use case of ML in the banking industry.
Imagine we work in the ML team of a Fortune 500 bank in the heart of London. We are saddled with the responsibility of automating the fraud detection process as the current manual process is too slow and costs the bank millions of pounds sterling, due to delays in the transaction processing time. Based on the preceding definition, we request historical data of previous transactions, containing both fraudulent and non-fraudulent transactions, after which we go through the ML life cycle (which we will cover shortly) and deploy our solution to prevent fraudulent activities.
In this example, we used historical data that provides us with the features (independent variables) needed to determine the outcome of the model, which is generally referred to as the target (dependent variable). In this scenario, the target is a fraudulent or non-fraudulent transaction, as shown in Figure 1.1.
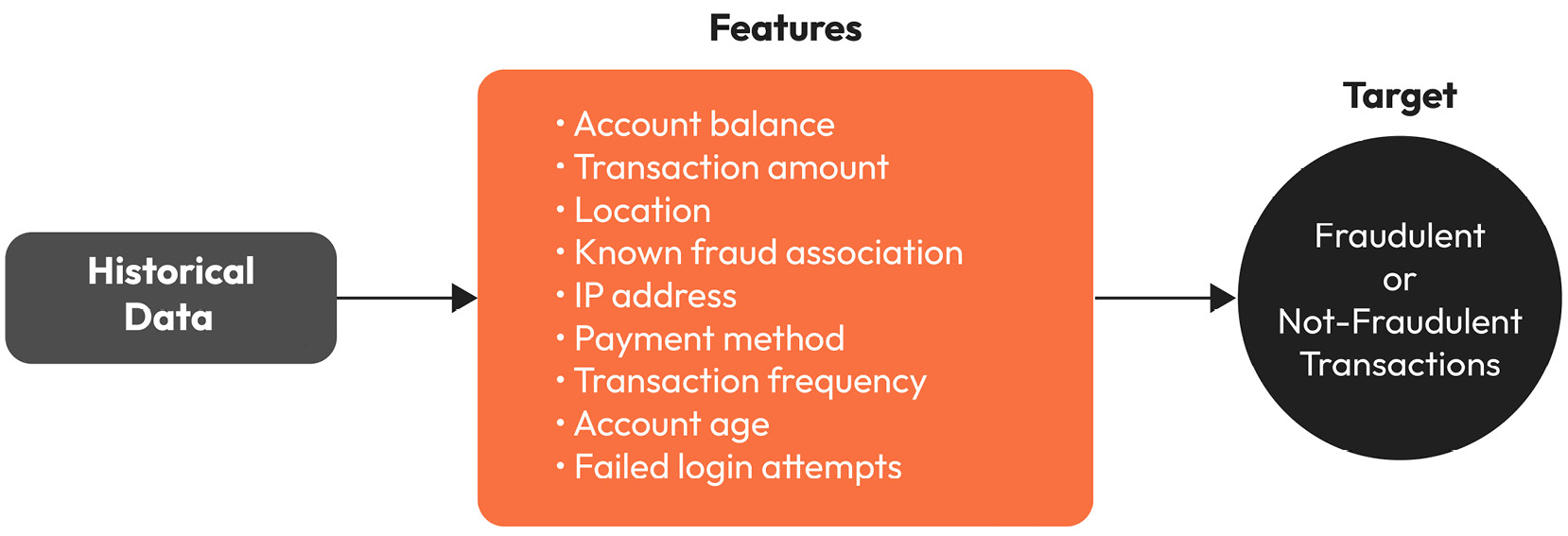
Figure 1.1 – A flowchart showing the features and the target in our data
In the preceding scenario, we were able to train a model with historical data made up of features and the target to generate rules that are used to make predictions on unseen data. This is an example of what ML is all about – the ability to empower computers to make decisions without explicit programming. In classic programming, as shown in Figure 1.2, we feed in the data and some hardcoded rules – for example, the volume of a daily transaction to determine whether it is fraudulent or not. If a customer goes above this daily limit, the customer’s account gets flagged, and a human moderator will intervene to decide whether the transaction was fraudulent or not.
