It is easy to discover patterns by visualizing data with two or three dimensions. A high-dimensional dataset cannot be represented graphically, but we can still gain some insights into its structure by reducing it to two or three principal components. Collected in 1936, Fisher's Iris dataset is a collection of fifty samples from each of three species of Iris: Iris setosa, Iris virginica, and Iris versicolor. The explanatory variables are measurements of the length and width of the petals and sepals of the flowers. The Iris dataset is commonly used to test classification models, and is included with scikit-learn. Let's reduce the iris dataset's four dimensions so that we can visualize it in two dimensions. First we load the built-in iris dataset and instantiate a PCA estimator. The PCA class takes the number of principal...
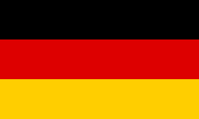
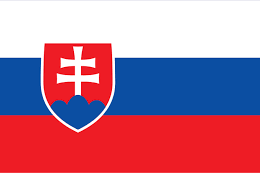
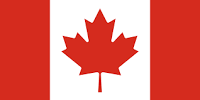
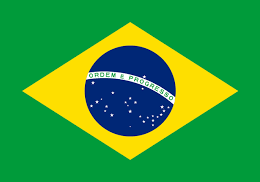

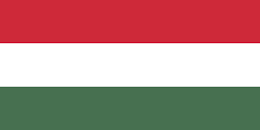
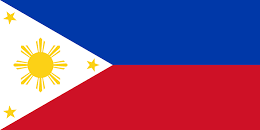
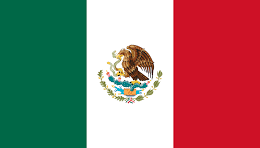
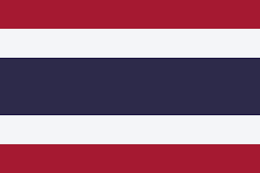
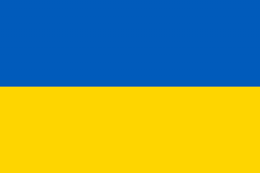
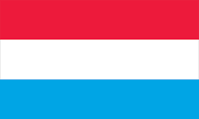
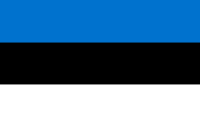
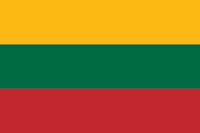
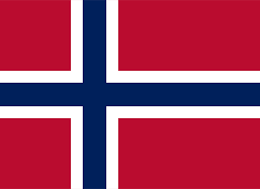
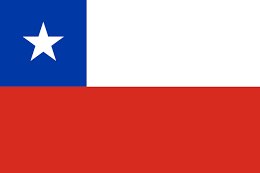
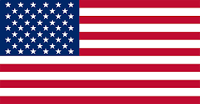
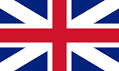
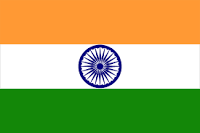
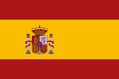
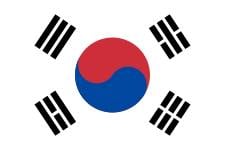
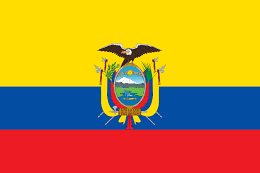
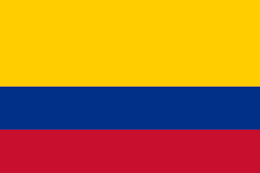
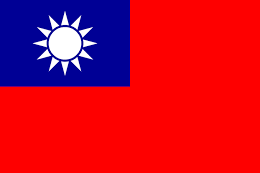
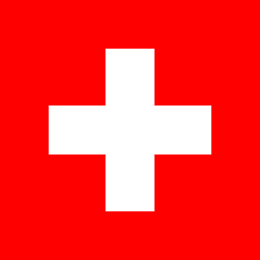
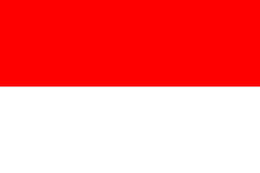
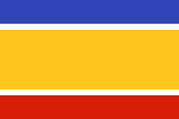
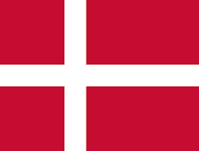
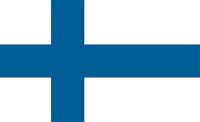
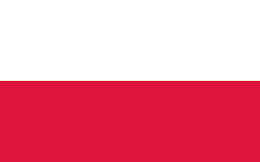
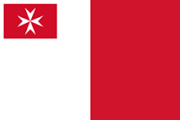
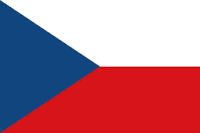
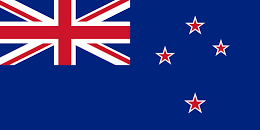
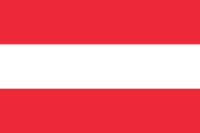
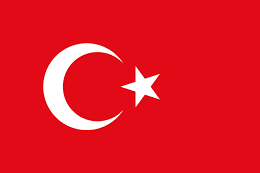
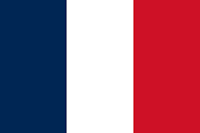
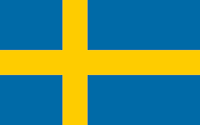
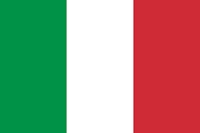
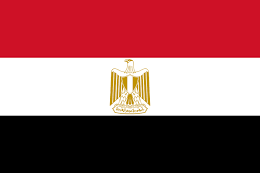
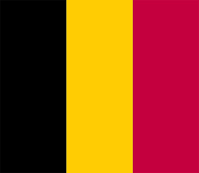
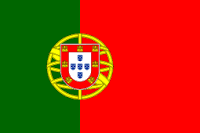
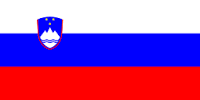
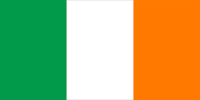
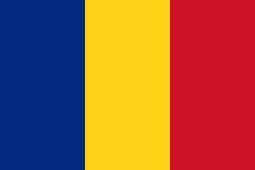
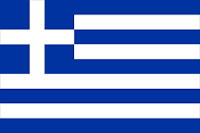
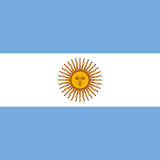
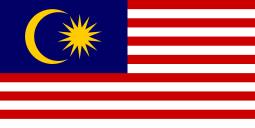
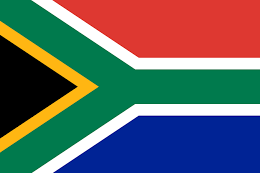
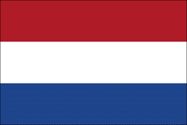
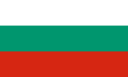
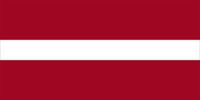
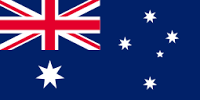
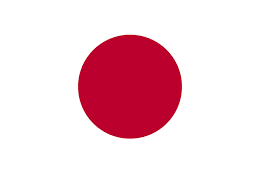
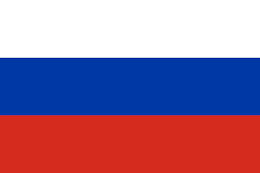