All beginnings are hard, and this is equally true when a company wants to start with data-informed decision making (DIDM). There are sometimes reports that give a general idea of how well the organization is doing financially. People often think, We have some Excel reports, that’s enough. But when reality hits, they realize they need more information (for example, not only information about financial matters).
When new data projects start or new plans are made to add more information to existing reports, the projects take too long, the people who wrote the hard macros are no longer available, or the project methods are no longer good. With a big bang scenario, projects will take too long and more parts will be added as the project goes on. This is called scope creep.
Another common way organizations limit the effectiveness of their data is when business users in organizations just use the numbers on the Key Performance Indicators (KPI) reports as they are. In Chapter 6, Aligning with Organizational Goals, we help you understand the KPIs and how they should align with the strategic objectives. These business users do things such as hear, see, and be quiet. They seem to think, Oh no, that’s too bad. Let’s hope the numbers are better tomorrow, next week, or next month. To be honest, this is still a very serious problem in organizations. Most of the time, it’s because business users can’t make decisions that are based on facts. Many times, it is even worse when we don’t know how to answer business questions even when we are in business and can’t make a decision as simple as how to read graphs and reports or how to analyze. Still, it’s important to keep track of your organization’s goals and help people understand the insights that can be drawn from data. This will help them make decisions based on the data and not get lost in the data-insights jungle.
As mentioned at the beginning of this chapter, we have so much data in this digital age. Our data mountain is growing every day (1.7 MB per second per person on this planet), and data can help us be successful in what we do. So, we need to look at how information moves through an organization and what kind of knowledge and processes need to be taken care of. The following diagram represents a visualization of how the data grows, covering the flow of data and which disciplines are involved.
Figure 1.12 – The flow of data
If we look at how data-informed decision making works, we can see that it all starts with signals. There are a lot of signals around us right now. These signals are turned into data, and then that data becomes information (something we can do something with), and finally, knowledge. We’ll use the following simple example of a traffic light to show how this works:
- Signal: We are receiving light waves in our eyes.
- Data: The data that we receive or see is that the light is red.
- Information: The information that we have is that we know it’s a traffic light.
- Knowledge: The knowledge that we have is that we know we must stop.
- Action: The action is that we will stop in front of the traffic light as we don’t want to have an accident or get fined for passing the red traffic light.
One thing that can hinder the ideal flow of data in an organization is where data is stored and how it is managed. Data is kept in many places, for example, POS systems, CRM systems, and Excel documents, which keep track of sales data, marketing data, and financial data respectively. Multiple source systems are usually not a problem in smaller organizations, but in larger ones, they could be a problem and a potential risk.
When organizations don’t pay attention to data management for simple things, such as different types of addresses or different definitions of fields, this could cause problems in how data flows through the organization. Data governance needs a place as well within your processes when we think of the usage of data. Do not confuse data governance with data management. Data management is about the day-to-day business when we use data in a company, whereas data governance looks at how the company can make more money using data in the future. The earlier Figure 1.12 shows the different disciplines that an organization could use to set up a data and analytics environment, not just from a technical point of view, but also from a business point of view. To ensure data is managed effectively, businesses need the right skills and processes in place:
- Data management: Businesses deal with a lot of data. That data needs to be managed well so everyone can use it effectively. It is necessary to take care of things such as data entry, data registers, data quality, and so on. Today, we call this the data office, and a chief data officer usually runs it.
- Privacy management: Keeping personal, customer, or proprietary information safe and secure is vital. Privacy laws exist in many countries, covering things such as the right way to handle data. Most of the time, a data protection officer takes care of this. Later in this book, there will be more about privacy and how to handle data in an ethical and secure way.
- Information management: Information management is an important part of turning data into the insights that are needed. There are many ways to describe information management, but we think this is the best one: information management is the process that ensures that the information needs arising from various processes of an organization are translated into insights necessary for measuring and achieving the organizational objectives.
- Knowledge management: Businesses need to manage their information and knowledge effectively to avoid wasting people’s time searching for the right information. Too much knowledge is stored in our (collective) brains, so we need to register and collect knowledge and plan for the future.
On a small side note, some organizations in the Netherlands have a staff with an average age of 48. That means that information about processes, systems, designs, and other things needs to be kept for the future.
- Change management: Change management is a systemic approach to dealing with changes in a business. Every new dashboard, report, or change to an existing dashboard or report is overall a change, and we need to help the management and business stakeholders accept the change and work with the new dashboards and reports. There is more in this book about this subject.