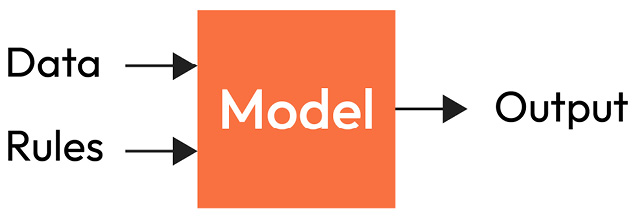
Figure 1.2 – A traditional programming approach
This approach will soon leave the bank overwhelmed with unhappy customers constantly complaining about delayed transactions, while fraudsters and money launderers will evade the system by simply limiting their transactions within the daily permissible limits defined by the bank. With every new attribute, we would need to update the rules. This approach quickly becomes impractical, as there will always be something new to update to make the system tick. Like a house of cards, the system will eventually fall apart, as such a complex problem with continuously varying attributes across millions of daily transactions may be near impossible to explicitly program.
Thankfully, we do not need to hardcode anything. We can use ML to build a model that can learn to identify patterns of fraudulent transactions from historical data, based on a set of input features in it. We train our model using labeled historical data of past transactions that contain both fraudulent and non-fraudulent transactions. This allows our model to develop rules based on the data, as shown in Figure 1.3, which can be applied in the future to detect fraudulent transactions.
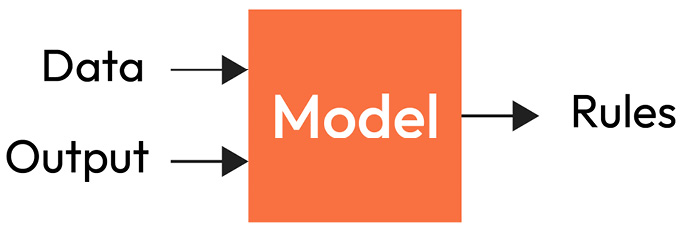
Figure 1.3 – An ML approach
The rules generated by examining the data are used by the model to make new predictions to curb fraudulent transactions. This paradigm shift differs from traditional programming where applications are built using well-defined rules. In ML-powered applications, such as our fraud detection system, the model learns to recognize patterns and create rules from the training data; it then uses these rules to make predictions on new data to efficiently flag fraudulent transactions, as shown in Figure 1.4:
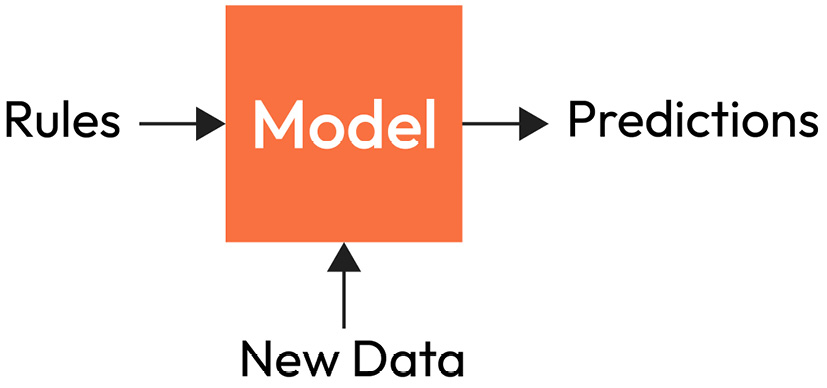
Figure 1.4 – An ML model uses rules to make predictions on unseen data
In the example we just examined, we can see from Figure 1.1 that our training data is usually structured in a tabular form, made up of numerical values such as transaction amount and frequency of transactions, as well as categorical variables such as location and payment type. In this type of data representation, we can easily identify both the features and the target. However, what about textual data from social media, images from our smartphones, video from streaming movies, and so on, as illustrated in Figure 1.5? How do we approach these types of problems where the data is unstructured? Thankfully, we have a solution, which is called deep learning.
