What is a visual dictionary?
We will be using the Bag of Words model to build our object recognizer. Each image is represented as a histogram of visual words. These visual words are basically the N centroids built using all the keypoints extracted from training images. The pipeline is as shown in the image that follows:
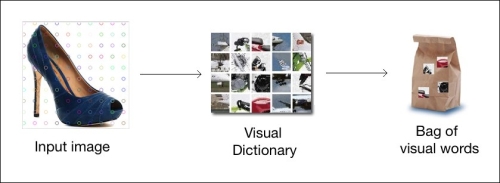
From each training image, we detect a set of keypoints and extract features for each of those keypoints. Every image will give rise to a different number of keypoints. In order to train a classifier, each image must be represented using a fixed length feature vector. This feature vector is nothing but a histogram, where each bin corresponds to a visual word.
When we extract all the features from all the keypoints in the training images, we perform K-Means clustering and extract N centroids. This N is the length of the feature vector of a given image. Each image will now be represented as a histogram, where each bin corresponds to one of the 'N' centroids. For simplicity, let...