- Strategic management: Strategic management is how an organization manages and achieves its overall goals. Data and analytics need to be embraced by the management or board of directors, and they need to set an example for the whole staff. If the people in charge don’t take part in data and analytics projects, the idea will fail.
As you read through this book, there may be many concepts that are new to you. We’ll help you gain a better understanding through case studies, such as the next one.
Intermezzo 1 – Is data management necessary?
This first story is from an organization that has a lot of complex systems. This organization wanted to set up a new source system for their registrations. Moreover, it was necessary to build connections between other systems. Of course, one of them was the connection to the data warehouse system.
Because no data management or data governance was in place, or no register for data elements was available in conversations that were held about common things such as how we arrange the connections, which protocols we need, and so on.
It would have been much better if we would have talked about the definition of attributes, formats of date fields, or other elementary data fields.
In fact (and this is something we say a lot during lectures and presentations), we all should speak the same data language! When one source system is in a different language than another system, we will run into problems when we want to extract data (or even connect data) or use data for dashboards and reports. You will get into problems if data management or data governance is not in place.
P.S. All the stories that are written in this book are real-life stories, and out of respect for the organizations, we will sometimes not mention any names.
The DIDM journey
Anyway, if we have data and are willing to use any tool, and we want to work with it, the data becomes information. Information management is an important part of the process because of this. In the end, information will become knowledge if we are willing to take in the information and go on that journey to understand the insights. The ideal flow of data or data journey will eventually look like this:
Figure 1.13 – The data-informed decision-making journey
The hardest part is to act on and make decisions based on what you’ve learned. This happens a lot in the places where we work or have worked. As we’ve already said, when the insights are actionable but we forget to deal with the change or forget to put them into action in our projects, we tend to take the insights for granted.
But how do you move forward? You can do this by analyzing the insights in small steps and slowly learning that data literacy and taking action go hand in hand.
In this book, we’ll show you how to work with data, how to read and evaluate insights (be critical and ask questions), and how to make decisions based on what you learn. You must go on a journey, which starts with figuring out your (data) strategy, working with that data and gaining insights, and eventually starting to think about hypothetical questions and using more complex ways to show data. Put that dot on the horizon, start small, and grow quickly.
In the next section, we’ll tell the story of The Oakland A’s who did a great job of using data and analytics.
The success story of The Oakland A’s
Let’s go through the success story of The Oakland A’s where we describe their journey when they started working with data-informed decision making. This story is a true, real-life story and they even created a movie about this success story, named Moneyball.
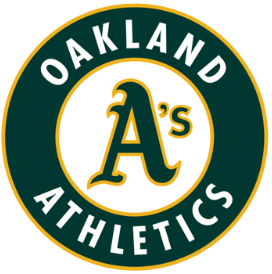
|
Billy Beane, general manager of the Oakland Athletics, is devastated by the team’s loss to the New York Yankees in the 2001 American League Division Series. Some of the star players left the team and Beane needed to assemble a new competitive team for the 2002 competition with a limited budget.
During a scouting visit to the Cleveland Indians, Beane meets Peter Brand, a young Yale economics graduate with some amazing ideas about evaluating players.
Using Brand’s method, Beane signed up undervalued players and traded another player.
|
Figure 1.14 – The Oakland A’s
People are biased, and one of the things said in the movie was about Chad Bradford, who is one of the best pitchers. Bradford was not chosen by any big team, but with the analysis that Brand performed, they found out that he was highly underestimated; why? Because he threw the ball in a funny way (as they say in the movie, because he throws funny). Based on the analysis, they hired Bradford and he became a successful player for the team. This is a big reason why we’re telling this story. He didn’t get picked because of bias. But if you looked at his batting scores, he did well, so he was one of the most undervalued players.
The scouts for the Athletics didn’t like the plan Beane had made, and in the end, Beane fired the head scout. Beane stuck with the new plan, and even though they lost the first 10 games, critics wrote of the new way of working with data.
As Brand says, math and statistics get rid of bias, and to be successful, you need to get things back to one number (one fact, one measure). They traded some players again, and then the team started to win.
The Athletics broke a record by winning 20 games in a row. Hatteberg’s walk-off home run helped them do this. Beane tells Brand that he won’t be happy until they win the World Series with their system and change baseball.
Fun fact
Billy Beane has helped a Dutch soccer team improve its statistical approach and use data to make decisions about players. He even bought some shares in the Dutch soccer team AZ Alkmaar.