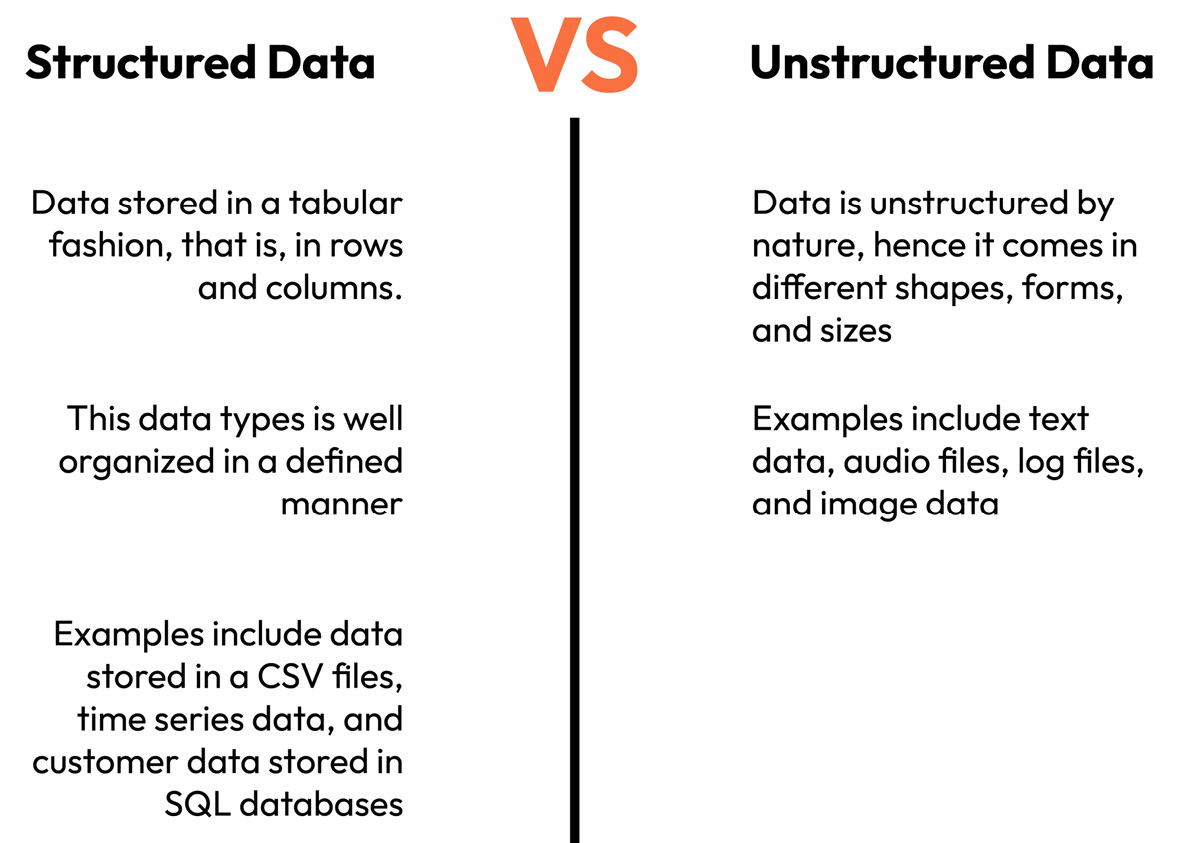
Figure 1.5 – An illustration of structured and unstructured data types
Deep learning is a subset of ML that mimics the human brain by using complex hierarchical models that are composed of multiple processing layers. The buzz around deep learning lies around the state-of-the-art performance recorded by deep learning algorithms over the years across many real-world applications, such as object detection, image classification, and speech recognition, as deep learning algorithms can model complex relationships in data. In Sections 2 and 3, we will discuss deep learning in greater detail and see it in action for image and text applications, respectively. For now, let us probe further into the world of ML by looking at the types of ML algorithms.